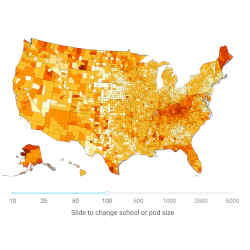
In the initial days of the COVID-19 pandemic, a new kind of computational modeling theory began to emerge: instead of relying on traditional public health data as used in "tried and true" SIR (Susceptible, Infected, Recovered) models, new types of data, such as aggregated mobility data gleaned from smartphone GPS signals, helped policymakers and scientists alike begin to make sense of the dangerous and deadly effects of the novel virus.
The fast spread of the virus and the drastic effects it had on daily life were, it turned out, a tailor-made field of possibility for academic computer scientists and public health researchers, who ramped up complex models very quickly.
"In the beginning of the pandemic, there were a lot of national-level data models, and in our virtual water cooler talks we said that was ridiculous," David Rubin, M.D., director of the Children's Hospital of Philadelphia (CHOP) PolicyLab, which undertook localized data modeling on a national scale during the pandemic. "The fact that New York City was surging didn't mean that Montgomery, AL, was surging, so we developed a model that grew to about 800 counties. With lots of local variables incorporated every week, we started to calibrate a model that was more like a weather forecast for a local area, and I think that was the real value of the approach we took."
Other leading-edge modeling efforts also emerged, such as the University of Texas (UT) COVID-19 Modeling Consortium, and the University of Virginia (UVA) Biocomplexity Institute. Among the results of the UT consortium was a staged alert system that treaded a careful line between restrictive measures and keeping society open, forecasting hospital demand on that fine line. At UVA, Biocomplexity Institute researchers began modeling the spread of the disease in January 2020 and had already produced predictions of the effects of social distancing mandates within weeks of the near-national shutdown that March.
These innovative models are no longer considered to be outliers in critical computational public infrastructure; their quickly assembled mandates have now been codified with significant investment from their institutions, as well as from state and federal governments. In some cases, just as the research community demonstrated that datasets like phone mobility data could provide valuable information to public health officials seeking to mitigate disease spread, researchers now looking at responses to COVID are also discovering answers to age-old questions in computer science.
Team Science Writ (very) Large
The work of the UVA modelers proved so foundational to the state's response to the pandemic that the university expanded its reach through a $5-million investment in a formal contagion science program, composed of faculty members and students from multiple schools across the university.
"It started maybe partly as a result of the work we were doing on the pandemic response efforts, I think that's what motivated it," program co-director Madhav Marathe said, "but I think the key part there was the realization that pandemics are not just about disease transmission, but about multiple other transmissions. This particular pandemic is at least equal measures of social contagion, economic contagion, and many others."
Christopher Barrett, executive director of UVA's Biocomplexity Institute and co-director of the contagion science program with Marathe, said the program was meant to "catalyze" work around the sorts of big challenges something like the pandemic presents, with data pools vast and not even imagined until the past few years – "the kinds of things the institute is designed to do, which is to work outside of traditional academic disciplinary areas like math or computer science or this or that, but to work in derived areas from the problems themselves the world presents."
UVA researchers already are publishing work around these problems that tangentially emerged from the pervasive effects of the pandemic, and others are beginning to plan for such comprehensive efforts. For example, UVA economics professor Anton Korinek co-authored a paper in The BMJ outlining possible job-killing effects of the widespread advent of technologies such as artificial intelligence in telemedicine, which boomed in popularity as offices shut down.
At the University of Texas, too, Meyers sees the possibility for new constellations of multi-disciplinary research. Her group has received a $1-million pilot grant from the National Science Foundation's newly launched Predictive Intelligence for Pandemic Prevention (PIPP) program to explore how to expand the scope of the consortium's work. More than 40 multidisciplinary investigators from 11 institutions are collaborating to establish the university's new Center for Pandemic Decision Science, and during the next 18 months, the center will host five workshops and conduct five pilot projects, including a hackathon to forecast human health behaviors and a pathogen "wargame" exercise for Texas public agencies.
Meyers said the grant will allow her and her colleagues to "connect the dots," many of which made themselves very apparent during the course of the consortium's modeling work–and many of which had not been "mainstreamed" in modeling prior to the pandemic.
"A lot of things happened during COVID that the modeling community realized was a huge oversight in our models," she said. "We had data elements like age, or cities, but we didn't have something like the CDC social vulnerability index.
"Now it's used very widely and is shown to correlate very strongly with a risk of becoming infected, a risk of ending up in the hospital, risk of delay in getting access to vaccination and care, and risk of dying. When we look at Austin, we are a very segregated city, and sadly, it played out with very low vaccination rates where vulnerability is very high."
New data sources must be preserved
If the modeling community can reach consensus on anything surrounding the pandemic, it is that the avalanche of usable data sources was probably even bigger than the most optimistic data fusion expert could imagine–and that much of that data, which could be of immense use, is disappearing, to the community's chagrin.
One of the most salient lessons emerging from the pandemic, according to CHOP's Rubin, is that mask mandates were probably the most effective non-pharmaceutical intervention in slowing the spread of the disease. In a Health Affairs study, the CHOP team developed a methodology that compared like counties demographically and geographically with and without mandates.
In a paper soon to be published in Nature Scientific Data, UVA students undertook a painstaking recording of similar mandates across the entire state of Virginia. What they found in starting their research, Marathe said, was that the public record about interventions had already eroded, less than two years after the pandemic's beginnings.
"No state in the country had complete information on all the social interventions that have happened," Marathe said. "Things have just moved on. So we went painstakingly through Web sites, called churches sometimes, looked at hospitals and mayors' offices, and finally have reconstructed a timeline, county by county, of the social interventions that happened across Virginia."
Though some data has been lost irretrievably, Marathe said enough was preserved to be of real use for either archival purposes for historical research or utilitarian purposes in another public health crisis.
Rubin said he worries about the return of a "business as usual" ethos.
"People are finding it easy to retreat back to what they were doing before, and even with hospital reporting, there has been some erosion in the data," he said. "We need to take stock in the things that were welcome interventions. For example, telehealth really emerged, and that's what patients want, but some insurers are stalling on continuing reimbursement. I am afraid we will lose a lot of ground on areas that required rapid innovation around the pandemic, but sort of fall off the radar a bit. How prepared will we be when the next pandemic comes around, to just restart them up?"
Barrett and Marathe's colleague Bryan Lewis, a computational epidemiologist at the Biocomplexity Institute, said preserving the data detritus of the pandemic is crucial not just for disease modeling, but for the conceptual methodologies, including an ethos of historical preservation, around preserving big data.
"If you are going to learn something, it is based on experience," he said. "This is the experiential record itself. This is the trace left. As it stands now, it's a very extensive enterprise to go back and perform this natural history in this one area. One of the things Madhav's team has done is to put a bit of a rope around the problem of the natural history of the big data world. I think in the past, scientists thought of themselves as natural historians in part.
"And, whether or not this experience results in learning, whether it changes anything or not, is as importantly related to what happened and understanding that as it is in predicting whatever it is we think is going to happen next."
Symbiotic results
Just as public health officials came to rely upon the modeling community's expertise in sourcing and analyzing new types of data to help manage public policy through the pandemic, the discipline of computer science itself made some fundamental advances through serendipitous research into the disease.
University of Copenhagen researcher Joachim Kock made one of these discoveries; in trying to corral the data surrounding epidemiological progression of the disease, Kock stumbled upon a long-standing problem in computer science called the Petri net problem. By re-defining what a Petri net was, Kock was able to reconcile serial and graphical approaches to complex processes that had eluded computer science. His findings were published in the Journal of the ACM.
UVA's Barrett said the pandemic presented a host of similar opportunities in fundamental computer science research.
"We can work on down to very fundamental theoretical research by trying to work on these problems and running out of tools we know how to use," he said. "We take problems and we don't factor into them, 'Here's the computer science piece and here's the biology piece.' We say, 'This is the problem and we are supposed to calculate this.' That generates, very frequently, very commonly–it's like our whole business–very fundamental research questions. We have lots of theoretical scientists who work on these problems that are not obviously theoretical problems on their surface.
"But the reality is for us to be able to study it, it induces all kinds of research in basic knowledge we didn't know how to do. There are literally dozens of basic things that have been done over the course of the last three years that are really quite fundamental."
Gregory Goth is an Oakville, CT-based writer who specializes in science and technology.
Join the Discussion (0)
Become a Member or Sign In to Post a Comment