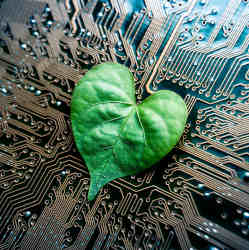
As artificial intelligence (AI) becomes increasingly pervasive and learning models more complex, researchers are thinking about the impact of their work, including the environmental costs. Discussions on green topics—from carbon costs to efficiency improvements—are evolving within the community.
We asked researchers in the fields of computer science and machine learning for their insights.
Growth is exponential, a new approach is required.
"AI is everywhere," said Roy Schwartz, a professor of natural language processing at The Hebrew University of Jerusalem, Israel. "We're growing at an exponential pace which is something which is quite unprecedented in any technological field." For Schwartz, the environmental and societal impacts of this growth are unsustainable in AI's current form.
The speed of progress goes hand-in-hand with leaps in the computational, energy, and financial costs of AI. In a 2018 blog, OpenAI analyzed the amount of compute—measured in petaflop/s-days, where a petaflop/s-day (pfs-day) consists of performing 1015 neural net operations per second every second for one entire day—used in the largest AI training runs, from AlexNet, a convolutional neural network designed in 2012, to DeepMind's 2017 AlphaGo Zero.
The bloggers—Dario Amodei, CEO of AI safety and research company Anthropic and OpenAI research scientist Danny Hernandez wrote the original post, while OpenAI's Girish Sastry, Greg Brockman, and Ilya Sutskever, and Anthropic co-founder Jack Clark contributed to its addendum— wrote that since 2012, "The amount of compute used in the largest AI training runs has been increasing exponentially with a 3.4-month doubling time." They pointed out that Moore's Law— an observation on the growth of computing speed and capability—had a two-year doubling period.
Schwartz argues it is time for the AI community to take a harder look at the impact of its work. A 2020 contributed article in Communications that he co-authored with postdoctoral researcher Jesse Dodge of Carnegie Mellon University, Amazon Professor of Machine Learning Noah A. Smith, and Allen Institute for AI CEO Oren Etzioni, advocates for so-called Green, as opposed to Red, AI.
"When we talk about Red AI, it's the practice of trying to get a better result by increasing the model capacity, and Green AI's kind of the alternative, of trying to take other things into consideration," said Schwartz.
According to Schwartz, Red AI has made a "tremendous contribution" to where AI is today, however, it encourages state-of-the-art results, regardless of the cost. Under a Green AI approach, the "other things" that should be considered include making the technology more environmentally friendly and inclusive—despite spiraling costs that, Schwartz pointed out, constitute a barrier to who can participate in AI research.
Schwartz and his co-authors suggest a two-pronged approach to Green AI. On the one hand, they are calling on researchers to do a better job at quantifying and reporting the environmental and computational costs of their scientific results; on the other, they are asking them to develop models that are more efficient. "Try to quantify, try to estimate more accurately how much energy, electricity, computation is being used throughout these operations and obviously try to promote more efficient solutions," said Schwartz.
The challenge right now is how to count these costs. Calculations depend on many factors, like the number, speed, and power of processors; the efficiency of datacenters, and the amount and type of energy used.
Carbon emissions are particularly hard to pin down, said Schwartz. "It depends so much on where you run your experiments, whether you're running it in a region that uses coal or nuclear power or hydroelectricity, and so on."
One solution that could allow efficiency comparisons between geographical locations is reporting the total number of floating-point operations (FPOs)— a measure of computer performance— required to generate a result, said Schwartz. He acknowledged it is not a perfect measure: "We're compromising some of the aspects that other measures capture because we think this is the most important thing at the moment, to be able to compare different models and in different locations."
For Schwartz, transparency is key to future endeavors. As a sign of progress, he points to a recent initiative by researchers at Google and the University of California, Berkeley (UC Berkeley), who quantified the amount of carbon released by the training of some of the largest Natural Language Processing (NLP) models in recent years.
Training is not AI's biggest environmental threat.
David Patterson is a computer scientist at UC Berkeley, a distinguished software engineer at Google, and a Turing Award recipient. He led the group of researchers—Quoc Le, Chen Liang, Lluis-Miquel Munguia, David So, Maud Texier, and Jeff Dean at Google, and Joseph Gonzalez and Daniel Rothchild at UC Berkeley— that carried out the research highlighted by Schwartz.
Patterson got involved in the project due to increasing concerns—raised within the AI community and the press—about the carbon footprint of very large NLP models. The group undertook the work to try to "figure out what the facts are about it," he said.
The researchers calculated the energy use and carbon footprint of five large NLP models: T5, Meena, GShard, Switch Transformer, and GPT-3. The results for each model are described in detail in the resulting paper. Training Meena, a multi-turn open-domain chatbot, for example, used 232 MWh of electricity, resulting in emissions into the atmosphere of 96 tonnes (metric tons) of carbon dioxide equivalent (tCO2e); training T5, a pre-trained language model, used 86 MWh and produced 47 tCO2e.
Patterson said the research allowed the carbon footprint of training to be put into perspective. "Google publishes how much energy it uses every year, and so we calculated the cost of the training in terms of energy consumption for these large models, as it says in the paper. It's a very small fraction of what we do."
Based on the results, he argued that training is not as costly to the environment as other activities undertaken by the AI community, as "People travelling to conferences have much larger carbon footprints." Patterson said he recently turned down an invitation to give an in-person keynote address in Shanghai due to the carbon cost of travel, opting for a remote format instead. He encouraged the AI community to adopt virtual conferences as good green practice.
Still, he said, there is a lot that can be done to improve the energy efficiency of training. "With the cloud you can train anywhere, literally anywhere in the world. You should pick datacenters that have the best carbon footprint."
"Remarkably," the researchers suggest in their paper that "the choice of DNN, datacenter, and processor can reduce the carbon footprint up to ~100-1,000X." Like Schwartz, they call for transparency, suggesting energy usage and CO2e should be key metrics used to evaluate the training requirements of AI models.
Their recommendations include deploying large but sparsely activated deep neural networks (DNNs) that consume less energy than dense DNNs, optimizing where and when models are trained, and working at datacenters with the smallest carbon footprint.
Patterson's hope is for a "virtuous circle" in which the carbon footprint of models is reported in papers, efficacy incentivized, and good practice rewarded.
The current situation is unsustainable, the future doesn't have to be.
In a February 2020 blog post, Ameet Talwalkar, an assistant professor in the Machine Learning Department at Carnegie Mellon University, argued that AI must get greener and outlined several strategies for doing so, including increasing hardware performance and encouraging partnerships.
Talwalkar wrote the post just before Covid hit. Reflecting now, over 20 months later, he said the pandemic has exacerbated the trends. "People lean more into technology, and leaning more into technology these days means leaning more into AI and so, if anything, I think the pandemic has further accelerated what was already a very fast, quickly accelerating industry."
Models are getting bigger and bigger, Talwakar said, and "They're really inefficient from an energy point of view. They're not very environmentally friendly." However, he stressed it is vital to remember that AI is still in its early stages. "We're throwing whatever resources we can at seeing how we can push things forward."
Talwalkar draws a parallel between the Human Genome Project (HGP), estimated to have cost $2.7 billion over 13 years, and Red AI. "It was incredibly expensive, horribly inefficient, clearly not a reproducible thing that we can sequence one person's genome for that long and that much money, but it showed us that it was possible, and it paved the way."
Talwalkar points out that leading-edge technologies are not usually built on the most efficient processes. He expects the efficiency of training AI models will improve. "In datacenters, we've seen algorithms get way more efficient; I think that we know how to solve those problems."
However, he said that AI is still "fairly black box," in that we do not yet fully understand it, and experimentation is taking place out in the real world, not only in the lab. This raises other concerns around issues like fairness and privacy. "That experimentation leads to Red AI, but it also leads to these societal issues," said Talwalkar.
Perspectives on AI and sustainability vary. However, as the community thinks more deeply about the impact of its work on the planet and society, greater transparency, accountability, and improved efficiency are emerging as shared goals.
Karen Emslie is a location-independent freelance journalist and essayist.
Join the Discussion (0)
Become a Member or Sign In to Post a Comment