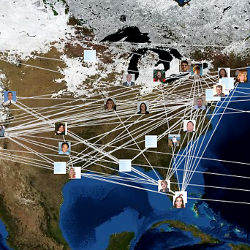
What’s the best way to measure employee productivity in the digital age? From lines of code to number of sales, each industry has its own imperfect standards. Yet according to a new line of thought, the answer may, in fact, lie in your email inbox. And your chat log. And the comments you added to a shared document—in the sum, that is, of your electronic activity. Researchers at IBM and Massachusetts Institute of Technology, for example, analyzed the electronic data of 2,600 business consultants and compared their communication patterns with their billable hours. The conclusion: the average email contact is worth $948 in annual revenue. Of course, it also makes a difference who your contacts are. Consultants with strong ties to executives and project managers generated an average of $7,056 in additional annual revenue, compared with the norm.
According to Redwood City, CA-based Cataphora, one of the companies at the forefront of the movement, the objective is to build patterns of activity—then flag and analyze exceptions.
“We’re interested in modeling behavior,” says Keith Schon, a senior software engineer at Cataphora. “What do people do normally? How do they deviate when they’re not acting normally?” Using data mining techniques that encompass network analysis, sentiment analysis, and clustering, Schon and colleagues analyze the flow of electronic data across an organization. “We’re trying to figure out relationships,” he explains.
Cataphora got its start in the electronic discovery field, where understanding what people know and how that knowledge spreads is critical to legal liability. The company thus works to uncover so-called “shadow networks” of employees who know each other through non-business channels like colleges or churches, or who share a native language, and could collude with one another. Its engineers search for unusual linguistic patterns, and set actual communication networks against official organization charts to determine when people interact with those to whom they have no ostensible work connection.
Yet Cataphora and others are also developing tools to analyze such patterns of behavior in non-investigative settings in the hope of understanding—and enhancing—employee productivity. Microsoft examines internal communications to identify so-called “super connectors,” who communicate frequently with other employees and share information and ideas. Eventually, researchers say, that data could help business leaders make strategic decisions about a project team’s composition, effectiveness, and future growth. Likewise, Google is testing an algorithm that uses employee review data, promotions, and pay histories to identify its workers who feel underused, and therefore are most likely to leave the company. Though Google is reluctant to share details, human resources director Laszlo Bock has said the idea is to get inside people’s heads before they even think about leaving—and to work harder to keep them engaged.
“We have access to unprecedented amounts of data about human activity,” says Sinan Aral, a professor of management sciences at New York University’s Stern School of Business who studies information flow. Of course, not every benefit an individual brings to a company can be captured electronically, “but we can explain a lot,” Aral says. Researchers hasten to add they’re not seeking to punish people for using Facebook at work or making personal phone calls. “The social stuff may be important, and we don’t count that against a person,” says Cataphora’s Schon. In most cases, in fact, personal communications are filtered out and ignored.
Measuring Electronic Productivity
Some measures of electronic productivity are relatively straightforward. Cataphora, for example, seeks to identify blocks of text that are reused, such as a technical explanation or a document template, reasoning that the employees who produce them are making a comparatively greater impact on the company by doing work that others deem valuable. Such text blocks can be difficult to identify, for their language often evolves as they spread through a corporate network. Cataphora has developed a fuzzy search algorithm to detect them, but Schon admits the task is complex. Creating an algorithm that organizes sentences into text blocks, for example, often forces researchers to make inflexible choices about boundaries, using punctuation, length limits, paragraph breaks, or some other scheme. That, in turn, could cause a program to overlook a document whose author formats things differently, such as not breaking the text into paragraphs very frequently or using unconventional punctuation.
Cataphora has also developed a proprietary set of ontologies that cover human resources-related topics, marketing issues, product development, and more to examine various subject-specific communications. One way in which they are useful, Schon explains, is for studying the relationships between people and topics. If an executive is central to communications about product development, marketing, and finance, but marginal to those about sales, it’s likely that she or he is out of the loop when it comes to the newest sales tactics. Ontologies can also identify communications related to particular tasks, such as hiring and performance reviews. From there, engineers can statistically determine what the “normal” procedure is, and see when it is and isn’t followed. Thanks to the training corpus Cataphora has built over time through its clients, these ontologies perform quite well. Yet to detect communication that is specific to a particular industry, location, or research group and whose names can be idiosyncratic, “we may need to examine the workflow and develop more specific ontologies,” says Schon.
Further analysis helps identify how employees influence each other at work. Aral, for example, correlates his electronically derived network topologies with traditional accounting and project data, such as revenues and completion rates, to try to understand which factors enhance or diminish certain outcomes. “The old paradigm was that each employee had a set of characteristics, like skills or education, which he or she brought to a firm,” Aral explains. “Our perspective is that employees are all connected, and that companies build a network of individuals who help each other.” In a study of five years of data from an executive recruiting firm, Aral found that employees who were more central to the firm’s information flow—who communicated more frequently and with a broader number of people—tended to be more productive. It makes a certain amount of sense. “They received more novel information and could make matches and placements more quickly,” Aral notes. In fact, the value of novel information turned out to be quite high. Workers who encountered just 10 novel words more than the average worker were associated with an additional $70 in monthly revenue.
Microsoft examines internal communications to identify so-called “super connectors,” who communicate frequently with fellow employees and share information and ideas.
Yet Aral’s conclusions also point to one of the more challenging aspects of this type of research. If a position in the corporate network is associated with increased productivity, is it because of the nature of that position or because certain kinds of people naturally gravitate toward it? “You always have to question your assumptions,” admits Aral. New statistical techniques are needed, he says, to more accurately distinguish correlation from causation.
Large-scale data mining presents another challenge. IBM’s SmallBlue, which grew out of research at its Watson Business Center, analyzes employees’ electronic data and creates a networked map of who they’re connected to and where their expertise lies. Employees can then search for people with expertise on certain subjects and find the shortest “social path” it would take to connect them. SmallBlue is an invaluable tool for large, international firms, and IBM has used it to connect its 410,000 employees since 2007. Yet indexing the 20-plus million emails and instant messages those employees write is not a trivial task—not to mention the 2 million blog and database entries and 10 million pieces of data that come from knowledge sharing and learning activities. It is the largest publicly known social network dataset in existence, and the project’s founder, Ching-Yung Lin, says IBM worked hard to design a database that would hold different types of data and dynamically index the graphs that are generated.
Proponents of electronic productivity analysis say the markers are best used to augment, rather than replace, traditional metrics and peer evaluations. “It’s a sanity check,” asserts Schon. In the future, predicts Aral, who is helping IBM refine SmallBlue, the software could provide real-time, expertise-based recommendations: automatically suggesting connections while employees work on a particular task, for example, or helping managers assemble compatible project teams.
Aral, S., Brynjolfsson, E., and Van Alstyne, M.
Information, technology and information worker productivity. International Conference on Information Systems, Milwaukee, WI, 2006.
Manning, C.D., Raghavan, P., and Schütze, H.
Introduction to Information Retrieval. Cambridge University Press, New York, 2008.
Mikawa, S., Cunnington, S., and Gaskis, S.
Removing barriers to trust in distributed teams: understanding cultural differences and strengthening social ties. International Workshop on Intercultural Collaboration, Palo Alto, CA, 2009.
Wasserman, S. and Faust, K.
Social Network Analysis: Methods and Applications. Cambridge University Press, Cambridge, 1994.
Wu, L., Lin, C.-Y., Aral, S., and Brynjolfsson, E.
Value of social network: a large-scale analysis on network structure impact to financial revenue of information technology consultants. Winter Information Systems Conference, Salt Lake City, UT, 2009.
Join the Discussion (0)
Become a Member or Sign In to Post a Comment