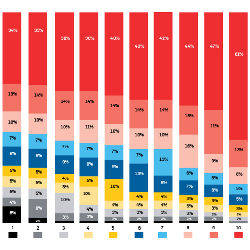
Online commerce has affected traditional retailers, moving transactions such as book sales and movie rentals from shopping malls to cyberspace. But has it fundamentally changed consumer behavior?
Wired Editor-in-Chief Chris Anderson thinks so. In his 2006 book titled The Long Tail and more recently on his Long Tail blog, Anderson argues that online retailers carry a much wider variety of books, movies, and music than traditional stores, offering customers many more products to choose from. These customers, in turn, pick more of the niche products than the popular hits. While individual niche items may not sell much more, cumulatively they’re a bigger percentage of overall business.
The book’s title comes from the shape of a probability distribution graph. Many phenomena follow the normal or Gaussian distribution; most of the values cluster tightly around the median, while in the tails they drop off exponentially to very small numbers. Events far from the median are exceedingly rare, but other phenomena, including book and movie purchases, follow a different course. Values in this distribution drop much less rapidly, and events of roughly equal probability stretch far out into the tail.
Plotted on a graph, such distributions produce a peak very near the y-axis, a cluster near the intersection of the axes, and a long tail along the x-axis. This gives rise to the name long tail, or heavy tail. While a long tail is technically just one class of a heavy tail distribution—other terms include fat tail, power law, and Pareto distribution—in popular usage and for practical purposes there’s no notable difference.
Because many phenomena have heavy tails—computer job sizes, for instance, and Web page links—researchers want to know how the distributions work and their effects.
Recently, for instance, business experts have tested the Long Tail theory and found that its effect on Internet commerce may not be as straightforward as some thought. Serguei Netessine, associate professor of operations and information management at the University of Pennsylvania, looked at data from Netflix, the movie rental service, containing 100 million online ratings of 17,770 movies, from 2000 to 2005, by 480,000 users. Netessine used the ratings as a proxy for rentals in his study, but says he has since looked at actual rental data.
Netessine’s conclusion is, when looked at in percentage terms, the demand for hits actually grew, while the demand for niche products dropped. “There is no increased demand for bottom products that we are seeing in this data,” Netessine says.
“There might be an increasingly long tail, but that tail is getting thinner,” says Anita Elberse.
Anita Elberse, associate professor of business administration at Harvard Business School, earlier looked at data from Quickflix, an Australian movie rental service similar to Netflix, and from Nielsen VideoScan and Nielsen SoundScan, which monitor video and music sales, respectively, and reached the same conclusion. “There might be an increasingly long tail, but that tail is getting thinner,” Elberse says.
Anderson disputes their conclusions, saying the researchers define the Long Tail differently. Anderson defines hits in absolute terms, the top 10 or top 100 titles, while the academics look at the top 1% or 10% of products. “I never do percentage analysis, since I think it’s meaningless,” Anderson wrote in an email interview. “You can’t say ‘I choose to define Long Tail as X and X is wrong, therefore Chris Anderson is wrong.’ If you’re going to critique the theory, you’ve got to actually get the theory right.”
Anderson points to the same Netflix data used by Netessine and notes that the top 500 titles dropped from more than 70% of demand in 2000 to under 50% in 2005. In 2005, Anderson notes, 15% of demand came from below the top 3,000, about the point where brick-and-mortar stores run out of inventory.
“In that sense, Anderson was right, but to me that was more or less a trivial finding, because each year we have more and more movies available,” Netessine says.
Influencing Consumers’ Choices
An improved understanding of where in the distribution consumers land could lead to new methods of swaying their choices, in the form of better-designed recommendation engines. Researchers postulate that one reason consumers fail to choose niche products is that they have no way to find them. “If nobody is buying these items, how do they get recommended in the first place?” asks Kartik Hosanagar, associate professor of operations and information management at the University of Pennsylvania.
Hosanagar says collaborative filtering, based on user recommendations, can’t find undiscovered items. It can, however, find items that are somewhat popular, and bring them to the attention of customers who might have missed them. He found that consumers appreciated recommendation engines that suggest more niche items, perhaps because they were already aware of the blockbusters.
He says retailers might boost their sales with improved recommendation engines. Using content analysis, which identifies characteristics of a product—say, the director or genre of a movie—and suggesting it to buyers of products with similar characteristics, could increase the diversity of recommendations. Hosanagar says researchers looking at Internet commerce shouldn’t assume sales mechanisms and buyers’ behavior are unchangeable. “A lot of social scientists are looking at the system as a given and trying to look at its impact, but what we are saying is the system is not a given and there are a lot of design factors involved,” he says.
That matches the thinking of Michael Mitzenmacher, a professor of computer science at Harvard, who says researchers need a better understanding of how heavy tails come to be, so that they can turn that knowledge to practical use. “For a long time people didn’t realize power law distributions come up in a variety of ways, so there are a variety of explanations,” Mitzenmacher says. “If we understand the model of how that power law comes to be, maybe we can figure out how to push people’s behavior, or computers’ behavior, in such a way as to improve the system.”
For instance, file size follows a power law distribution. An improved understanding of that could lead to more efficiently designed and economical file storage systems. Or if hyperlinks are similar to movie rentals—that is, if the most popular Web pages retain their popularity while the pages out in the tail remain obscure—it might make sense to take that into account when designing search engines. And if search engines have already changed that dynamic, it could be valuable to understand how.
One area where heavy tails affect computer systems is the demand that UNIX jobs place on central processing units (CPUs). Mor Harchol-Balter, associate department head of graduate education in the computer science department at Carnegie Mellon University, says the biggest 1% of jobs make up half the total load on CPUs. While most UNIX jobs may require a second or less of processing time, some will need several hours.
If there were low variability among job sizes, as people used to believe, it would make sense to have just a few, very fast servers. But because the job size distribution is heavy tailed, it’s more efficient to use more, slower machines. Designing server farms with this understanding, Harchol-Balter says, could cut electricity demand by 50%.
“In computer science, we really concentrate on understanding the distribution of the sizes of the requirements, and making our rules based on this understanding,” she notes. “We are having to invent whole new mathematical fields to deal with these kinds of distributions.”
Harchol-Balter finds the origin of heavy tail distributions a fascinating question, one she sometimes asks her classes to speculate about. “Why are files at Web sites distributed according to a Pareto distribution? Why on Earth? Nobody set out to make this kind of distribution,” she says. “Somehow most people write short programs and some people write long programs and it fits this distribution very well.”
But the “why” isn’t her main concern. “I don’t need to know why it happens,” Harchol-Balter says. “I just need to know if it’s there what I’m going to do with it.”
Tan, T.F. and Netessine, S.
Is Tom Cruise threatened? Using Netflix Prize data to examine the long tail of electronic commerce. Working paper, University of Pennsylvania, Wharton Business School, July 2009.
Elberse, A.
Should you invest in the long tail? Harvard Business Review, July-August 2008.
Mitzenmacher, M.
Editorial: the future of power law research. Internet Mathematics 2, 4, 2005.
Harchol-Balter, M.
The effect of heavy-tailed job size distributions on computer system design. Proceedings of ASA-IMS Conference on Applications of Heavy Tailed Distributions in Economics, Engineering and Statistics, Washington, DC, June 1999.
Fleder, D.M. and Hosanagar, K.
Blockbuster culture’s next rise or fall: the impact of recommender systems on sales diversity. Management Science 55, 5, May 2009.
Newman, M.E.J.
Power laws, Pareto distributions, and Zipf’s law. Contemporary Physics 46, 323351, 2005.
Figures
Figure. Each vertical bar represents a decile of DVD popularity, with the DVDs in decile 10 being the most popular. Each bar is subdivided to demonstrate how, on average, customers who rented at least one DVD from within that decile distributed their rentals among all the deciles. Shoppers in the bottom decile, for instance, selected only 8% of their rentals from among its titles—and 34% from among top-decile titles.
Join the Discussion (0)
Become a Member or Sign In to Post a Comment