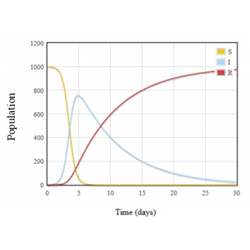
If a sick person fails to take a fully prescribed dose of antibiotics for a bacterial infection, it is commonly accepted the disease may come back, more resistant and robust than it was previously.
That concept may appear to be at work at population levels in a new model of infectious disease, though one of the theory's authors says it's a little more complicated than that. However, the outcome of the model—which demonstrates that halting temporary control measures, such as spraying insecticide that kills disease-bearing mosquitoes, could result in outbreaks more severe than if no control measures at all had been employed—is evidence that computational mathematicians and biologists are developing sophisticated guidance for public health officials in ways that weren't possible even five years ago.
One of the new theory's authors, Alun Lloyd, Drexel Professor of Mathematics at North Carolina State University, said, "I'll just say this about our results: they were sufficiently counterintuitive to us that we didn't believe it at first. We found this result in a much more complicated model which is much less transparent and easy to understand, and that model was sufficiently complicated that we wondered for a while if we actually had a mistake in our code."
Lloyd said such work is representative of an increased symbiosis between mathematical modelers and real-world information that can fuel those models. Paramount among the issues facing the public health research community now is how to determine what data best fits into a given model, and how best to validate each using the other.
"One of the ways in which the field has developed in my time—not because of me, I've just observed this—is these models have become a lot more connected to data," he said. "The enhanced connections work both ways because the data can help inform the model. Maybe sometimes it will show us where the models are working well or not working well, but also you can use the models to tell you about what sort of data you would need to be able to make those improvements."
Lloyd and his colleagues presented their findings at the Society for Industrial and Applied Mathematics conference on dynamical systems in May, in a presentation called "After the Honeymoon, the Divorce: Unexpected Outcomes of Disease Control Measures." Unlike vaccination campaigns, which give recipients permanent immunity, transient controls such as spraying of insecticides do not offer immunity to people who are added to a population after such measures are halted.
The team's findings have not been published in full yet, and Lloyd said there may be some resistance to them when they come out.
"I hesitate to say this; I can imagine there might be people who wouldn't be happy about the fact that we are saying doing something can be worse than doing nothing, so I expect to get some pushback as a result," he said. "Of course, we would never say 'Do nothing.' We are saying you need to think about these things and maybe design your intervention with this in mind, or maybe even design your observation or surveillance of a system with this in mind."
Coupling data sources
Moritz Kraemer, a research fellow at Harvard University Medical School and Boston Children's Hospital, is among those in the foreground of discerning what types of data might be most relevant to trying to stem a disease outbreak, and when that data is best introduced into a epidemiological investigation.
For example, he said, official channel reporting of the recent Zika virus in Brazil could take four to six weeks from the initial observations of an outbreak to final dissemination of data and recommendations from the national government back to local public health officials.
"That's why non-traditional data sources are very relevant early, where you have no one talking about it except the local people in the field, and they can then communicate the information they have," Kraemer said. "But later on, if you look at the actual distribution of Zika reports from non-traditional data sources, they would pop up in all regions; for example, from northern Canada, where we know from an ecological perspective that Zika can not be transmitted by a mosquito.
"That's one takeaway from that particular example," Kraemer said. "The coupling of things is now on the research agenda. It's not just relying on one data source alone. That data source for a long time was traditional health records, and then things moved into a 'Data can tell us everything' perspective. The community I work in is realizing we need to have a smart way of integrating this all together. It makes sense, because if you only have the traditional sources, it takes a long time and is very sparse. If all you have is digital, it may be all over the place and nobody has contextualized what it means."
The coupling of data Kraemer speaks of can take the form of empirical network data one might consider as traditional, such as geolocation data of mobile phones in the wake of the 2010 Haitian earthquake. That data signaled which areas received the largest influx of refugees from the stricken area around Port-Au-Prince and enabled relief agencies to respond more quickly and efficiently.
Urs Gattiker, founder of CyTRAP Labs, a Zurich, Switzerland, developer of blog analytics software, said the lesson to be taken from studies such as one using the Haiti data is that such data must be available as close to real time as possible.
"The crux of the matter is, one must get access to these data rather quickly, or preferably in real time, and regulators must move quickly," Gattiker wrote in a blog post examining new big data approaches in public health. "One challenge is that researchers are often elsewhere, so regulators need to find a way to give them access. Of course, neither violating local regulation nor mobile phone users' rights, such as privacy, is an option."
However, even data created for purposes far removed from basic tracking can help measure population movements and discern whether those movements might lead to greater disease risk. For instance, Kraemer and some colleagues have pointed out that "novel" data sources, such as augmented reality games like Pokemon Go, could be overlaid with data on where Zika was reported in Miami, to provide game players and designers with information that could aid precautions against becoming infected.
"It was kind of a neat example of the ability to use some of these augmented reality and network data in the context of public health," Kraemer said. "You have to be careful with these things, because it's a very early exploration of data and we tried to make it clear that this is in no way something conclusive."
Adding by subtracting
One of the principal points of the "After the Honeymoon" research is not that it added a data source, novel or otherwise, to reach its hypothesis, but rather took away a variable, according to co-author Kenichi Okamoto. The SIAM research was an extension of an earlier paper co-authored in part by Okamoto (who served as lead author) and Lloyd.
"We took away the element of a vector (a transmitting insect) in our model," Okamoto, who was a researcher at Yale University when the work was written, said. "We as modelers work under the premise that the more general you can make your claims, the more widely appreciated they will be."
The next step in advancing the work will be testing the model's theory with empirical data. Exactly where that data will come from is still open, and demonstrates the widening options available to researchers, as well as the necessity to rigorously examine the most accurate approaches.
"Once we start confronting the predictions with more empirical data, it will become clear as to what kinds of data sources we can integrate," Okamoto said. "I have been wondering if there is a way to address this experimentally. The classic tradition in theoretical epidemiology is to confront models with experimental data in mice. I think it would be nice to be able to bridge theory to the experiment and then confront the clinical data that would be out there, from my perspective. This approach is not widely practiced anymore for a variety of reasons, but this is an instance where the hypothesis might benefit from it."
Okamoto, now a professor at the University of St. Thomas in St. Paul, MN, said his colleagues are exploring how to better integrate the fields of biology and public health with a computational mindset.
"They are thinking about how to encourage not just biology majors but also people going into clinical settings, to have more quantitative literacy and more of a computational approach to thinking about problems. I think that means breaking the problem down to natural components, and the particular software platform or tool or whatever you use is very easy to pick up. The hard part is getting clinicians and people on the ground to think about how it is computational problems are solved and how to integrate data into problem solving."
Gregory Goth is an Oakville, CT-based writer who specializes in science and technology.
Join the Discussion (0)
Become a Member or Sign In to Post a Comment