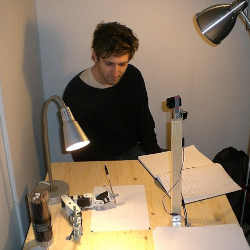
About 15 years ago, Frederic Fol Leymarie and a colleague developed a robot called Aikon that can draw portraits of people. The system uses a camera to capture a person's face as they sit in front of it. Information about the image is converted into commands that are sent to a robotic arm equipped with a pen so it can sketch the person's face. "The objective was simply to try to achieve an aesthetic that was similar to a human aesthetic," says Fol Leymarie, a professor of computer science at Goldsmiths, University of London in the U.K.
At the time, Aikon was groundbreaking due to the human-like quality of its sketches, which was achieved partly by the imprecise strokes of its rudimentary robot arm. Today, artificial intelligence (AI) systems can produce artifacts of much higher quality: OpenAI's DALL-E2 system can generate photorealistic images when a human provides a short description, for example, while companies such as AIVA Technologies have developed AIs that can compose original music. This is prompting researchers to consider deeper questions such as what the creative potential of AI could be and how it can be quantified.
"I think creativity is a behavior that needs to be understood before we even start making claims that [AI models] are creative," says Payel Das, an AI researcher at the IBM Thomas J. Watson Research Center in Yorktown Heights, NY. "So for an AI agent or for a machine learning model, what does it even mean to be creative?"
When trying to evaluate AI creativity, researchers typically refer to the work of British cognitive scientist Margaret Boden, a key player in the field who identified three types of creativity. Combinatorial creativity involves bringing together existing ideas in new ways, while exploratory creativity consists of generating new ideas within a specific conceptual space, such as making improvements to an existing object. Transformative creativity is the most radical, and involves coming up with ideas that are fundamentally different from existing ones. "A lot of the current dimensions of creativity being explored, either in human or in AI agents, probably fall more into the [combinatorial] category," says Das.
Researchers are now trying to come up with metrics to quantify AI creativity. The first step is to define creativity, which can be a challenge since over 100 different definitions have been proposed over the years. In the past, it has often been considered as a mysterious and uniquely human trait that cannot be explained scientifically. Certain elements of the creative process, such as taking inspiration from lived experiences and self-awareness, are sometimes considered to be important contributing factors. "At the moment, this is something that is very hard to reproduce in a machine," says Mirco Musolesi, a computer science professor at University College London in the U.K.
However, Boden's famous definition, which considers creativity to be the ability to come up with artifacts that are new, surprising, and valuable, is typically chosen when trying to assess the trait in AI systems. In recent work, Musolesi and his colleague have come up with a way to measure the three elements in this definition by using deep learning. Their goal was to create an automated approach that wouldn't require human judgment to assess the creativity of artifacts produced by generative algorithms. "When you have a problem that has a lot of dimensions, deep learning is good for that because it is able to capture information and learn from that," says Musolesi.
In an initial experiment to test their method, the team focused on American poetry and trained two types of neural networks using 2,676 poems from the 19th Century that were publicly available. They then tested the models to see if they could predict the creativity of a subset of the training data that was not previously seen. Further tests also used a dataset of poems from the 20th century and another consisting of poems from the 17th and 18th centuries.
Musolesi says the models performed well with respect to the training sets: they could capture to a certain extent how the creativity of poems changed over different historical periods. Poems would have a low rating in terms of surprise, for example, if their themes, such as love, were similar to those in the training set. Musolesi stresses that the work is preliminary, since there are several limitations: one is the relatively small size of the training dataset, which represents a limited number of poems. Another constraint is that the system only considers the creativity of the style or genre, without assessing other aspects, such as the words used.
Musolesi and his colleague anticipate their system could be incorporated into the generative process of AI models. A model could be trained to maximize its creativity, or its output could be evaluated using the approach as part of an iterative process. "Since we are generating [creative products] using such deep learning techniques, it is quite natural also to use it to judge it," says Musolesi. "You can use machines to judge machines."
Another team has come up with a metric to characterize the creativity of generative models that is inspired by neuroscience. Research that involved scanning people's brains while they complete creative tasks has shown that the creativity of their output is linked to differences in brain activity. Das and her colleagues therefore hypothesized that the creativity of AI models could also be detected from activation patterns in the artificial neural networks that make up deep learning algorithms by using a method called group-based subset scanning. Since generative AI models are designed to mimic training data, differences in certain types of activity while they are producing artifacts should indicate creativity. "We look for anomalous patterns," says Das.
In experiments with a generative AI model and different image datasets, Das and her team showed that atypical activation did correlate with more novel and meaningful generated images. Humans asked to rate the creativity of the output saw their scores largely match what was predicted from the activation patterns.
Das and her team are now interested in how machine creativity can be controlled. Current generative models are powerful, but their creative output varies in terms of its value to humans and society. When used to help design new drugs, for example, they may propose solutions that are creative, but they must also meet other criteria, such as not being toxic. "The challenge from now on is going to be how we steer them towards good creativity," says Das.
So far, AI creativity has focused on systems generating digital artifacts. However, Fol Leymarie, thinks interest will soon spread to robots producing physical objects such as paintings or sculptures, which are starting to move beyond the research and development stage. In a new project, he and his colleagues are investigating how robots and AI are influencing creativity in visual art, partly by trying to characterize the creativity of state-of-the art technologies.
"We should be ready for a similar sort of revolution in the next few years," he says.
Sandrine Ceurstemont is a freelance science writer based in London, U.K.
Join the Discussion (0)
Become a Member or Sign In to Post a Comment