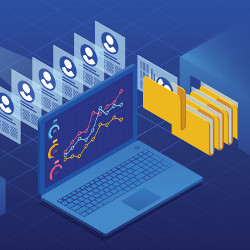
The way we apply for jobs has changed radically over the last 20 years, thanks to the arrival of sprawling online job-posting boards like LinkedIn, Indeed, and ZipRecruiter, and the use by hiring organizations of artificial intelligence (AI) algorithms to screen the tsunami of résumés that now gush forth from such sites into human resources (HR) departments. With video-based online job interviews now harnessing AI to analyze candidates’ use of language and their performance in gamified aptitude tests, recruitment is becoming a decidedly algorithmic affair.
Yet all is not well in HR’s brave new world.
After quizzing 8,000 job applicants and 2,250 hiring managers in the U.S., Germany, and Great Britain, researchers at Harvard Business School, working with the consultancy Accenture, discovered that many tens of millions of people are being barred from consideration for employment by résumé screening algorithms that throw out applicants who do not meet an unfeasibly large number of requirements, many of which are utterly irrelevant to the advertised job.
For instance, says Joe Fuller, the Harvard professor of management practice who led the algorithmic hiring research, nurses and graphic designers who need merely to use computers have been barred from progressing to job interviews for not having experience, or degrees, in computer programming. Retail workers schooled in inventory management have been excluded for not having experience (cleaning) shop floors. In addition, algorithms are rejecting highly qualified people with any kind of career gaps, even if they are for perfectly legitimate reasons, such as ill health, volunteering, or elder/child caregiving, and that is a factor that can fuel discrimination against candidates on gender, race, diversity, and disability grounds, too.
It is clear, Fuller says, that screening algorithms are not being programmed to separate the merely “nice to have” experience of applicants from the essential ones. Because this ruthless applicant filtering process conspires to conceal people from employers, the Harvard team refer to these digitally disregarded, would-be employees as “hidden workers.”
As Fuller and his colleagues say in their report Hidden Workers: Untapped Talent, “An enormous and growing group of people are unemployed or underemployed, eager to get a job or increase their working hours. However, they remain effectively ‘hidden’ from most businesses that would benefit from hiring them by the very processes those companies use to find talent.”
In the U.S. alone, the Harvard team estimates there are 27.4 million hidden workers, “with similar proportions of hidden workers across the U.K. and Germany.” With a record 10 million unfilled jobs in the U.S. alone in mid-2021, algorithmic hiring is simply wasting willing person power.
Many hiring companies are beginning to realize the algorithms are failing to deliver, Fuller says, as the flipside of having algorithms reject so many good people is that they often are employing the wrong people with out-of-date or obsolete skills. According to Fuller, only half of U.S. employers are satisfied with their hires, and only around a third of German employers.
Understanding the Problem
One of the major problems hiring firms need to address is that the providers of their AI technology—who are keen to develop algorithmic screening software in a bid to grab a slice of the global recruitment technology market that is expected to be worth $3.1 billion by 2025—are not questioning the software requirements enough when they are commissioned to develop résumé screeners.
“Tech companies are executing a vision, as expressed to them by their clientele, which is flawed,” says Fuller.
That vision? A quest for what he calls “hyperefficiency”: hiring firms are seeking AI that will winnow down the number of applicants to the most qualified few, in the shortest possible time, and at the least cost. However, Fuller believes companies seeking such a capability should be told by the AI engineering firms they work with that the consequences of writing such algorithms will necessarily exclude a lot of very strong candidates, who may only need a little extra training to excel in the roles the companies are seeking to fill.
It is not hard to see why hiring firms want to make résumé screening processes way more efficient, however. In 2010, an online job posting by a large U.S. company would garner about 120 résumés, but as job boards have grown, often amplifying each others’ posts, Harvard’s research suggests that number is now more like 250 applications.
Consider that the average number of people making it through to a first interview lies between four and six applicants, which shows just how hard hiring firms want to make their algorithmic assets sweat. “That shows you how they’re trying to create the very smartest of smart bombs—you know, ‘just give me Sir Galahad, or just give me Madame Curie’,” says Fuller.
The Soul of the Machine
How those résumé screening algorithms attempt to wield the knife is important if regulators are to “craft effective policy and oversight,” says Manish Raghavan, who was part of a Cornell University research team that presented a paper on mitigating bias in algorithmic hiring at the ACM FAT Conference on Fairness, Accountability and Transparency (now called ACM FAccT) in Barcelona, Spain, in January 2020.
“Transparency is crucial to further our understanding of these systems. While there are some exceptions, vendors in general are not particularly forthcoming about their practices. They treat their models as part of their IP (intellectual property), and so they don’t make them available to the public,” Raghavan says.
“But beyond this, vendors also have no obligation to release any data or studies on the validity of their assessments. Pressure from clients using their systems might get vendors to provide more information to those clients, but it’s not clear that this will lead to meaningful public transparency. Without increased legal pressure towards transparency, there’s a limit to how much information the public will get,” he adds.
He seems to have a point. One of the market leaders in algorithmic hiring, ZipRecruiter of Santa Monica, CA, declined to discuss how its screening algorithm works. Last September, when The Wall Street Journal asked another vendor, Oracle, to do likewise, it also declined to comment.
What is known is that 99% of Fortune 500 firms now use algorithmic screening, the Harvard team report.
By working backward from who gets excluded, however—and Harvard interviewed 125 hidden workers in Japan, France, Germany, Britain, and the U.S.—some aspects of how applicant screening algorithms work have been ascertained. “They do it on the basis, often, of ‘proxies’—something that causes us to infer something about the attributes of a candidate,” says Fuller.
“The classic proxy is requiring a university degree when the job does not require one. Another proxy, which is more perverse, is what’s called the ‘continuity of employment screen’. If someone has been out of work for more than six months, half the employers in the U.S. would automatically exclude that person from consideration,” he says.
Why? While hiring companies might justify this six-month cut-off by arguing that the “half-life” of workplace tech skills is shortening, with software versions ever-changing, markets moving, and new competitors arriving, people could be off their game after six months, but the actual reasons could differ markedly, says Fuller.
Employers might conclude the applicant was not supported by their network of industry colleagues, and wonder why they had been out of work for so long. Or, the applicant may have suffered from an illness, like depression, and now be over it, perhaps, or maybe had experienced a difficult pregnancy requiring complete bedrest, factors it would be illegal to bar someone from employment for, but which a six-month proxy cutoff handily takes care of, with no legal redress possible or provable.
Mission Impossible
Another problem relates to the bloated job descriptions programmed into algorithmic hiring that people simply cannot match up to: “They have become elephantine,” says Fuller, running to many pages. He cites the fact that some job ads for retail clerks now require an average of 30 skills, instead of focusing on the “four or five attributes that we know correlate with success.” The problem is that such listings are rarely if ever edited and, used again and again, are continually added to over time as job roles change, gathering unmeetable numbers of requirements.
Despite the slight gloom here, it is possible to boost your chances of getting past them and obtaining an interview. The trick is to use precisely the language of the job posting in your résumé and cover letter, says Fuller, even if it is not the way you normally describe your work. “You should also look at the LinkedIn pages of people who got jobs there, to see how they’re describing their skills.”
“Hacking the system” in that way is becoming the order of the day for those who actually get past algorithmic screeners for an online video interview, as people are now posting ‘how to beat the robot interviewer’ guides online. In these, job applicants are exhorted to be confident, smile, use positive body language, and make eye contact with their virtual interlocutor.
They are largely wasting their time, however, at least with video interview site HireVue. Says Lindsey Zuloaga, HireVue’s chief data scientist, “HireVue will never evaluate a candidate’s personal appearance, eye contact, what they are wearing, or the background where they are taking the interview. Those attributes are not relevant to skills and competencies for a job, and there is no visual analysis used in any of HireVue’s technology.”
Where HireVue does apply machine learning, however, is in the natural language processing it applies to the interviewee’s speech—to analyze their word choice, their use of language, and to check what they say against what Zuloaga calls “a set of job-relevant competencies that research shows indicate success in a role.” HireVue also avoids any kind of emotion detection, she says, as the company does not believe it works or is at all relevant, and is phasing out an earlier system that attempted to measure the perceived “tone” of someone’s voice, too.
As it works downstream of algorithmic hiring screeners, HireVue has a ringside seat to observe what works and what does not. “We agree that résumé screening is broken, and that the traditional résumé is not a valid tool to assess and screen candidates,” says Zuloaga. “Nearly everyone who has applied for a job has seen how broken the process is, and we are working hard to change that.”
Many blue chip companies are realizing this now, too, and some already are acting to deconstruct the worst pillars of algorithmic hiring, including Amazon, General Motors, Ikea, LinkedIn-owner Microsoft, Google, Slack, and McDonald’s. All have established programs designed to seek out the hidden workers Fuller’s team identified.
While Fuller believes the blame for algorithmic hiring’s failures lies squarely with the specification, not the code—because “code just does what it’s asked to,” he says—there is always the nagging doubt that it might not. The reason? Deep learning neural networks can be “brittle” —that is, they can make unexpected, unexplainable statistical associations that could be unethical, if not even legally actionable.
This risk was highlighted at FAT 20 by Raghavan and his Cornell colleagues. Machine learning, they said, may uncover “relationships that we do not understand” and produce “ethically problematic correlations” about an applicant.
There is a strong precedent for this concern. In late 2018, a client of Minneapolis, MN, law firm Nilan Johnson Lewis was vetting a machine-learning-based résumé screening tool when they realized it had hit upon the perfect fitness factors for a stellar job candidate: the best people to hire, it decided, were people called Jared who played lacrosse.
Fuller believes the blame for algorithmic hiring’s failures lies squarely with the specification, not the code—because “code just does what it’s asked to,” he said.
“Maybe lacrosse is positively correlated with success—perhaps because applicants who played lacrosse have developed some teamwork skills—but it can also be correlated with attributes like gender and race,” says Raghavan.
“Machine learning is designed to uncover these kinds of correlations, but without human oversight, it has no way to know whether ‘lacrosse’ is ethically problematic.” Putting such human oversight in place is what is desperately needed to make algorithmic hiring work more fairly: HR directors would do well to remember what the H stands for in their departmental acronym.
Fuller, J. B., Raman, M., Sage-Gavin, E., Hines, K., et al.
Hidden Workers: Untapped Talent Harvard Business School’s Project on Managing the Future of Work, and Accenture, September 2021 https://hbs.me/3CDxoUw
Mirowska, A. and Mesnet, L.
Preferring the devil you know: Potential applicant reactions to artificial intelligence evaluation of interviews Human Resource Management Journal, June 2021 https://doi.org/10.1111/1748-8583.12393
Raghavan. M., Barocas, S., Kleinberg, J., and Levy, K.
Mitigating Bias in Algorithmic Hiring: Evaluating Claims and Practices ACM Conference on Fairness, Accountability and Transparency, January 2020 https://doi.org/10.1145/3351095.3372828
Gershgorn, D.
Companies are on the hook if their hiring algorithms are biased Quartz, October 22, 2018 https://bit.ly/2Y9y0lL
McKeever, V.
How to ace a job interview with a robot recruiter, CNBC, April 13, 2021 https://cnb.cx/2ZViBpT
Join the Discussion (0)
Become a Member or Sign In to Post a Comment