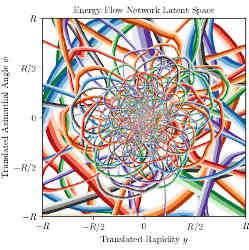
If a computer can teach itself to play the age-old board game Go better than the human world champion, if a computer can even conjure up a genius new Go move, couldn't a computer also discover new physics?
Jesse Thaler, an associate professor of physics at the Massachusetts Institute of Technology (MIT), investigates the potential of artificial intelligence (AI) in particle physics. In 2020, Thaler also became the director of the National Science Foundation's AI Institute for Artificial Intelligence and Fundamental Interactions (IAIFI), which is dedicated to advancing physics knowledge and galvanizing AI research innovation.
At the moment, the Standard Model of particle physics is the best description of three of the four fundamental forces of nature, and of a large family of elementary particles. Finding deviations from the Standard Model might lead physicists to discover new particles or new interactions, and AI might be able to play an important role in this.
In a Zoom interview, Thaler talks about the present and the future of applying AI to particle physics.
Why does particle physics need AI?
Particle physics is a data-rich field. In CERN's Large Hadron Collider (LHC), particles collide every 25 nanoseconds. Processing and analyzing these collisions has to be done in an automated and robust way. We are seeing now that our traditional tools have reached their limits. AI has the potential to exploit new features of these datasets."
Where are we right now with AI in particle physics?
Right now, AI has augmented and improved on strategies that particle physics has already used for many decades. AI gives physicists a better ability to reconstruct particles from the collision debris and interpret the results.
What type of AI is used in particle physics?
It's almost all machine learning, and the biggest distinction to make is between supervised and unsupervised machine learning. Thus far in particle physics, we have focused on supervised learning, based on training sets with labeled data.
How open are physicists to applying AI?
Some years ago, a number of my colleagues, and I as well, were quite skeptical about applying deep learning to particle physics. In the past, we had faced challenges with the robustness of machine learning. Looking back, this was due to limited computational power and a limited amount of data. This has all changed. We are now using data in more sophisticated ways and we have the computational power to leverage advanced architectures. But especially important is that we have learned how to bake physical knowledge into the AI that allows us to make predictions in a more robust way.
Can you give an example of physical knowledge that is used in the AI?
Take as an example a picture of a cat. If the cat is moved to a different part of the image, it is still the same cat. A physicist calls the move a translation, and you want that image recognition system to be invariant under that translation. However, generic AI techniques of the past didn't know about that translational structure. Convolutional neural networks, which are baked into the present-day deep learning technologies, automatically incorporate this translational symmetry. This has led to much more robust answers. And there are more examples of baking physics principles into your AI architecture.
What is the goal of the new institute of which you are the director: the NSF AI Institute for Artificial Intelligence and Fundamental Interactions?
Our goal is to advance physics knowledge, from the smallest building blocks of nature to the largest structures in the universe. At the same time, we want to galvanize innovation in AI research on a foundational level. In our institute, about one-third of the people work in theoretical physics, one-third in experimental physics, and one-third in foundational AI. It is very important to develop a common language that allows these three communities to communicate better. We have seen all kind of surprising connections between fundamental physics and AI techniques. We expect that we can use various physics principles, like symmetries, to drive AI forward.
What are your expectations for AI in particle physics over the next 10 years?
The trend that will absolutely continue is using AI as a refinement of existing techniques. That is a no-brainer. Secondly, we will see a transition in the types of analyses that we are going to do. Instead of saying: 'does this data set come from the Standard Model versus from a specific hypothesis?', we will be able to ask the question: 'to what extent is this data consistent with the Standard Model versus any hypothesis?' This requires the field to wrestle with the question: 'what does it mean to search for all possible deviations from what is already known?' That is a hard question to answer, but there is evidence that unsupervised machine learning can be very powerful tool."
Will the next Einstein be a computer?
I don't know whether the next Einstein will be a computer, but I know that developing a common language between humans and computers, and figuring out how the concepts we deal with in theoretical physics can be expressed in the form of a computer program, that is going to be very powerful. For the next generation of physicists, a new type of training is required: learning to use computational resources as part of the creative process.
Bennie Mols is a science and technology writer based in Amsterdam, the Netherlands.
Join the Discussion (0)
Become a Member or Sign In to Post a Comment