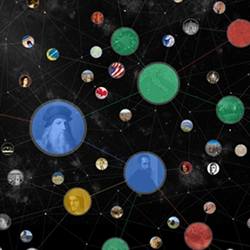
One of the biggest problems with science today is its sheer bulk; not in the context of something as complex as Kurzweilian singularities, but simply regarding plain old "finding the needle in the haystack." Scholarly papers are published every day, and no one can keep track of them all without a legion of helpers to pore through them all and identify the best (or those with new or unexplored findings), or a big-buck account with a supercomputer that can read them all and rank them in accordance with your priorities.
A more reasonable solution to the problem is the growing popularity of neural networks that can learn from experience. For instance, Meta, a self-described "scientific knowledge network powered by machine intelligence," has built a gigantic knowledge graph (usually acknowledged as an invention of Google, which amalgamates semantic-search information from a wide variety of sources to provide a structured knowledge base about a topic; the search engine provider uses it to provide spoken answers to Google Now searches).
Meta’s Knowledge Graph underpins both its free discovery platform for researchers (Meta.Science) and its commercial products and services, which include Bibliometric Intelligence and Sales & Marketing Analytics.
Svetlana Sicular, research director at information technology research and advisory firm Gartner, said her firm "has only had a single presentation from them so far, so I can not yet give you an informed opinion, but I am very intrigued by Meta’s approach."
Toronto, Canada-based Meta was launched in 2010 as Sciencescape Inc. With its name change, the company upgraded its Sciencescape prototype predictor to what it calls MetaScience, which does a deep dive into the literature knowledge graph to deliver a new type of user experience: predicting the future, from tomorrow to years from now.
The company modeled its original knowledge graph technology upon the published descriptions of Google Scholar and Google Brain (but has no business association with Google). The latest incarnation of its proprietary MetaScience predictor now is called Meta Horizon Scanning.
"We are working with our first customers on Meta Horizon Scanning. There is still ongoing R&D work underway that will produce an update later this year, and we’ll be incorporating that update into our offering at that time," said Meta CEO Sam Molyneux.
Meta uses its proprietary version of a knowledge graph, based on "a network of researchers and entities derived from the entirety of PubMed, over 25 million full-text biomedical research papers, and the Web," to relate the different associated ideas in a research field, be it computer science or electrical engineering or medical diagnosis. It started with the latter, and this year will select a content area for its next knowledge graph project based on user input (you can cast your own vote at Meta’s hello-user mailbox).
"We are expanding into physics right now, and want to expand into computer science, engineering, chemistry, and other fields, but are trying to decide which comes first right now," said Meta chief science officer Ofer Shai.
So far, Meta has partnerships with 30 major publishers worldwide, each of which feed it a stream of newly submitted scholarly papers that Meta’s apps rank by importance, as well as recommending which specific journals at that publisher would be most like interested in the paper’s topic (all calculated from Meta’s gigantic knowledge graph).
"Publishers love us, because we incorporate as many as 4,000 submissions a day, which is overwhelming for a publisher’s staff," said Molyneux. "whereas we can narrow down the most important papers almost instantly, and even target which paper’s topic is most appropriate for each of a publisher’s journals."
Meta’s knowledge graph already has tens of millions of entries, each associated with even more connections to associated nodes mainly organized around researchers, journals, and concepts (with over 20 subcategories)–all generated from the historical papers in an area. It adds a steady stream of new academic paper submissions that help fine-tune and keep the knowledge graph up-to-date in real time. Meta is also building apps to mine its knowledge base in different ways it thinks will be useful to publishers, journals and to the researchers themselves.
"We are building many new apps using neural networks with deep machine learning on the docket; deep learning is just a neural network with many, many layers," Molyneux said. "One is called Bibliometric Intelligence, which inspects new, unpublished manuscripts using over 200 factors, and recommends which journal worldwide would be most likely to publish them."
Meta is moving from data mining to prediction, a new capability that will allow subscribers to anticipate the future of a research area with intelligent suggestions about what the most popular, most needed, or even most profitable directions an academic or even industrial researcher might want to consider.
Meta is also developing apps with visualization interfaces to present overviews of the state of science that can be moved forward and backward in time, like radar weather maps. These Knowledge Graph Analytics seek answers to questions like "who are the leading researchers working on a specific problem, which approaches seem most promising, and who are researchers that are working in that area," Molyneux said.
"We do it by using something like business analytics to look at a knowledge graph in a different way," Molyneux said. "Whether you are researcher, a grant provider, or just a professional, you need to be asking these kind of questions today, whether you are looking for researchers or asking where a research area is going to be in three to five years."
A Meta application under development, Horizon Scanning, applies predictive analytics that operate on top of a knowledge graph to calculate future prominent entities–properties Meta calls "emergent."
R. Colin Johnson is a Kyoto Prize Fellow who has worked as a technology journalist for two decades.
Join the Discussion (0)
Become a Member or Sign In to Post a Comment