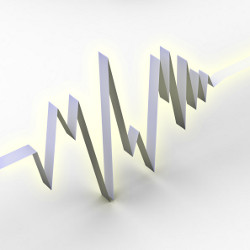
In a critical episode of The Mandalorian, a TV series set in the Star Wars universe, a mysterious Jedi fights his way through a horde of evil robots. As the heroes of the show wait anxiously to learn the identity of their cloaked savior, he lowers his hood, and—spoiler alert— they meet a young Luke Skywalker.
Actually, what we see is an animated, de-aged version of the Jedi. Then Luke speaks, in a voice that sounds very much like the 1980s-era rendition of the character, thanks to the use of an advanced machine learning model developed by the voice technology startup Respeecher. “No one noticed that it was generated by a machine,” says Dmytro Bielievtsov, chief technology officer at Respeecher. “That’s the good part.”
Respeecher is one of several companies developing systems that use neural networks to model the voice of a particular speaker, then apply that model and create speech that sounds like that individual, even if the person has never actually uttered the words being spoken. The potential for deepfake-type uses is unsettling, so Respeecher is careful to secure approval from individuals before applying the technology to their voices. The company, and others like it, also are working on digital watermarking and other techniques to indicate a sample is synthesized.
There are many positive applications for such voice cloning systems. “If you know that you might lose your voice because of surgery or a medical condition, then you could record it in advance, create a model of your voice, and have the synthesized speech sound like you,” observes Simon King, a professor of speech processing at the U.K.’s University of Edinburgh.
Some companies are pushing the technology even further, developing systems that automatically dub dialogue into other languages while retaining the voice characteristics of the original speaker. Although many challenges remain, advances in speech recognition, translation, and synthesis have accelerated progress in the area, suggesting we might be hearing more subtly synthesized voices in the years to come.
From Fiction to Fact
Researchers have been working to develop automatic speech-to-speech translation for at least three decades, according to computer scientist Alan Black of the Language Technologies Institute at Carnegie Mellon University. In the early 2000s, the U.S. Defense Advanced Research Projects Agency (DARPA) funded a project with the goal of developing a universal translator. Black says the teams involved made significant progress translating from English to Arabic and Iraqi dialects, but there were limitations, and it never achieved the sleek functionality of the universal translator popularized in Star Trek.
“It was far away from what you see in Star Trek, but it actually worked with sentence-level translation, in the sense that targeted, non-expert users could get something out of it,” says Black.
The process of automatically generating speech in a language different from the original requires several steps. First, speech recognition transforms the original audio into text (think Siri). Machine translation technology then converts that text into the target language (Google Translate has significantly advanced this domain, but it remains tremendously complex, as characteristics such as word order may vary from language to language). Finally, a text-to-speech (TTS) system generates natural-sounding, personalized audio.
In the past, TTS technologies worked by drawing from a huge audio database consisting of prerecorded phrases broken down into segments. To generate speech from text, systems would draw on the appropriate audio fragments in the database and stitch them together. What resulted was often stereotypically robotic dialogue that lacks the pauses, tonal shifts, prosody, and overall flow characteristic of human speech.
Recent breakthroughs in TTS have led to more natural-sounding results. Generally, TTS is broken down into two steps. Text is transformed into acoustic features, typically in the form of a spectrogram, and then a tool called a vocoder is applied to transform the spectrogram into audio.
Google’s Tacotron 2 model represented a breakthrough in the first step, and its partnership with London-based DeepMind spurred advances in the second step through a tool called WaveNet, which uses neural networks to convert the acoustic features into audio samples. The resulting speech, produced by these models instead of stitched-together fragments, was more realistic and human. Today, says computer scientist Brian Mak of the Hong Kong University of Science and Technology, there are other neural-network-based vocoders that perform as well as WaveNet.
Amazon followed up with advances of its own, using a neural network approach to produce more natural-sounding speech for Alexa and to adjust the program’s voice style based on context. For example, Alexa now sounds different when relaying news or current events than when talking about a song that just played.
The Future of Dubbing
Hong Kong University of Science and Technology’s Mak developed a system that can generate speech in a different language while retaining the characteristics of the original speaker. His team trained their model on audio samples from 2,380 people, each of whom provided just 20 minutes of training speech; the system then modeled each person’s voice by converting it into a high-dimensional vector comprised of 128 different qualities and characteristics. These are not standard qualities such as pitch and tone; instead, the machine learning model identifies the distinguishing features of each voice within the raw audio data. The vectors, Mak explains, are not entirely explainable in human terms. “Right now, it sounds like magic, but if we have to say exactly what the numbers in the vector represent, it’s very hard,” Mak says.
The system does not include translation, but if you want to generate Cantonese speech from an English speaker, Mak explains, then you input the Cantonese text, and the resulting audio sounds like the speaker in a different language. The technology works best if the speaker contributed to the training set, yet it is also effective roughly 50% of the time for random speakers who did not help train the model, according to Mak.
Tel Aviv, Israel-based startup Deep-dub is developing technology that rapidly dubs movies, television series, and other video content into other languages. To create a model of an actor’s voice, the Deepdub system segments a voice sample into pieces, then runs the sample through a neural network that maps the speaking style of the person. This, in turn, generates a model that can be applied to speech translated and then synthesized in other languages. The system maps variables such as pitch, pace, timbre, expressivity, and emotion.
“If you just chain together automatic transcription, translation, and speech synthesis, you end up accumulating too many errors.”
Deepdub chief revenue officer Oz Krakowski echoes Mak’s point that there are qualities the machine learning model identifies that are not recognizable to humans. “There is a limit to how many words we have to describe voice style,” Krakowski says. “The machine has way more, in the realm of hundreds of thousands of different specific items the machine is mapping.”
The company says its technology is capable of generating a complete voice style from just two to five minutes of high-quality audio. This does not lead to instantly perfect translation of the sort depicted in science fiction, however. According to Krakowski, the Deepdub technology eliminates the common shortcomings of machine-generated speech, such as breaks in the voice, metallic-sounding artifacts, and unnatural sounds. Yet the more expressivity in a voice sample—shouting or pleading emotionally, for example—the greater the challenge. The company fine-tunes the output to bring the quality of the results up to Hollywood standards. A reviewer flags any segments that need tweaking, then effectively instructs the model to focus on that particular area and correct the speech fragment.
London, U.K.-based voice-dubbing company Papercup also keeps humans in the loop, explains the University of Edinburgh’s King, who advises the organization. For example, in addition to other applications and use-cases, Papercup creates dubbed versions of time-sensitive news reports from the digital outlet Insider in a matter of hours, translating news segments from English into Spanish, which vastly increases the outlet’s reach. “They will have humans correcting at all stages in that pipeline of speech recognition, translation, and synthesis,” says King. “If you just chain together automatic transcription, translation, and speech synthesis, you end up accumulating too many errors.”
Both Deepdub and Papercup aim to reduce the number of these review iterations and accelerate the process. Deepdub hopes to be able to cut the time required to dub a movie into another language from the 15 to 20 weeks needed using traditional voice actors to a matter of three weeks.
This year, Deepdub will use its technology to dub multiple foreign-language programs from the streaming service Topic into English. Papercup is expanding its customer base as well, and Respeecher plans to build off its Star Wars success by launching its own dubbing solution, along with a voice-over tool that will let actors perform and generate speech in other voices.
“If you add some humans in, and correct it, you can satisfy some segments of the market,” says King, “but it will be quite a long time before you get perfect transcription followed by perfect machine translation and synthesis.”
Breen, A. and Sharma, N.
How We Make Alexa Sound More Human-Like, Amazon re:MARS, https://www.youtube.com/watch?v=FdVYnhzvQtQ
Liu, Z. and Mak, B.
Cross-lingual Multi-speaker Text-to-speech Synthesis for Voice Cloning without Using Parallel Corpus for Unseen Speakers, ICASSP 2020, Nov. 26, 2019, https://arxiv.org/abs/1911.11601
King, S.
Measuring a decade of progress in Text-to-Speech. Loquens, January 2014, https://doi.org/10.3989/loquens.2014.006
Van den Oord, A. and Dieleman, S.
Wavenet: A generative model for raw audio, DeepMind Blog, Sept. 8, 2016, https://bit.ly/3pXZNzm
Wang, Y. et al.
Tacotron: Towards end-to-end speech synthesis, InterSpeech 2017; https://arxiv.org/abs/1703.10135
Join the Discussion (0)
Become a Member or Sign In to Post a Comment