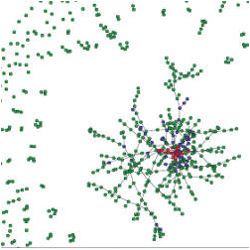
Step inside Brandon Marshall’s lab at Brown University and you get a glimpse into the unfolding future of epidemiology. Marshall, along with a multidisciplinary team comprised of IT specialists, software developers, mathematicians, healthcare analysts and others, are melding their expertise to take HIV policymaking to a new level. In a world filled with what-if questions, the assistant professor of epidemiology is supplying answers by building a model that in many respects resembles a consumer-oriented simulation game such as The Sims.
For example, Marshall can choose to view a fictional person based on particular characteristics. Overall, approximately 150,000 life courses reside within the model, which is focused on New York City. By selecting one agent and observing the interactions with other agents—including how they approach sex and drugs and how officials and policymakers approach issues such as the distribution of condoms and sterile needles, it is possible to understand behavior and choices in a far more complete and realistic way. “We can track individuals over time, which is really novel and exciting,” he says.
Marshall is one of a growing number of researchers turning to computer modeling to go where researchers have not gone before. For most of human history, understanding the impact of a disease or health problems was nothing less than guesswork. When a new virus or bacterial infection popped up, public officials did their best to assemble data, extrapolate on it and establish a course of action. “The problem,” observes Marshall, “is that healthcare and public policy experts have had limited knowledge and visibility into all the factors and variables.”
However, by plugging into massive datasets and tapping into today’s computing power—the list includes mobile tools, social media, and crowd-sourcing to track movements and behavior in real time—it is possible to create far more realistic forecasts and what-if scenarios. The impact of computer modeling on decision-making and public policy is nothing less than revolutionary. Ruben Juanes, an associate professor at MIT, also turned to algorithms and advance models “to understand extremely complex issues in ways that weren’t possible in the past.”
By the Numbers
The idea of using computers to analyze health data is nothing new, of course. In the 1950s, researchers—including epidemiological and public health officials—began exploring ways to transform data into actionable models. Unfortunately, a general lack of computing power and far less sophisticated software imposed severe limits on the breadth and depth of analysis that could take place.
“Traditionally, researchers have relied on a reductionist approach to problem solving,” points out Patricia Mabry, senior advisor in the Office of Behavioral and Social Sciences Research at the National Institutes of Health (NIH). However, this approach—known as a randomized controlled trial—typically addresses only one small aspect of the overall problem. Reductionism essentially attempts to condense and simplify issues in order to make the process more manageable. Controlled studies focus on different groups and different assigned or measured variables. Both of these techniques rely on a relatively narrow focus.
It was not until the 1990s that the first robust computer models began to appear. The addition of the Internet and big data analytics has revolutionized computer modeling over the last decade. Today, many new data points exist, including databases and metrics gleaned from mobile geolocation data and social media. In the healthcare arena, researchers now use computer modeling to examine the effect of different HIV policies, emergency response scenarios surrounding a poison gas attack, how eating and exercise impact obesity and healthcare costs, and more.
Comprehensive simulation modeling is a key to effective decision-making, particularly in today’s cost-conscious environment, states Peter Aperin, M.D., vice president of medicine for Archimedes Inc., a San Francisco, CA, company that develops full-scale simulation models of human physiology, diseases, behaviors, interventions, and healthcare practices for the likes of Kaiser Permanente and the Robert Woods Foundation.
Aperin says these techniques are useful for examining a wide range of issues: everything from obesity and diabetes to heart disease treatment options. “What if we change treatments? What if we use different interventions? What if different interventions or treatments have different side effects or cause different behavioral changes? We are able to view how an almost infinite number of decisions affect downstream health outcomes and costs.” In fact, it is possible to visualize these complex datasets rather than poring over an endless stream of statistics and numbers.
Moreover, researchers can select specific groups—men, women, gays, those who take certain medications, or display high or low compliance rates and much more—and watch these simulations play out as agents interact with others and change their behavior over time. These virtual people influence each other, just as they do in the real world. It is also possible to toss new factors or variables into the equation, such as a virus that mutates or a new pharmaceutical drug, and watch events unwind in a completely different way. After researchers run a simulation a number of different ways they begin to view patterns and trends that provide valuable clues about public policy strategies and outcomes.
Marshall’s HIV research is a perfect example. “The modeling allows us to combine data in very interesting ways that would be almost impossible empirically,” Marshall explains. “We can combine datasets based on things like gender, sexual orientation, drug use, drug treatments and associated behavioral outcomes, access to drug abuse treatment programs and more. The model lets us see how decisions support one another or how an approach or program could prove detrimental.” Marshall’s model is designed to examine different approaches and policy decisions over a 20-year span.
Comprehensive simulation modeling is a key to effective decision-making in today’s cost-conscious environment.
Consider “Agent 89,425,” who is male and has sex with men. He participates in needle exchanges, but according to the probabilities built into the model, in year three he begins to share needles with another drug user with whom he is also having unprotected sex. In the last of those encounters, Agent 89,425 becomes infected with HIV. In year four he begins participating in drug treatment and in year five he gets tested for HIV, starts antiretroviral treatment, and reduces the frequency with which he has unprotected sex. Because he takes his HIV medication without exception, he never transmits the virus further.
The research has already yielded some remarkable insights. For example, Marshall projects that with no change in New York City’s current programs, the infection rate among injection drug users will be 2.1 per 1,000 in 2040. Expanding HIV testing by 50% would drop the rate only 12% to 1.9 per 1,000; increasing enrollment in drug treatment programs by 50% would reduce the rate 26% to 1.6 per 1,000; providing earlier delivery of antiretroviral therapy and better adherence would drop the rate 45% to 1.2 per 1,000; and expanding needle exchange programs by 50% would reduce the rate 34% to 1.4 per 1,000. However, adopting all four tactics would cut the rate by more than 60%, to 0.8 per 1,000.
These types of analyses/dependences are routine in large data analysis in other fields. Is this true in healthcare or are the actors just learning this now? Or is Marshall’s model something new and better than what already exists?
Putting Models to Work
The appeal of advanced computing modeling—referred to by Mabry and her NIH colleagues as systems science methodologies—is that it allows researchers far greater latitude to address the complexity of real-world phenomenon and “and to investigate what-if scenarios that cannot be studied in the real world due to time, money, ethical, or other constraints,” Mabry says.
This is no small matter. Complexities of the real world include making sense out of bidirectional relationships where one variable affects another and vice versa. Because changes in the variables feed off one another, such relationships have the potential to, as Mabry puts it, “generate vicious cycles in which we observe things deteriorating rapidly or virtuous cycles in which we observe a situation improving rapidly.”
Because the underlying cause of such situations is not always apparent to the naked eye, policymakers may actually make bad situations worse by applying a remedy to the wrong place in the system. “The goal of using systems science methods is to understand how the various components that make up a system interact and affect each other to produce an outcome. These methods excel at identifying nonlinear relationships, and time-delayed effects as well as inter-dependencies,” Mabry explains.
At MIT, researchers in the Department of Civil and Environmental Engineering, led by Ruben Juanes, are applying seemingly incongruous methods to understand contagion dynamics through the air transportation network. Presently, the team is attempting to understand how likely the 40 largest U.S. airports are to influence the spread of a contagious disease originating in their home cities. The project could help determine how fast a virus might spread and appropriate measures for containing the infection—from quarantining individuals to closing airports—in specific geographic areas. This information could also aid public health officials in making decisions about the distribution of vaccinations or treatments in the earliest days of contagion.
In order to predict how fast a contagion might spread, researchers are examining variations in travel patterns among individuals, the geographic locations of airports, the disparity in interactions among airports, and waiting times at individual airports. Juanes, a geoscientist, has tapped past research on the flow of fluids through fracture networks in subsurface rock to build an algorithm for the current task. Moreover, the team plugs in cellphone usage data to understand real-world human mobility patterns. The end result is “a model that’s very different from a typical diffusion model,” he says. It is plugging in more data—and better data—to create a more robust model than has ever before existed.
Archimedes’ Peter Alperin says that today’s models are aiding and speeding policy decisions in ways that were unimaginable only a few years ago. The NIH, government agencies, pharmaceutical firms, and healthcare organizations use these models to help build more effective policies or develop treatment strategies or new medicines. Last year, the firm began building models for the U.S. Food and Drug Administration (FDA) to better understand clinical trials evaluating weight loss medications. The data is being used to better understand the benefits of weight loss against the long-term risks of cardiovascular outcomes in patients treated with weight loss drugs.
Nevertheless, computer modeling is not a fix-all, says Sandro Galea, chair of the Department of Epidemiology at Columbia University. Among other things, he has examined how policy decisions affect social problems ranging from obesity to how large-scale disasters and trauma affect mental health among various demographic groups. In the latter scenario, for example, modeling helps identify who is at greater risk and what types of treatment and services can help reduce mental illness.
However, all models are built on assumptions and have some flaws and errors. Indeed, there is no standard for how to build an effective computer model or to establish confidence in what a model produces. Mabry and her partners at NIH—a major provider of research grants—are now focusing on model “verification, validation, and uncertainty quantification.” They hope to collectively produce some guidance for model builders, as well as those reviewing journal manuscripts and grant applications. Without this, the fledgling field will continue to produce models that range widely in quality, she says.
Yet, computer modeling continues to evolve and gain acceptance. “Computer modeling isn’t a crystal ball,” Mabry concludes. “But it is helping to illuminate the complexity of health and social problems—along with potential remedies. Success is ultimately dependent on culling huge amounts of data about the population, developing good algorithms, and harnessing the success of supercomputers to make sense of complex relationships. This information can then be used by public policymakers to do their job.”
Further Reading
Auchincloss, A.H., Gebreab, S.Y., Mair, C. and Diez Roux, A. V.
A review of spatial methods in epidemiology, 20002010, Annual Review of Public Health 33, 107122; http://www.annualreviews.org/doi/abs/10.1146/annurev-publhealth-031811-124655
Marshall B.D.L., Paczkowski, M.M., Seemann, L., Temalski, B., Pouget, E.R., Galea, S. and Friedman, S.R.
A complex systems approach to evaluate HIV prevention in metropolitan areas: Preliminary implications for combination intervention strategies, PLOS, 2012; http://www.plosone.org/article/info%3Adoi%2F10.1371%2Fjournal.pone.0044833
Meyer, J., Ostrzinski, S., Fredrich, D., Havemann, C., Krafcyk, J. and Hoffmann, W.
Efficient data management in a large-scale epidemiology research project, Computer Methods and Programs in Biomedicine 107, 3, Elsevier North-Holland, NY, 2012.
Mysore, V., Narzisi, G. and Mishra, B.
Emergency response planning for a potential Sarin Gas Attack in Manhattan using agent-based models, agent technology for disaster management, Hakodate, Japan, 2006; http://www.cs.nyu.edu/mishra/PUBLICATIONS/06.sarin.pdf
Sobolev, B., Sanchez, V. and Kuramoto, L. Health Care Evaluation Using Computer Simulation, Springer, 2012.
Join the Discussion (0)
Become a Member or Sign In to Post a Comment