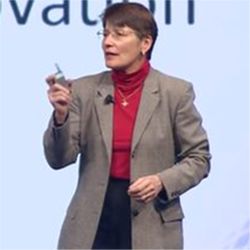
“Think about how you got here,” said Katharine Frase, formerly IBM CTO and more recently head of strategy and business development for IBM’s Watson Education unit, in her keynote at the SC16 (supercomputing) conference in Salt Lake City last week. Most of us, she said, “relied on our phones or some other device to wake us up, remind us what we were doing today, update us on what happened overnight in our public or private network, track our steps on the treadmill,” and for those who drove, “you relied on your phone or some other form of GPS. It helped you navigate. It recalculated when you missed that turn. Or you relied on up-to-minute social information to help you avoid that accident up ahead.”
Now imagine, she said, you started out at the conference; “Almost everything I just said was not true. So almost without realizing it, how we live our lives every day has been transformed by the use of data, by the use of devices, by the assumption those devices will be up to date, adaptable, and, to some degree, learn our preferences.”
Frase said what she really came to talk about in her keynote speech “Cognitive Computing: How Can We Accelerate Human Decision Making, Creativity and Innovation Using Techniques from Watson and Beyond?” was how to “take us beyond answers, however adaptive, flexible, or real time, to an exploration of how do we move toward co-creation, co-hypothesizing. How do we work with systems that can help us ask the right questions, not just find the right answers?”
The challenge of big data, she said, “is not always that it’s big… People in this room have been extracting insight from enormous datasets of digital structured data for generations, insights that have had enormous value in every aspect of science, technology, industry. But it’s not the structured data that we’re drowning in and that is exploding. It’s the unstructured stuff. It’s the variety of data, different modalities, different link scales. It’s the velocity of the data. It’s flying at us all the time faster than we can process it. How do we separate the signal from the noise in all the sensor data that flies at us?” Not to mention the “veracity, truthfulness, or noisiness” of that signal?
We also, she said, “have a number of ways of thinking about the history of computing. In rooms like this we have a tendency to think about it as an architecture statement—mainframe, client, server, PC—or a technology statement—single core, multicore—but I’d like you to think in another way—in terms of how humans and systems have interacted. So the first generation of what we would now think of as early computers were really tabulators. They counted things. Really important things, for example, whether we could ever have done the social security system without some data system behind it is a big question. So there’s an era of tabulation, which we now almost forget about it because it feels so routine.”
The second era, she said, “which we’re still in,” is “50 years of programmable systems I would characterize in three ways. The first is that, by design, it is going to give you the same reliable answer to the same question every time you ask it. That’s enormously valuable. It’s how we all run our businesses. It’s here to stay. The second is we humans to some degree decided what those questions were going to be in order to structure what the system knew how to answer. The third is that we’ve spent 50 years teaching humans to speak the way a computer thinks. Which is really what programming is.”
Now, she asked, “What do we mean when we talk about a cognitive system? What’s different in a cognitive system?" The first thing, she said, is that "it understands language the way people use it, including through natural language processing and machine learning. The second is it learns rather than being programmed. So the system continues to get smarter day on day, which means (if we give it the right feedback) it can give us a different answer depending on our context. Depending on where I am or when it is, I may want a different answer to that question. And the system needs to know that about me” … also that it responds to the most recent information.
“So said another way,” she said, “cognitive systems understand not just language (although it’s where we started) but imagery, sounds, other forms of unstructured information. It can reason. It can extract concepts out of that unstructured information. It learns. It continues to improve. It’s not omniscient, but it improves its statistical understanding of a concept, and it interacts with humans in a dialog-driven, symbiotic kind of way.”
Another trend is massive datasets, though they do not, said Frase, “mean all the data is good, particularly when I look at some of the social media data; hmm, is it really even data?” Some are curated datasets, some are noisy datasets, but “increasing size of the datasets with which you can train a system, whether it’s for speech, language, or images.” A number of projects are underway, she said, “to see whether you could have a system that could pass the radiology exam. Really difficult… but difficult even for humans. So big datasets in which to train.” But these are not omniscient systems; “you can’t,” she said, “hand them all your unstructured information and magically they will know the right answer tomorrow. This is a co-recreated kind of thing.”
Add to that, she said, “with all credit to people in this room, the dramatic improvement in performance, improvement in density, and drop in cost of pure computational power. None of this would be possible without it.” None of the things she was talking about, she said, as to how we got here this morning “would be possible without it. Let alone these higher-level problems.”
“Why,” she asked, “does moving forward in these new relationships with systems matter?” She then mentioned that she had been involved with the Watson system when it played Jeopardy! on television, then “figuring out, 'oh no, we won, now what?'” She said, “because we thought Jeopardy! had been a difficult problem, we thought we’d take an easier first leap into the real world, so we tackled oncology.” So, she said, “We unplugged Shakespeare and the Bible” as “core diagnostic references” and plugged in the Merck Index, The New England Journal of Medicine, and other relevant resources, and started training the system. Some of that was to get the system to recognize “the vocabulary of health. What is a symptom? What is a diagnosis? What is a treatment?” Some of it was adding new features. “When you play Jeopardy!,” she said, “time doesn’t matter, except how fast you answer the questions. But in health, if you get the fever before you get the rash, it really does matter.”
“Now, what other domain has the same characteristics as healthcare,” she asked, “massive, affects every human being, fragmented, not as data-driven as we wish, huge economic impact, feels intractable?” Education, for one. To illustrate, she said, “Some of you will have heard people at IBM talk about it as our second moonshot.” After “Can cognitive computing help healthcare?” the second moonshot is “Can it help education?” In the U.S., she said, “we are painfully aware of the unsatisfactory numbers for high school graduation rates, college graduation rates, college debt, employment of young people, our ability to retrain adults when their world changes, our ability to retrain and reintegrate into society our veterans. Those are daunting problems.”
In healthcare, she said, “we wish we had more data than we do. We have great data in some places and spotty data in many other places. Education is data-poor. If you think about the notion of a clinical trial… in education there’s no such thing.” But, she said, “one of the things we absolutely know, and we know it about ourselves, we know it about our children … the more engaged a student is the better they learn. Because they become learners, rather than repeaters. They become explorers and discovers. It’s the Wikipedia effect. 'Ooh, I’ll read this, I’ll read that.'” So therefore “I figure,” she said, “a big technical conference like this needs Big Bird. What can we do with the very youngest learner? Sesame Workshop has done marvels over 40 years, in early literacy, early school readiness, and they would say that one of their goals is not just to create smarter children but kinder children. Sesame has tackled a lot of really tough societal issues in a format that kids can handle—and maybe help their parents, too. But it’s been a broadcast mode, and I mean that not because it’s just television. As much as we each love Elmo, it’s the same Elmo for every child. But what if it weren’t?”
She concluded, “This [high-performance computing] community has done amazing things for technology- and data-driven industries and science… We also have the capability to start addressing huge societal issues, systems that affect every human being and every economy on the globe. It’s not just esoteric computer science for those of us who love to work on machine learning or natural language processing. It is a collaborative effort that includes psychologists and domain experts and designers and all those people we never thought we used to have to talk to.”
It is, she said, “… a community effort that could have enormous value to society and to how we look at our own reflections in the bathroom mirror. I invite you into the era of cognitive computing.”
Andrew Rosenbloom is senior editor of Communications.
Join the Discussion (0)
Become a Member or Sign In to Post a Comment