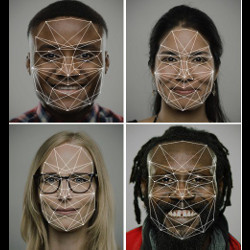
In January 2020, Robert Williams of Farmington Hills, MI, was arrested at his home by the Detroit Police Department. He was photographed, fingerprinted, had his DNA taken, and was then locked up for 30 hours. His crime? He had not committed one; a facial recognition system operated by the Michigan State Police had wrongly identified him as the thief in a 2018 store robbery. However, Williams looked nothing like the perpetrator captured in the surveillance video, and the case was dropped.
A one-off case? Far from it. Rewind to May 2019, when Detroit resident Michael Oliver was arrested after being identified by the very same police facial recognition unit as the person who stole a smartphone from a vehicle. Again, however, Oliver did not even resemble the person pictured in a smartphone video of the theft. His case, too, was dropped, and Oliver has filed a law-suit seeking reputational and economic damages from the police.
What Williams and Oliver have in common is that they are both Black, and biases in deep-learning-based facial recognition systems are known to make such technology highly likely to incorrectly identify people of color. “This is not me. You think all Black people look alike?” an incredulous Williams asked detectives who showed him the CCTV picture of the alleged thief, according to The New York Times. In the Detroit Free Press, Oliver recalled detectives showing him the video of the perpetrator and realizing immediately, “It wasn’t me.”
It is such cases, borne out of the foisting of the privacy-invading mass-surveillance technology on whole populations, that continue to raise major questions over what role facial recognition should have in a civilized society. Dubbed the “plutonium of artificial intelligence” in an appraisal in the ACM journal XRDS, Luke Stark of Microsoft Research’s Montreal lab described facial recognition as “intrinsically socially toxic.” Regardless of the intentions of its makers, he says, “it needs controls so strict that it should be banned for almost all practical purposes.”
Figure. Joy Buolamwini of the Massachusetts Institute of Technology Media Lab is one of many researchers that have found facial recognition technology to be deeply biased with regard to race, gender, age, and other factors.
Such controls are now the subject of ongoing legislative efforts in the U.S., the E.U., and the U.K., where lawmakers are attempting to work out how a technology that Washington, D.C.-based Georgetown University Law Center has characterized as placing populations in a “perpetual police lineup” should be regulated. At the same time, activist groups such as Amnesty International are monitoring the rollout of facial recognition at a human rights level, naming and shaming Western firms that provide the technologies to China’s surveillance state.
With politicians and pressure groups focused on facial recognition’s regulation, deployment, and human rights issues, where does that leave the technologists who actually make the stuff? Can software design and engineering teams charged with developing such systems address at least some of facial recognition technology’s deep-seated problems?
There’s certainly room for them to try. Kush Varshney, a senior researcher in trustworthy artificial intelligence at IBM’s T.J. Watson Research Center in Yorktown Heights, NY, says a raft of researchers have found facial recognition technology to be deeply biased with regard to race, gender, age, and disability, problems engineers can attempt to address. Perhaps the best known of these researchers are Joy Buolamwini of the Massachusetts Institute of Technology Media Lab, and Timnit Gebru of Microsoft Research who, at a Conference on Fairness, Accountability, and Transparency at New York University in 2018, revealed just how badly commercial facial recognition systems fare when attempting to distinguishing gender across races.
The pair had tested three face-based gender classifiers (from IBM, China’s Megvii, and Microsoft) and found the datasets the face recognition systems were trained on to be overwhelmingly (between 79% and 86%) comprised of faces of lighter-skinned people. As a result, they found the systems were skewed to better detect light-skinned people from the outset: the systems misclassified darker-skinned females as men 34% of the time, while lighter-skinned males were only misclassified as female 0.8% of the time.
“All classifiers performed best for lighter individuals and males overall. The classifiers performed worst for darker females,” the researchers wrote in their paper Gender Shades: Intersectional Accuracy Disparities in Commercial Gender Classification.
The critiques did not end there: in late 2019, Patrick Grother and colleagues at the U.S. National Institute for Standards and Technology (NIST) published an exhaustive analysis of 189 face recognition algorithms from 99 developers. Although accuracy varied across algorithms, Grother’s team found that in general, Asian and African faces garnered false positive matches 10 to 100 times more often than the faces of white people. Like Buolamwini and Gebru, they found African-American women experienced the highest rates of false positives. “Differentials in false positives in one-to-many matching are particularly important because the consequences could include false accusations,” the NIST team said in its report.
The NIST team also found that where an algorithm is written can affect its performance. U.S.-developed software, they note, had the highest rates of false positives on faces of Asians, African-Americans, Native Americans, American Indians, Alaskan Indians, and Pacific Islanders. Algorithms developed in Asia, they found, did not have dramatic differences between matching accuracy of Asian and white (Caucasian) faces.
Although accuracy varied across algorithms, Grother’s team found Asian and African faces garnered false positives 10 to 100 times more often than white faces.
In a July 2020 report to Congress on facial recognition, the U.S. Government Accountability Office said this non-deterministic hodgepodge of unpredictable capabilities adds up to a technology that might, or might not, be accurate. As such, it generates performance differences where “higher error rates for certain demographic groups could result in disparate treatment, profiling, or other adverse consequences for members of these populations.”
Worse, the GAO reports there is “no consensus” at all among academics, industry, standards bodies, or independent experts on how to fix the biases behind these “performance differences,” which could have life-changing consequences for the mismatched. Facial recognition performance, the GAO says, depends on multiple algorithmic factors, such as the breadth of ethnicities used in the training data and variables like false-positive threshold settings, as well as photograph-related factors such as pose angle, illumination, skin tone, skin reflectance, expression, cosmetics, spectacle use, and image quality.
It was this proven propensity for dangerous biases (and, therefore, the potential for racist policing) that led IBM, Microsoft, and Amazon to halt entirely, or pause pending hoped-for legislation, their sales of facial recognition technology to police departments. That move was provoked by the police killing of George Floyd, a Black father of five, in Minneapolis, MN, in late May 2020, the event that sparked the global resurgence of the Black Lives Matter movement.
In an early June letter to Congress explaining its pullout from facial recognition sales and R&D, IBM CEO Arvind Krishna said his company “firmly opposes and will not condone uses of any technology, including facial recognition technology,” for “mass surveillance, racial profiling, or violations of basic human rights and freedoms.”
IBM’s move was quickly followed by similar actions from Microsoft and Amazon, which in June 2020 each began one-year moratoria on sales of the technology to law enforcement agencies. The hope of all three firms is that legislation will be forthcoming to ensure facial recognition can only be used in ethical, unbiased ways that respect human rights and avoid racial or gender profiling.
Yet despite these moves, the global market for this biased technology is growing, as the GAO reported facial recognition system revenues were anticipated to grow from $3 billion in 2016 to $10 billion in 2024. In addition, innovation is rocketing: 631 U.S. patents were granted for facial recognition technologies in 2015, a number that grew to 1,497 in 2019, suggesting there is a lot more related (but potentially biased) technology to come.
Although IBM has departed from the facial recognition market, the runaway development of the technology concerns Varshney. After Buolamwini and Gebru showed the API for IBM’s gender classifier to be so error-prone, Varshney said there are just too many points where biases can creep into the development process. “One is specifying the problem, which includes describing what the task is and describing the [facial recognition] metrics by which you’ll be judging the task.
“And then there are the data understanding, data gathering, and data preparation stages. Following that, there is the modeling stage, which is when you’re actually training a neural network, or some other type of model. Then there’s the testing and evaluation phases, and then finally, there’s the deployment phase. And there are issues that crop up in every single part of that complex cycle,” Varshney says.
To fix such issues, he says, facial recognition system developers need to “acquire as diverse a set of images as possible in order to not undersample certain groups.” The best way to do that, Varshney says, is to have as diverse a development team as possible, in terms of members’ races, genders, ages, and disabilities, so everyone can bring what he calls “their lived experience” to the task of specifying the facial recognition problem.
“The broader the set of stakeholders, the broader their set of perspectives and variety of experiences, and the more problems you can identify,” Varshney says.
Taking disability and health as an example, Varshney says a facial recognition system ought to be able to cope with people who have skin conditions, such as vitiligo, which can cause discolored patches on people’s faces. “That is something that you wouldn’t normally think about if you don’t bring in people with different perspectives. And people who have been victims of domestic abuse might have bruises that would create havoc with classification algorithms, too,” Varshney said.
NIST speculates its finding that algorithms developed in Asia are more accurate than those written in the U.S. may be due to some Asian development teams being more diverse. If so, says Grother, “The results are an encouraging sign that more diverse training data may produce more equitable outcomes.”
One facial recognition firm that continues to supply U.S. law enforcement, and which claims to use a very diverse development team, also happens to be the current enfant terrible of the field, Clearview AI of New York City. The firm hit the headlines because it scraped 2.8-billion face photos from publicly accessible Internet sites like Instagram, Facebook, Youtube, Twitter, and LinkedIn, all without user permission. Basically, the firm has created a search engine for any face image hosted on the public Internet.
That vast database already has landed Clearview in trouble with Google, Twitter, and LinkedIn, whose lawyers have issued cease-and-desist orders related to the scraping of their sites. That scraping also is likely to land Clearview AI in hot water in Europe, where GDPR data protection legislation requires individuals to opt in to permit the collection of their personal biometric data. The firm already has ceased operations in Canada, for similar reasons.
Clearview AI CEO Hoan Ton-That makes an extraordinary claim for the technology that company claims is in use by 600 U.S. law enforcement agencies to date: it is bias-free.
“When creating Clearview AI’s algorithm, we made sure to have trained our neural network with training data that reflects each ethnicity in a balanced way. So, unlike other facial recognition algorithms, which haved misidentified people of color, an independent study indicates Clearview AI has no racial bias. As a person of mixed race, this is especially important to me,” Ton-That says.
The study he refers to is one Clear-view AI commissioned itself—and it mimicked to a degree the methodology the American Civil Liberties Union (ACLU) used to test Amazon’s Rekognition system in 2018. ACLU had searched a database of 25,000 images of people who had been arrested using images of 535 members of Congress: Rekognition wrongly matched 28 Congresspersons to arrestees, with that total heavily skewed to politicians of color.
In its test, Clearview AI searched its database of 2.8 billion scraped faces using mugshots of 834 U.S. congressional and state legislators. “No incorrect matches were found…Accuracy was consistent across all racial and demographic groups,” the firm says in a six-page report signed off on by three independent observers: a former New York state judge, an expert in computational linguistics, and a management consultant.
Peter Fussey, director of the Centre for Research into Information, Surveillance, and Privacy (CRISP) at Essex University in the U.K., questions the accuracy of Clearview AI’s self-evaluation. Its brief report, he says, bears no comparison in length and detail to the “comprehensive” NIST facial recognition system studies, adding that the facial recognition expertise of the report’s three adjudicators is also unclear.
Fussey also questions the “ecological validity” of the methodology. “This is the idea that something tested in a lab can be replicated in wider society. For example, testing efficacy on U.S. Congress members that have a great deal of searchable and publicly available photographs in circulation. This does not seem to approximate to the information we have about how the police are using Clearview AI on the public.”
Varshney thinks it’s time people stood back, as IBM has, and realized it is simply not a technology worth keeping. “Face recognition is a particularly thorny technology because it doesn’t have many beneficial uses. There’s just nothing good that can come out of it. It can be used in so many bad ways that even improving the technology could be worse for society,” he says.
Hill, K.
Wrongfully Accused By An Algorithm, The New York Times, June 24, 2020, https://nyti.ms/356Zt8D
Anderson, E.
Facial Recognition Got Him Arrested for a Crime He Didn’t Commit, Detroit Free Press, July 11, 2020, https://bit.ly/3bnpJwN
Buolamwini, J. and Gebru, T.
Gender Shades: Intersectional Accuracy Disparities in Commercial Gender Classification, Proceedings of Machine Learning Research, 81:1–15, 2018, Conference on Fairness, Accountability, and Transparency. https://bit.ly/354ucDu
Grother, P., Ngan, M., and Hanaoka, K.
Face Recognition Vendor Test Part 3: Demographic Effects U.S. National Institute of Standards and Technology, December 2019, https://bit.ly/32Uv1vF
Report to Congressional Requestors, Facial Recognition Technology: Privacy and Accuracy Issues Related to Commercial Uses, U.S. Government Accountability Office, July 2020, https://bit.ly/2DrR5oV
Krishna, A.
IBM CEO’s letter to the U.S. Congress on its abandonment of face recognition technology, June 8, 2020, https://ibm.co/3hXDIM3
Amazon: A one-year moratorium on police use of ‘Rekognition’ Amazon’s COVID-19 blog, June 10, 2020, https://bit.ly/3gTOPUZ
Smith, B.
Microsoft: Facial recognition: It’s Time for Action The Official Microsoft Blog, December 6, 2018, https://bit.ly/3gVScuA
Join the Discussion (0)
Become a Member or Sign In to Post a Comment