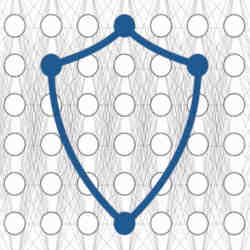
Artificial intelligence (AI) has made great strides in catching attempted credit-card fraud—most of us have received communications from our credit-card issuers to confirm attempted purchases made by cybercriminals. Using machine learning (ML) to compile "synthetic identities" that display the usual behavior patterns of its credit holders, financial institutions can spot anomalous behaviors in real time. Unfortunately, cybercriminals likewise are using AI to create their own synthetic identities, producing results realistic enough to fool the AI that spots anomalous behaviors.
This battle of the AIs—pitting fraudster again cybersecurity—is also being fought in the trenches of fake news, fake videos, and fake audio. Thus the arms race has begun: AI versus AI.
Jupiter Research's Steffen Sorrell says synthetic identities are the "low-hanging fruit" of credit card fraud. According to Jupiter Research's latest Online Payment Fraud report, synthetic identities are driving online payment fraud toward $200 billion in losses to the bad guys by 2024. For the good guys, it is also driving the fraud-detection market to reach $10 billion over the same period, up from $8.5 billion this year.
"Online fraud takes place in a highly-developed ecosystem with division of labor," said Josh Johnston, director of AI Science at Boise, ID-based fraud prevention enterprise Kount Inc. Johnston said cybercriminals specialize in different types of crimes ranging from manually "skimming cards" to creating synthetic identities with AI. "Others test stolen card numbers and credentials against soft targets like charities and digital goods merchants to make sure they haven't been cancelled," said Johnston, who claims high-limit credit card numbers with accurate name, address, and CVV (card verification value) can be purchased for less than a dollar in Internet black markets on the dark web.
"A fraudster can buy a list of these verified cards and monetize them through any number of online schemes," said Johnston. "AI is used heavily by these criminals, who also share software tools and tips on Internet forums just like legitimate developers."
These high-volume fakes use all types of AI and other automation techniques, ranging from small programs that generate and register realistic email addresses by combining real first and last names followed by random numbers, to large ML programs that create synthetic identities by combining bits of information from multiple real people to create a composite, according to Johnston. If a fraud detector checks on a synthetic identity, they often find a fake email account, Facebook page, and other Internet presences showing details of the synthetic identity have been recorded by the fraudster.
Thus the fraud-detection skills of cybersecurity programmers are pitted against the fraud-creation skills of the Black Hats.
These fraud-creation skills are not just used in credit-card scams, but extend into the fields of image and speech recognition, where the tools are being used in reverse to create fake news, fake videos, and fake audio. In fact, money transfer fraud, in which fake audio is used, is growing faster than online payment fraud, according to Nick Maynard at Juniper Research, who said losses in this arena are predicted to grow by 130% through 2024.
"Machine learning," said Maynard, "is increasingly essential to constrain fraud."
Deep-fake fraud is a game of whack-a-mole as each AI—bad and good—temporarily gains the upper hand. "It's a cat and mouse game," according to Johnston, who measures success and failure by a single variable that he calls friction, that slows down one side or the other until a new form of 'lubrication' can put one side ahead of the other.
"Fraudsters respond to friction, just like legitimate users on the Internet. When we get the upper hand, causing fraudsters too much friction, they move on to a softer target not protected by fraud detectors. A good fraud solution increases friction for the bad guys and reduces friction for good customers. Advances on one side, however, cause a shift in strategies on the other side," said Johnston.
When the Internet was new, there wasn't much worth stealing online, according to Johnston, so fraudsters mostly tested credit cards online, then monetized them by buying goods in person. Today, online commerce is as convenient for the bad guys as it is for the rest of us, and the widespread adoption of credit cards with security chips inside them makes in-person transactions a hazard for fraudsters, with the result of moving fraud activity increasingly online. As a consequence, more detailed analysis is being used by fraud-detection AIs, according to Johnston.
"Without giving away the farm, our successful fraud prevention methods in 2020 rely on spanning large data sets to look for patterns inherent to fraud," said Johnston. "A bad guy can steal all your secrets, but they can't mimic your tastes, behavior, and history. At the end of the day, fraudsters have to pull a scam to get their hands on something of value, and with the right data, we can tell the difference between a scam and a good customer."
Fake News/Video/Audio
AI already has been used to automatically create fake news stories. For instance, OpenAI's prototype GPT-2 text generation system uses machine learning to translate text, answer questions, and write fake news. When the sentence "Russia has declared war on the United States after Donald Trump accidentally …" was fed into GPT-2, it created this fake news story:
Russia has declared war on the United States after Donald Trump accidentally fired a missile in the air. Russia said it had "identified the missile's trajectory and will take necessary measures to ensure the security of the Russian population and the country's strategic nuclear forces." The White House said it was "extremely concerned by the Russian violation" of a treaty banning intermediate-range ballistic missiles.
More details about GPT-2 generating fake news can be found in a sample on OpenAI's website.
"Open-source consortiums like OpenAI are showing us what the future of frauds may be like: using automated tools that can be scaled up to massive assaults," said David Doermann, professor of science and engineering at the University at Buffalo. "Right now, the fakes have the upper hand, but we need to keep that gap small so that we can overtake them quickly. The situation has become much like malware, where each new vulnerability exploited by a hacker is patched by a cybersecurity programmer. It could someday become too expensive for fakers to pursue further, but is more likely to remain a back-and-forth game with no clear winner."
In the meantime, according to Doermann, the good guys need to educate the public to take everything on the Internet with a grain of salt; if it sounds too good (or too bad) to be true, then it probably is. "This is not an impossible task. For instance, most people now know not to click on attachments from sources they don't know, and media outlets know how to identify spam and filter it out before it even reaches your inbox," said Doermann. "Likewise, known fakes and even possible fakes could be labeled as such, to alert people to not take them so seriously. And in some cases, such as child pornography, fakes could be filtered out altogether without violating First Amendment rights."
Irakli Beridze, head of the Center for Artificial Intelligence and Robotics of the United Nations International Crime and Justice Research Institute (UNICRI), agrees. "Deep fakes are just a new dimension of the 'manipulated' news problem. The technology has been there, but has only recently been 'democratized,' becoming easier to use through numerous applications, that enable individuals with little technical know-how, to create their own deep fakes," said Beridze. "The spread of deep fakes poses far-reaching challenges that can threaten national security, including threatening elections and public safety, as well as undermining diplomacy, democracy, public discourse, and journalism."
Many organizations are trying to come up with software to make it easier to identify deep fakes, according to Beridze. He and Doermann claim the technological tools to identify deep fakes are already available, needing only to be further developed into turnkey solutions. In the meantime, both agree that more work is needed to reduce the gullibility of the average consumer. Just as the spam problem was raised in public awareness, the awareness of fakes likewise needs to be raised, in what Beridze calls raising "the critical analysis of consumers themselves."
Just last year, UNICRI put forward a deep-fake challenge at the Hackathon for Peace, Justice, and Security in the Hague. The contest challenged participants to create tools for the detection of manipulated videos that can be made available to support law enforcement, security agencies, the judiciary, media and the general public.
"The winning team proposed a neural network architecture for image classification and a web application that simplifies interaction with the user," said Beridze. "This solution—a technological proof of concept—has subsequently been refined in technical workshops during 2019. And in 2020, we are actively working to take this technology from a proof of concept to full-scale usage."
Beridze warns, however, that there is no quick fix for the deep fake problem. He explains that the increasingly fast pace of changing technologies requires more holistic solutions that monitor technological advances and the fakes using them, in order to stay ahead of unseen problems by anticipating next year's more advanced technologies.
"This is a cycling process that requires multi-stakeholder and cross-sectoral collaboration. In this regard, one of the goals of the U.N. Center is to increase and promote knowledge-sharing and relationship-building with stakeholders throughout the public sector, industry, academia, as well as related security entities, intelligence agencies, and counter-terrorism bodies, among others, to help the authorities keep pace," said Beridze. "In doing so, we provide guidance for the development, deployment, and use of solutions that are lawful, trustworthy, and which respect human rights, democracy, justice, and the rule of law."
Fake video and audio are the newest of the fraud innovations driven by bad AI. Arguably, public awareness of such fakes began during the 2016 election year, when 'fake news' became a buzzword. Most of the political videos were obvious fakes, consisting of one-off imitations that merely replaced the lips of politicians with 'April Fool's' versions of their speeches. However, by repurposing AI facial recognition tools using machine learning, programmers have created deep-fake videos that fool even the most sophisticated viewer.
Doermann, formerly program manager for the U.S. Defense Advance Research Project Agency (DARPA) MediFor (Media Forensics) program, says DARPA already has developed automated forensic tools for images obtained by government agencies that once were manual, requiring experts to wield them, but have since been installed into an AI that performs the authentication.
"We developed the AI tools to detect deep fakes long before it became a problem for the public. We were concerned about terrorists and disinformation sources in foreign governments. Our goal was to completely automate the methods used by human experts to identify fakes so that, ideally, every image-based media collected by the government could be run through our authenticator as a matter of course," said Doermann.
MediFor is ongoing, but has entered the stage where the results of its basic research are being incorporated into the final automated tool. Simultaneously, a new program called Semantic Forensics (SemaFor) has picked up the basic research baton. SemaFor aims to take imagery identified as fake and apply AI attribution algorithms to infer where the media originated, along with characterization algorithms that determine whether the fakes were generated for malicious purposes (such as disinformation campaigns) or for benign purposes (such as for entertainment).
For the public, the first deep video fakes that are indistinguishable from genuine campaign videos will likely surface during the 2020 U.S. presidential campaign. Deep fake audio has already been successfully used by cybercriminals in money transfer fraud. For instance, The Wall Street Journal reported that a deep-fake phone call mimicking the voice of a company's chief executive officer (CEO) fooled the company into wiring $243,000 to a cybercriminal. The fake ultimately was discovered, but the money was long gone through a network of wire transfers that could not be traced by authorities.
The brass ring for fraud detection is a validator that can instantly identify and label fakes in real time. The results, unfortunately, will likely be fraudster AI retaliation aimed at fooling the validators in real time.
R. Colin Johnson is a Kyoto Prize Fellow who has worked as a technology journalist for two decades.
Join the Discussion (0)
Become a Member or Sign In to Post a Comment