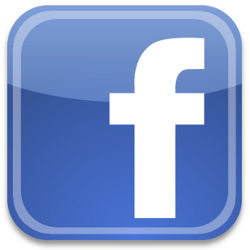
People who avoid social networking sites to maintain their privacy may not be as secure as they think, German computer scientists say.
By applying standard machine learning techniques to sets of Facebook members’ profiles, researchers were able to predict whether two non-members knew each other, with high accuracy. "We were very much astonished that this simple machine learning with simple features already gave 40 percent accuracy," says Katharina Zweig, formerly of the Interdisciplinary Center for Scientific Computing at the University of Heidelberg and one of the authors of the paper, "One Plus One Makes Three (for Social Networks)," which appeared in the April issue of Proceedings of the Library of Science One. With additional work, she says, "we’re certain you could do much better."
The team looked at sets of people who were Facebook members at five universities in 2005. They trained their algorithm on subsets of members from four of those schools, looking for patterns that distinguished members who were linked to each other from those who were not. The most important factors, as it turned out, were the number of friends each person had, the density of their connections within the network, and how many of those friends who were also linked to each other. The researchers then tested their model on an anonymized version of the data set from the fifth school. The 40 percent accuracy is high, Zweig says, because the actual chance of any two people in the data set being linked was around 2 percent.
Social networks often ask new members for access to their email address book, to find other people they know on the network. That means a network such as Facebook or LinkedIn knows about non-members that are linked to members. Using a procedure similar to Heidelberg’s, the network could in theory infer connections between non-members, who have not signed any agreement to cede some of their privacy in exchange for the benefits of membership. Zweig, who has since moved to the Technical University of Kaiserslautern, stresses that she does not know that any social network does this, and she is not sure what the negative consequences would be if they did."We just wanted to show that it is easily possible," she says.
In fact, she adds, because social networks typically have access to more information, such as age, sex, and location, they can probably predict connections with even greater accuracy. They could also study communication patterns between members to learn about the closeness of a connection, and could watch the networks evolve over time.
Other researchers have shown they can use social networks to infer potentially sensitive information, such as sexual orientation or political affiliation. Murat Kantarcioglu, director of the Data Security and Privacy Lab at the University of Texas, Dallas, worries about what information can be gleaned if social network profiles are linked with other information, such as credit reports, and how that might affect things such as insurance rates or access to credit. "I think we need a data mining bill of rights" that would allow people to redact information and fix errors, he says.
Kantarcioglu is working to identify the factors that can reveal private information. With that knowledge, it might be possible to "sanitize" profiles to maintain privacy. And while he doesn’t think that social networks are using data mining techniques now, he believes they will in the future. "This is just the beginning," he says.
Neil Savage is a science and technology writer based in Lowell, MA.
Join the Discussion (0)
Become a Member or Sign In to Post a Comment