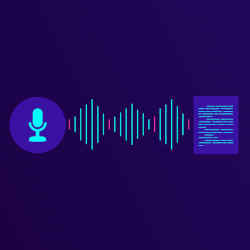
ChatGPT and generative artificial intelligence (AI) may be having a moment, but don't underestimate the value of speech-to-text transcription, sometimes referred to as automatic speech recognition (ASR) software, which continues to improve.
ASR technology converts human speech into text using machine learning and AI. There are two types: synchronous transcription, which is typically used in chatbots, and asynchronous, where transcription occurs after the fact to capture customer/agent conversations, notes Cobus Greyling, chief evangelist at HumanFirst, which makes a productivity suite for natural language data.
ASR made some waves in recent months with the announcement of Whisper from OpenAI, the organization that created ChatGPT. Whisper was trained on 680,000 hours of multilingual and supervised data collected from the Web. OpenAI claims that large and diverse dataset has improved the accuracy of the text it produces; the company says Whisper also can transcribe text from speech in multiple languages.
"What that means is that it's extremely accurate—right off the top—without much tuning or training,'' says Christina McAllister, a senior analyst at research and advisory company Forrester Research. "The large language model aspect, which is based on huge amounts of data, is what's new and is the most innovative aspect of the ASR market today,'' she says.
Because of its ability to transcribe meetings and interviews more efficiently and accurately, one of the broadest enterprise use cases for speech-to-text is in customer call centers. The next phase in the development of ASR is to use artificial intelligence to analyze call center conversations for customer sentiment and to validate compliance in regulated industries, according to Annette Jump, a vice president analyst at Gartner.
The benefits of ASR in the call center context are its ability to identify customer problems early and to improve customer satisfaction by resolving issues sooner, says Jump.
Other use cases include generating closed captions for movies, television, video games, and other forms of media. ASR is widely used in healthcare by physicians to convert dictated clinical notes into electronic medical records.
Speech vendors typically leverage a third-party ASR engine so they don't have to build their own, McAllister says. That frees them up so they can "do all the rest of their magic from the transcript point forward,'' she says.
Some of the new AI capabilities for speech-to-text systems include taking actions based on a transcript, prompting someone when it's appropriate to ask a follow-up question, and summarizing a conversation at the end of a call, McAllister says.
One frequently used AI-powered speech-to-text transcription service is Otter.ai, which has added capabilities aimed at improving meetings, including integration with collaboration tools such as Zoom and Microsoft Outlook.
In February, Otter.ai announced OtterPilot, an AI-powered meeting assistant that automatically transcribes and summarizes key meeting takeaways while capturing slides and eliminating the need to take manual notes during a meeting. OtterPilot extends the functionality of Otter's AI assistant capabilities launched in 2022.
The AI-powered voice speech-to-text services market is growing more competitive. Microsoft recently announced that OpenAI capabilities have been integrated into Microsoft Teams Premium, including the automatic generation of meeting notes.
"Speech-to-text software is evolving all the time, with machine and deep learning-powered features like natural language processing (NLP) and real-time transcription improving the accuracy and accessibility of solutions,'' says Logan Spears, chief technology officer and co-founder of Plainsight, a computer vision platform provider.
Embedded computer vision systems are enabling speech-to-text systems to improve the transcriptions they produce by studying mouth movements and identifying speakers, while offering additional contextual insights based on factors like body language and facial expression, Spears adds.
Speech-to-text and ASR are more or less synonymous terms, as they are the engine that transfers the audio to a transcript, and are used by any technology that relies on understanding human speech, McAllister says. Conversation intelligence and speech analytics software require an ASR to transform audio into text; they then perform an analysis of the text once it has been converted, she notes. This gives offerings in which they are incorporated the ability to apply speech analytics after the fact to a transcript, to understand what someone said.
Eventually, these types of software might enable vendors to take a transcript and offer features like coordinating a meeting and sending an email out to invite participants. This requires NLP to determine the meaning of the words in a transcript, McAllister says.
"One of the most innovative uses that we're seeing is companies creating transcripts of Zoom calls and other virtual meetings,'' observed speech-to-text transcription service Rev in a 2022 blog.
There are some challenges with using ASR systems; most notably, accuracy. Translation and analysis in multiple languages are others issues because ASR systems do not have equivalent accuracy in all languages, McAllister says.
"It can be challenging to use the same models to apply to different languages because our language structures are so different,'' she explains.
Another consideration is that when pulling from a speech-to-text transcript, "You're kind of flattening the narrative a bit,'' McAllister notes, pointing to the fact that the system won't necessarily catch sarcasm or intonation.
Enhancements in the speech-to-text space are "coming fast and furious,'' says Rebecca Wettemann, principal at tech research firm Valoir. "The good news is ChatGPT is raising awareness of how far these tools have come, and the investments that Microsoft and others are making in embedding [AI] capabilities into their products … will drive a lot of adoption." The challenge for products like Otter and Dragon as these capabilities become part of broader applications will be differentiating what they provide as standalone products, Wettemann says.
Esther Shein is a freelance technology and business writer based in the Boston area.
Join the Discussion (0)
Become a Member or Sign In to Post a Comment