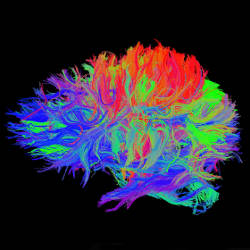
There is, as yet, no cure for Alzheimer’s disease, and little in the way of treatment. But computer models that can predict the course of the illness, which gradually destroys memory and other cognitive functions, might allow doctors to manage the disease and perhaps help scientists to better understand it. Computer modeling might, in fact, lead to clearer prognoses and better treatment for a whole range of brain disorders, from Parkinson’s disease to brain cancer.
One group of scientists, from Weill Cornell Medical College in New York and the University of California, San Francisco, developed a model that starts with a magnetic resonance image of the early stages of Alzheimer’s or frontotemporal dementia and predicts how the illnesses would spread throughout the brain.
“We assume that the disease in the brain diffuses from one place to another as if it were a gas,” says Ashish Raj, assistant professor of computer science in radiology at Weill Cornell. But instead of spreading through open air, the “gas” in question had to travel along the brain’s neural pathways. “We had to come up with the right kind of math that would model how something diffuses in a network rather than in space.”
The model works on the idea, arising from previous studies by other scientists, that these illnesses begin in one part of the brain and spread along a network of neurons. Scientists have recently learned that dementia seems to behave as if it is caused by an errant protein, known as a prion. Prions are known to cause the brain illness Creutzfeldt-Jakob disease, the human form of mad cow disease. Proteins normally fold into shapes that dictate their function in the body, but prions are misfolded, and can transmit the misfolding to healthy proteins, causing them to form into plaques called amyloids that destroy brain tissue and seem to play a role not only in Alzheimer’s but also in other degenerative brain diseases such as Huntington’s and Parkinson’s.
Raj and Michael Weiner, professor of radiology and biomedical imaging at UCSF, mapped out how proteins are dispersed in healthy brains, then applied the assumption that faulty proteins would spread from one area to another. To determine the rate at which they would spread, they looked at how the prevalence of dementia varies by age, which should be related to how long the disease takes to develop. They ran the model, then compared the results to actual brain images from 18 patients with Alzheimer’s and 18 with frontotemporal dementia, and found the model matched reality. Those results, gleaned by bootstrap analysis of their data, seems to validate the prion hypothesis, Raj says.
In practical use, the model should be able to look at a single scan from a dementia patient, figure out where he or she is in the course of their disease, and predict its progression. That might help doctors and patients make decisions about the few treatment options that exist.
Marc Diamond, associate professor of neurology at Washington University in St. Louis School of Medicine, says Raj’s model agrees with his studies, in cells and mouse brains, of the molecular mechanisms for Alzheimer’s spread. “The two are very consistent with one another,” Diamond says. “They are basically saying the network involvement in some of these diseases is consistent with an agent that moves between neurons, based on how they’re connected to each other.”
The computer model has limitations, Diamond says; he calls it a “30,000-foot view of the whole process” that does not explain why disease might not spread between some connected neurons. But he adds it might help provide researchers with hints as to regions of the brain they ought to study with other methods.
The MRI scans came from the Alzheimer’s Disease Neuroimaging Initiative, run by Weiner, which is collecting brain images that may help scientists understand the course of the disease. The patients in question have only been followed for two to four years, and Raj says it will be helpful to have images that cover a decade or two. But even over that shorter time span, an incorrect model would diverge from the actual images, which this model did not, he explains.
One advantage of the model, he says, is its simplicity. It ignores whatever complex activities may be taking place at a cellular level and focuses on diffusion patterns to get an accurate picture of disease progress. That, says Raj, is an example of how computer scientists can sometimes aid other scientists.
The computer model might help provide researchers with hints as to regions of the brain they ought to study with other methods.
Another advantage is that the model relies on well-understood principles. “We have used a set of tools from graph theory that really are quite well known, but have found a way that was quite unexpected to apply them to disease,” Raj says. His group is not the only one using that sort of modeling to study the communications network in the brain and how it relates to dementia. Neurologists at VU University Medical Center in Amsterdam also applied graph theory to examine the differences in connectivity in the neural networks of healthy brains versus Alzheimer’s brains.
They started with the idea that healthy brains may follow a small world network model, in which points in the network connect to several other points, and hubs with many connections link one cluster of connections to many others. What they found, says Willem de Haan, one of the researchers involved in the work, was that connections in the brains of Alzheimer’s patients showed a less ordered series of connections than healthy brains. Then they looked at patterns of brain activity from electroencephalograms to see if there was any link between activity levels and areas that were damaged by amyloid plaques.
“The hub in the network, the well-connected points, seemed vulnerable for some reason,” de Haan says. “We wanted to try to explain how these hub areas became vulnerable.”
Operating on the hypothesis that areas of high neural activity were more likely to suffer the damage that leads to dementia, the group employed a neural mass model, a simplified model that focuses on only a few variables to describe the activity of a population, or mass, of neurons. They then applied an algorithm that “damaged” the masses, reducing the number of signal-transmitting synapses based on the amount of activity in that particular area. They then used graph theory to analyze the results, and found the outcomes matched those in Alzheimer’s patients.
The computer model provides a theory to be tested in actual tissue, de Haan says. And it might suggest a possible treatment to ward off the early effects of the disease. If increased brain activity does lead to structural damage, perhaps methods that alter activity levels, through the use of electrical or magnetic stimulation, might slow the disease’s progression. The researchers would also like to look at scans of people in the early stages of Alzheimer’s to see if they can find the differences in activity levels that the computer model predicts.
Another Path
Kristin Rae Swanson, a professor of neurological surgery at Northwestern University’s Brain Tumor Institute, also combines brain scans and mathematical modeling to predict how areas of damage spread through the brain. But instead of misfolded proteins, she is looking at the spread of glioblastoma, a deadly brain tumor. All glioblastoma patients receive MRIs and are treated with radiation and perhaps brain surgery, but Swanson says her models can identify parts of the tumor that the scans cannot pick up, and predict how a tumor will spread through a particular patient’s brain. That in turn could lead to individualized treatments that could prolong a patient’s life and minimize side effects of excessive radiation treatment. “It’s a different approach to personalized medicine,” she says.
MRIs only pick up the densest part of a tumor and miss cells that are more sparsely concentrated; Swanson compares it to looking at an iceberg from above. The model takes advantage of what is known about the cancer’s proliferation rate (how quickly the cells reproduce) and its diffusion rate (how quickly they move through the brain), which is faster in the white matter than the gray matter. It also takes into account that cells tend to move along the blood vessels and fiber tracts in the white matter. So by looking at the shape of the iceberg, her model can predict where the invisible parts lie, so that a doctor can target them. And by seeing which parts of the brain’s structure are affected, it predicts how the tumor will grow.
MRIs only pick up the densest part of a tumor and miss cells that are more sparsely concentrated.
Raj believes his model could also be used to study the dynamics of brain development, looking not at just where brain connections go wrong, but how they form in the first place. It might also be applied to epilepsy, in which what is diffusing through the brain is not a misfolded protein but a disordered electrical signal. In fact, it should work with any brain ailment that is characterized by something spreading throughout the brain.
Further Reading
De Haan, W., Mott, K., Van Straaten, E.C.W., Scheltens, P; and Stam, C.J.
Activity dependent degeneration explains hub vulnerability in Alzheimer’s disease, PLOS Computational Biology, Aug. 16, 2012.
Duch, W.
Computational models of dementia and neurological problems, Methods Mol Biol. 401, 2007.
Harpold, H.L.P., Alvord, E.C. Jr. and Swanson, K.R.
The evolution of mathematical modeling of glioma proliferation and invasion, J Neuropathol Exp Neurol 66, Jan. 2007.
Raj. A., Kuceyeski. A. and Weiner M.W.
A network diffusion model of disease progression in dementia, Neuron 73, Mar. 22, 2012.
Weiner, M.
Dr. Michael Weiner, MRI specialist, San Francisco Vets Admin http://www.youtube.com/watch?v=aNo-KwmReiA
Join the Discussion (0)
Become a Member or Sign In to Post a Comment