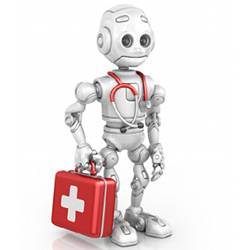
Late last year, the JASON group, an organization of elite science and technology advisers to the U.S. government, released a report suggesting the days of overblown hype for artificial intelligence (AI) and machine learning in medicine were being supplanted by truly useful technologies.
"Unlike previous eras of excitement over AI, the potential of AI applications in health may make this era different," said the report's executive summary, citing frustration with the legacy medical system as the first of three factors contributing to make AI "real" now. (The other two factors are the ubiquity of networked smart devices in our society, and acclimation to convenience and at-home services like those provided through Amazon and others.)
The JASON report footnoted an unheralded study by a group of researchers at the University of Nottingham in the U.K. that might serve to underline how that frustration manifests itself, among both clinicians and laypeople.
The Nottingham researchers developed four machine learning algorithms that analyzed the electronic health records (EHRs) of 378,000 people in the government's Clinical Practice Research Datalink for the onset of cardiovascular disease (CVD). It turned out the Nottingham algorithms were more accurate at predicting both who developed CVD and who registered "false positives than the current benchmark," the widely used ASCVD (Atheroslcerotic Cariovascular Disease) risk calculator of the American College of Cardiology (ACC) and American Heart Association (AHA). Moreover, instead of supporting the ACC/AHA's top risk factors for CVD of cholesterol and blood pressure readings, the Nottingham algorithms suggested age, gender, atrial fibrillation, and social status as defined by the census-based Townsend Deprivation Index were leading factors in the onset of cardiovascular disease.
However, the Nottingham researchers cited one factor that may hinder the willingness of front-line physicians in accepting such contrarian evidence: "It is acknowledged that the 'black-box' nature of machine-learning algorithms, in particular neural networks, can be difficult to interpret," they wrote. "This refers to the inherent complexity in how the risk factor variables are interacting and their independent effects on the outcome."
The JASON report acknowledges and addresses the issues around assessment and acceptance of these new algorithms, and the JASONs are not alone. Harvard University Law School and the University of Copenhagen are spearheading the Project on Precision Medicine, Artificial Intelligence, and the Law (PMAIL); industry analysts are starting to cover the regulatory climate around black-box machine learning; and leading medical informaticists are baking intellectual property and peer review considerations into precision medicine initiatives, which attempt to customize healthcare to the individual, such as the National Institutes of Health's All Of Us project.
Let there be light on either end
W. Nicholson Price II, assistant professor of law at the University of Michigan, has been in the forefront of scholarship on the legal and ethical issues around black box algorithms in medicine. Price, who is a working member of the Harvard-Copenhagen project, published a paper on Regulating Black-Box Medicine last December that recommended the U.S. Food and Drug Administration consider adopting a collaborative governance approach in this area. Such an approach would involve insurers, hospital systems, and providers in the evaluation of health-related algorithms as they are implemented and used in clinical practice.
Price said there is an emerging tension between the public good, which demands as much transparency as possible, and the intellectual property interests of black box developers. "I honestly don't know which way it is going to end up going," Price said. "There's a strong and easy path that says, 'We keep this stuff as secret sauce to the extent we possibly can,' and I think that has some pretty serious negative impacts, but it's not clear to me how exactly the incentive structure will work out if you don't do that."
Brian Edwards, an associate analyst at Boston, MA-based healthcare technology consultancy Chilmark Research, said the traditional method by which medical evidence is evaluated, through peer review of clinical trials, will adapt and demand insight into the training data sets that go into black box algorithms. As this ecosystem expands with more EHR data and outcomes data available, he said, the existing disconnect between clinicians who may be skittish of AI and developers promoting new algorithms may be mitigated.
"The best way we can address this issue is by having publicly available transparent training datasets, so the more the community knows about what went into developing and training the model, the easier it is to accept," Edwards said. "Ideally, you'll be using lots of clinicians to curate these datasets. So much of implementing these types of technologies involves multi-discipline collaboration, where you have lots of talented individuals from different fields working together. A lot of developers haven't understood that very well, yet. They try to build things in isolation and don't include subject matter experts who are extremely important to building a training data set."
I. Glenn Cohen, director of the PMAIL project, said he did not want to speculate about what conclusions the project's investigators might make about best practices, adding that in his previous work in this arena, "I have emphasized the importance of having the medical community be the builders of these technologies, the need to be very purposive about how to build them into hospital workflows, the need for third party auditing, and the question of whether medical education will need to change and incorporate more data science to truly achieve a sea change to make medical practice better."
How to align incentives?
Joshua Denny, M.D., a professor of biomedical informatics at Vanderbilt University in Nashville, TN, and a member of the All of Us working group charged by the U.S. National Institutes of Health with developing a framework for creating and managing a large national health-related research cohort, said misaligned incentives need to be addressed before large-scale black-box algorithm evaluation can begin.
"The legal issues are real, because there is a lot of risk to healthcare centers with EHR data being used for research or any sort of sharing component, but there's not much upside, and that doesn't help that process," Denny said. He has high hopes for the data-sharing potential of the All Of Us project, which plans to enroll 1 million or more Americans. The project's data will include answers to health questionnaires, biometric data, and biosamples such as blood and urine of some participants.
Currently, Denny said, "I can't open up my data from Vanderbilt to a peer reviewer at Columbia (University); there are just too many legal hurdles to get through. So we review things based on how they did it and how they checked it against another data set. There are lots of things we can do, but it would be great if we could also go run the code.
"The infrastructure we are building for All Of Us will be used on the cloud. Users would have to be credentialed, but since it's an open data platform, credentialed users could be in the peer review process."
The All Of Us project is seeing resistance from several of the organizations that could be best placed to help it grow, however. Executives of the U.S.-based Geisinger Health Systems and Kaiser Permanente.health systems recently told The New York Times the project was too time-consuming and they could not wait for All Of Us to mature.
Ultimately, however, Denny said amassing an open records and biobank the size of All Of Us is precisely the sort of resource good AI algorithms will need—records of large, diverse populations spread across a wide range of locations.
"You're going to have to test in multiple environments, and probably across multiple datasets feeding it, and you also want to make sure you test in multiple ancestries, and of course men and women," Denny said. "It's almost comical that you have to say that, but it's not always done."
Gregory Goth is an Oakville, CT-based writer who specializes in science and technology.
Join the Discussion (0)
Become a Member or Sign In to Post a Comment