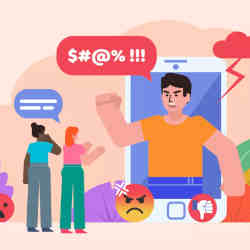
Venture online and participate in a discussion and it is likely that you will soon encounter mean, abusive, or ugly language. Virtual sniping, trolling, and flame wars are an inescapable part of the online world. "People are genuinely awful to each other," observes Eric Goldman, a professor of law at the Santa Clara University School of Law.
It is a problem that has been extraordinarily difficult to solve. However, "If we want to create a culture of inclusion and support a democracy, we have to create spaces where people are civil and where they behave in a way that is broadly defined as appropriate," says Libby Hemphill, an associate professor at the School of Information at the University of Michigan.
The challenge, of course, is to establish effective rules and controls for each site and situation. However, even with clear standards, it is tough for human moderators to keep up, and for built-in controls to work consistently. Too often, conversations deteriorate, participants toss verbal grenades, and a discussion group devolves into nastiness and chaos.
As a result, researchers and computer scientists are studying ways to improve online interaction and communication using new methods and techniques. They are developing better artificial intelligence (AI), machine scoring systems, and frameworks that support both machine and human involvement.
Words Matter
A fundamental problem with today's policing framework is that it typically reduces complex situations to a simple "leave it" or "remove it" mentality. Hemphill describes this approach as "a blunt instrument" that often doesn't serve the best interests of a community. "It also doesn't address the root problem and do anything to improve behavior," she says.
Hemphill and others believe it is time for change. A more nuanced approach would not only identify offending content, but guide participants toward more inclusive discussions. Ultimately, "We need to alert people that they are potentially hurting others and that they have crossed a line," she explains.
This might mean flagging words and sentence structure differently in a forum for, say, LGBTQI participants or a group of black or Hispanic members—while also constructing systems that recognize humor and sarcasm. "The same words and terms can be used as a point of reference or as weapons," Hemphill says.
Finding ways to aid human moderators is also at the center of research. Sarah T. Roberts, an assistant professor in the Department of Information Studies at the University of California, Los Angeles, says algorithms and machine scoring systems can work in conjunction with more traditional moderation methods—especially when the humans are visible. She points to Reddit Subgroups and Wikipedia as examples of fairly "successful ecosystems."
Digital Neighborhood Watch
Wikipedia and Reddit Subreddits allow groups to establish community standards and include checks and balances to improve discussions. The latter site, for example, allows moderators to adjust the level of tolerance to the group—and institute specific guidelines and rules—to keep conversations on track. Participants vote up and down on posts, and comments that don't meet community standards can be voted off the board.
This approach—think of it as a digital "neighborhood watch"—effectively blends human and computer interaction. In addition, a few sites—social media network Nextdoor, for example—have introduced popups that detect when a participant may be out of bounds and suggest different language. Nextdoor found the number of "unkind comments" dropped 25% as a result of using these "kindness reminders."
Roberts believes these approaches serve as a starting point for more advanced human-machine frameworks. "We have to think of more creative ways to address the issue. The goal should not be to simply delete people or block them because they haven't met norms and expectations. The goal is to get the conversation back on track, whenever possible."
Consequently, researchers are beginning to study how AI and machine learning can be used to not only identify problem conversations, but also to incorporate restorative justice. For example, a system might offer incentives for abusers to change their behavior, Roberts says. Instead of relying strictly on a punitive approach, a system might let a person push a reset button after a cooling off period and after receiving information about how to better communicate online.
Another idea, Hemphill says, is giving participants greater latitude about setting their own thresholds. Just as search engines like Google and Bing can filter sensitive content, based on a user's desires, an individual could slide a dial. "We all have times when we are more or less sensitive to what we view as abrasive or caustic remarks," she explains. Goldman believes that more thought also needs to go into site design. "Frictionless interaction isn't always desirable. In some cases, slowing things down may be beneficial."
Of course, as long as there are humans, the problem won't ever go away completely. Concludes Goldman: "We have to find better levers to encourage social behavior and discourage anti-social behavior."
Samuel Greengard is an author and journalist based in West Linn, OR, USA.
Join the Discussion (0)
Become a Member or Sign In to Post a Comment