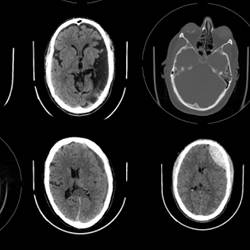
Artificial intelligence (AI) image analysis software has become more adept at diagnosing heart failure and various cancers than top-flight doctors, according to researchers at Case Western Reserve University in Cleveland, OH.
Specifically, the researchers reported their suite of computational imaging and AI tools had reviewed magnetic resonance imaging (MRI), computed tomography (CT), and other medical imaging and predicted, with 97% accuracy, evidence of pending heart failure in 105 patients. In comparison, two pathologists examining the same images for the same group of patients were only able to predict impending heart failures with 74% and 73% accuracy, respectively.
The AI software suite also generated more accurate results when going up against radiologists in a number of tests, including identifying the presence of significant prostrate cancer on test slides in 70% of cases where the radiologists saw no cancer.
The tool suite relies on a combination of image analysis, statistical pattern recognition, machine learning, and other AI tools to analyze images drawn from MRI, CAT scans, and slides.
"There is desperate need for better decision-support tools that allows (doctors) to serve patients, especially in places where there are very few pathologists or radiologists," says Anant Madabhushi, F. Alex Nason Professor II of Biomedical Engineering in Case Western's department of biomedical engineering and director of the university's Center for Computational Imaging and Personalized Diagnostics (CCIPD), who leads the research that underlies the development of the AI software suite.
Madabhushi says his team used machine learning to train the AI software to recognize patterns in benign versus malignant tumors, for example, by exposing it to thousands of images that his team has secured from clinics and hospitals around the world. In addition, the team inserts "hand-crafted" insights into the training process, essentially helping the software to learn more quickly by pointing out important signs of disease. "For instance, for the problem of distinguishing benign from malignant nodules on CT scans, a 'hand-crafted' feature might be a computerized measurement of the nodule shape, shape being known to be different between benign and malignant nodules," Madabhushi explains.
The researcher insists the AI software suite represents no threat to the livelihoods of human pathologists, radiologists, or other medical professionals. Instead, Madabhushi sees the software as an aide that will greatly automate the process of diagnosis, so doctors can spend more time working with patients. "So you can imagine, for instance, a pathologist coming into her office in the morning and finding a stack of digitally scanned cases rank-ordered by AI in terms of degree of suspicion for malignancy," Madabhushi says. With diagnoses completed by AI, the doctor can immediately start working with the most urgent cases, and then consult with non-urgent patients for whom the AI has found no evidence of cancer.
Madabhushi hopes to bring the AI tools to doctors' offices within the next five years.
Before that, however, the team plans to refine the software suite by training it to recognize slight variations in disease imaging that can crop up depending on the kind of imaging equipment used, or where the imaging was done. "For instance, different labs might use slightly different staining protocols, which can affect the color properties of the tissue slide," Madabhushi says. "MRI scans even for the same patient can look slightly different on scanners from different vendors."
Madabhushi and his colleagues also are working to advance the AI to be able to predict how a specific patient will respond to treatment, and to determine whether a patient will respond to treatment at all. "This could be a game changer in the treatment and management of patients with disease, since knowing which patients will or will not respond might allow for changing the therapy up front, even before initiating the initial choice of therapy," Madabhushi says.
Moreover, they are trying to adapt the software to diagnose a wide range of ailments, including cancers of the prostate, lungs, mouth, and throat.
While Madabhushi acknowledges the formidable power of high-performance computing clusters is needed to train the AI, he says the finished product (or at least an iteration of the software that can be used in doctors' offices with confidence) will be able to run on a desktop computer.
He says in the commercial version, users will not need to train the software; it will already be trained, so they can use it out of the box to identify indications of disease in slide images.
Madabhushi has no idea what the commercial version of the software would cost to purchase, but he is confident it will significantly reduce diagnostic costs for a wide range of diseases and ailments. Traditional computer imaging diagnostics for breast cancer using slide images can currently cost around $4,000, according to Madabhushi; with his software, he suggests the cost could be brought down to as little as $40. "Our hope is to be able to get some of these tests out" to clinics in the next 24 months, Madabhushi says.
Says Nasir Rajpoot, a professor of computational pathology at the University of Warwick in the U.K., "Anant's work has been at the forefront of machine learning. With rapid developments in machine learning and other groups working in this area, watch the space for true realization of precision medicine for cancer."
Anil Parwani, a professor of pathology, and vice chair and director of Anatomic Pathology at Ohio State University, sees tools like Madabhushi's becoming commonplace throughout healthcare during the next decade. "This will be a huge value proposition for the pathologist and other physicians. The pathologist and AI will be much better and superior than the pathologist alone, or AI alone."
Joe Dysart is an Internet speaker and business consultant based in Manhattan, NY, USA
Join the Discussion (0)
Become a Member or Sign In to Post a Comment