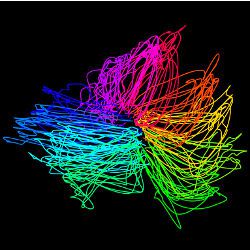
The growth of data volumes in industry and research poses tremendous opportunities, as well as tremendous computational challenges. As data sizes have outpaced the capabilities of single machines, users have needed new systems to scale out computations to multiple nodes. As a result, there has been an explosion of new cluster programming models targeting diverse computing workloads.1,4,7,10 At first, these models were relatively specialized, with new models developed for new workloads; for example, MapReduce4 supported batch processing, but Google also developed Dremel13 for interactive SQL queries and Pregel11 for iterative graph algorithms. In the open source Apache Hadoop stack, systems like Storm1 and Impala9 are also specialized. Even in the relational database world, the trend has been to move away from “one-size-fits-all” systems.18 Unfortunately, most big data applications need to combine many different processing types. The very nature of “big data” is that it is diverse and messy; a typical pipeline will need MapReduce-like code for data loading, SQL-like queries, and iterative machine learning. Specialized engines can thus create both complexity and inefficiency; users must stitch together disparate systems, and some applications simply cannot be expressed efficiently in any engine.
Key Insights
- A simple programming model can capture streaming, batch, and interactive workloads and enable new applications that combine them.
- Apache Spark applications range from finance to scientific data processing and combine libraries for SQL, machine learning, and graphs.
- In six years, Apache Spark has grown to 1,000 contributors and thousands of deployments.
In 2009, our group at the University of California, Berkeley, started the Apache Spark project to design a unified engine for distributed data processing. Spark has a programming model similar to MapReduce but extends it with a data-sharing abstraction called “Resilient Distributed Datasets,” or RDDs.25 Using this simple extension, Spark can capture a wide range of processing workloads that previously needed separate engines, including SQL, streaming, machine learning, and graph processing2,26,6 (see Figure 1). These implementations use the same optimizations as specialized engines (such as column-oriented processing and incremental updates) and achieve similar performance but run as libraries over a common engine, making them easy and efficient to compose. Rather than being specific to these workloads, we claim this result is more general; when augmented with data sharing, MapReduce can emulate any distributed computation, so it should also be possible to run many other types of workloads.24
Spark’s generality has several important benefits. First, applications are easier to develop because they use a unified API. Second, it is more efficient to combine processing tasks; whereas prior systems required writing the data to storage to pass it to another engine, Spark can run diverse functions over the same data, often in memory. Finally, Spark enables new applications (such as interactive queries on a graph and streaming machine learning) that were not possible with previous systems. One powerful analogy for the value of unification is to compare smartphones to the separate portable devices that existed before them (such as cameras, cellphones, and GPS gadgets). In unifying the functions of these devices, smartphones enabled new applications that combine their functions (such as video messaging and Waze) that would not have been possible on any one device.
Since its release in 2010, Spark has grown to be the most active open source project or big data processing, with more than 1,000 contributors. The project is in use in more than 1,000 organizations, ranging from technology companies to banking, retail, biotechnology, and astronomy. The largest publicly announced deployment has more than 8,000 nodes.22 As Spark has grown, we have sought to keep building on its strength as a unified engine. We (and others) have continued to build an integrated standard library over Spark, with functions from data import to machine learning. Users find this ability powerful; in surveys, we find the majority of users combine multiple of Spark’s libraries in their applications.
As parallel data processing becomes common, the composability of processing functions will be one of the most important concerns for both usability and performance. Much of data analysis is exploratory, with users wishing to combine library functions quickly into a working pipeline. However, for “big data” in particular, copying data between different systems is anathema to performance. Users thus need abstractions that are general and composable. In this article, we introduce the Spark programming model and explain why it is highly general. We also discuss how we leveraged this generality to build other processing tasks over it. Finally, we summarize Spark’s most common applications and describe ongoing development work in the project.
Programming Model
The key programming abstraction in Spark is RDDs, which are fault-tolerant collections of objects partitioned across a cluster that can be manipulated in parallel. Users create RDDs by applying operations called “transformations” (such as map, filter
, and groupBy
) to their data.
Spark exposes RDDs through a functional programming API in Scala, Java, Python, and R, where users can simply pass local functions to run on the cluster. For example, the following Scala code creates an RDD representing the error messages in a log file, by searching for lines that start with ERROR
, and then prints the total number of errors:
lines = spark.textFile("hdfs://...")
errors = lines.filter(s => s.startsWith("ERROR"))
println("Total errors: "+errors.count())
The first line defines an RDD backed by a file in the Hadoop Distributed File System (HDFS) as a collection of lines of text. The second line calls the filter
transformation to derive a new RDD from lines
. Its argument is a Scala function literal or closure.a Finally, the last line calls count
, another type of RDD operation called an “action” that returns a result to the program (here, the number of elements in the RDD) instead of defining a new RDD.
Spark evaluates RDDs lazily, allowing it to find an efficient plan for the user’s computation. In particular, transformations return a new RDD object representing the result of a computation but do not immediately compute it. When an action is called, Spark looks at the whole graph of transformations used to create an execution plan. For example, if there were multiple filter
or map
operations in a row, Spark can fuse them into one pass, or, if it knows that data is partitioned, it can avoid moving it over the network for groupBy
.5 Users can thus build up programs modularly without losing performance.
Finally, RDDs provide explicit support for data sharing among computations. By default, RDDs are “ephemeral” in that they get recomputed each time they are used in an action (such as count
). However, users can also persist selected RDDs in memory or for rapid reuse. (If the data does not fit in memory, Spark will also spill it to disk.) For example, a user searching through a large set of log files in HDFS to debug a problem might load just the error messages into memory across the cluster by calling
errors.persist()
After this, the user can run a variety of queries on the in-memory data:
// Count errors mentioning MySQL
errors.filter(s => s.contains("MySQL")).count()
// Fetch back the time fields of errors that
// mention PHP, assuming time is field #3:
errors.filter(s => s.contains("PHP")).map(line => line.split('t')(3)).collect()
This data sharing is the main difference between Spark and previous computing models like MapReduce; otherwise, the individual operations (such as map
and groupBy
) are similar. Data sharing provides large speedups, often as much as 100x, for interactive queries and iterative algorithms.23 It is also the key to Spark’s generality, as we discuss later.
Fault tolerance. Apart from providing data sharing and a variety of parallel operations, RDDs also automatically recover from failures. Traditionally, distributed computing systems have provided fault tolerance through data replication or checkpointing. Spark uses a different approach called “lineage.”25 Each RDD tracks the graph of transformations that was used to build it and reruns these operations on base data to reconstruct any lost partitions. For example, Figure 2 shows the RDDs in our previous query, where we obtain the time fields of errors mentioning PHP by applying two filters
and a map
. If any partition of an RDD is lost (for example, if a node holding an in-memory partition of errors
fails), Spark will rebuild it by applying the filter on the corresponding block of the HDFS file. For “shuffle” operations that send data from all nodes to all other nodes (such as reduceByKey
), senders persist their output data locally in case a receiver fails.
Lineage-based recovery is significantly more efficient than replication in data-intensive workloads. It saves both time, because writing data over the network is much slower than writing it to RAM, and storage space in memory. Recovery is typically much faster than simply rerunning the program, because a failed node usually contains multiple RDD partitions, and these partitions can be rebuilt in parallel on other nodes.
A longer example. As a longer example, Figure 3 shows an implementation of logistic regression in Spark. It uses batch gradient descent, a simple iterative algorithm that computes a gradient function over the data repeatedly as a parallel sum. Spark makes it easy to load the data into RAM once and run multiple sums. As a result, it runs faster than traditional MapReduce. For example, in a 100GB job (see Figure 4), MapReduce takes 110 seconds per iteration because each iteration loads the data from disk, while Spark takes only one second per iteration after the first load.
Integration with storage systems. Much like Google’s MapReduce, Spark is designed to be used with multiple external systems for persistent storage. Spark is most commonly used with cluster file systems like HDFS and key-value stores like S3 and Cassandra. It can also connect with Apache Hive as a data catalog. RDDs usually store only temporary data within an application, though some applications (such as the Spark SQL JDBC server) also share RDDs across multiple users.2 Spark’s design as a storage-system-agnostic engine makes it easy for users to run computations against existing data and join diverse data sources.
Higher-Level Libraries
The RDD programming model provides only distributed collections of objects and functions to run on them. Using RDDs, however, we have built a variety of higher-level libraries on Spark, targeting many of the use cases of specialized computing engines. The key idea is that if we control the data structures stored inside RDDs, the partitioning of data across nodes, and the functions run on them, we can implement many of the execution techniques in other engines. Indeed, as we show in this section, these libraries often achieve state-of-the-art performance on each task while offering significant benefits when users combine them. We now discuss the four main libraries included with Apache Spark.
SQL and DataFrames. One of the most common data processing paradigms is relational queries. Spark SQL2 and its predecessor, Shark,23 implement such queries on Spark, using techniques similar to analytical databases. For example, these systems support columnar storage, cost-based optimization, and code generation for query execution. The main idea behind these systems is to use the same data layout as analytical databases—compressed columnar storage—inside RDDs. In Spark SQL, each record in an RDD holds a series of rows stored in binary format, and the system generates code to run directly against this layout.
Beyond running SQL queries, we have used the Spark SQL engine to provide a higher-level abstraction for basic data transformations called DataFrames,2 which are RDDs of records with a known schema. DataFrames are a common abstraction for tabular data in R and Python, with programmatic methods for filtering, computing new columns, and aggregation. In Spark, these operations map down to the Spark SQL engine and receive all its optimizations. We discuss DataFrames more later.
One technique not yet implemented in Spark SQL is indexing, though other libraries over Spark (such as IndexedRDDs3) do use it.
Spark Streaming. Spark Streaming26 implements incremental stream processing using a model called “discretized streams.” To implement streaming over Spark, we split the input data into small batches (such as every 200 milliseconds) that we regularly combine with state stored inside RDDs to produce new results. Running streaming computations this way has several benefits over traditional distributed streaming systems. For example, fault recovery is less expensive due to using lineage, and it is possible to combine streaming with batch and interactive queries.
GraphX. GraphX6 provides a graph computation interface similar to Pregel and GraphLab,10,11 implementing the same placement optimizations as these systems (such as vertex partitioning schemes) through its choice of partitioning function for the RDDs it builds.
MLlib. MLlib,14 Spark’s machine learning library, implements more than 50 common algorithms for distributed model training. For example, it includes the common distributed algorithms of decision trees (PLANET), Latent Dirichlet Allocation, and Alternating Least Squares matrix factorization.
Combining processing tasks. Spark’s libraries all operate on RDDs as the data abstraction, making them easy to combine in applications. For example, Figure 5 shows a program that reads some historical Twitter data using Spark SQL, trains a K-means clustering model using MLlib, and then applies the model to a new stream of tweets. The data tasks returned by each library (here the historic tweet RDD and the K-means model) are easily passed to other libraries. Apart from compatibility at the API level, composition in Spark is also efficient at the execution level, because Spark can optimize across processing libraries. For example, if one library runs a map
function and the next library runs a map
on its result, Spark will fuse these operations into a single map
. Likewise, Spark’s fault recovery works seamlessly across these libraries, recomputing lost data no matter which libraries produced it.
Spark has a similar programming model to MapReduce but extends it with a data-sharing abstraction called “resilient distributed datasets,” or RDDs.
Performance. Given that these libraries run over the same engine, do they lose performance? We found that by implementing the optimizations we just outlined within RDDs, we can often match the performance of specialized engines. For example, Figure 6 compares Spark’s performance on three simple tasks—a SQL query, streaming word count, and Alternating Least Squares matrix factorization—versus other engines. While the results vary across workloads, Spark is generally comparable with specialized systems like Storm, GraphLab, and Impala.b For stream processing, although we show results from a distributed implementation on Storm, the per-node through-put is also comparable to commercial streaming engines like Oracle CEP.26
Even in highly competitive benchmarks, we have achieved state-of-the-art performance using Apache Spark. In 2014, we entered the Daytona Gray-Sort benchmark (http://sortbenchmark.org/) involving sorting 100TB of data on disk, and tied for a new record with a specialized system built only for sorting on a similar number of machines. As in the other examples, this was possible because we could implement both the communication and CPU optimizations necessary for large-scale sorting inside the RDD model.
Applications
Apache Spark is used in a wide range of applications. Our surveys of Spark users have identified more than 1,000 companies using Spark, in areas from Web services to biotechnology to finance. In academia, we have also seen applications in several scientific domains. Across these workloads, we find users take advantage of Spark’s generality and often combine multiple of its libraries. Here, we cover a few top use cases. Presentations on many use cases are also available on the Spark Summit conference website (http://www.spark-summit.org).
Batch processing. Spark’s most common applications are for batch processing on large datasets, including Extract-Transform-Load workloads to convert data from a raw format (such as log files) to a more structured format and offline training of machine learning models. Published examples of these workloads include page personalization and recommendation at Yahoo!; managing a data lake at Goldman Sachs; graph mining at Alibaba; financial Value at Risk calculation; and text mining of customer feedback at Toyota. The largest published use case we are aware of is an 8,000-node cluster at Chinese social network Tencent that ingests 1PB of data per day.22
While Spark can process data in memory, many of the applications in this category run only on disk. In such cases, Spark can still improve performance over MapReduce due to its support for more complex operator graphs.
Interactive queries. Interactive use of Spark falls into three main classes. First, organizations use Spark SQL for relational queries, often through business-intelligence tools like Tableau. Examples include eBay and Baidu. Second, developers and data scientists can use Spark’s Scala, Python, and R interfaces interactively through shells or visual notebook environments. Such interactive use is crucial for asking more advanced questions and for designing models that eventually lead to production applications and is common in all deployments. Third, several vendors have developed domain-specific interactive applications that run on Spark. Examples include Tresata (anti-money laundering), Trifacta (data cleaning), and PanTera (large-scale visualization, as in Figure 7).
Stream processing. Real-time processing is also a popular use case, both in analytics and in real-time decision-making applications. Published use cases for Spark Streaming include network security monitoring at Cisco, prescriptive analytics at Samsung SDS, and log mining at Netflix. Many of these applications also combine streaming with batch and interactive queries. For example, video company Conviva uses Spark to continuously maintain a model of content distribution server performance, querying it automatically when it moves clients across servers, in an application that requires substantial parallel work for both model maintenance and queries.
Scientific applications. Spark has also been used in several scientific domains, including large-scale spam detection,19 image processing,27 and genomic data processing.15 One example that combines batch, interactive, and stream processing is the Thunder platform for neuroscience at Howard Hughes Medical Institute, Janelia Farm.5 It is designed to process brain-imaging data from experiments in real time, scaling up to 1TB/hour of whole-brain imaging data from organisms (such as zebrafish and mice). Using Thunder, researchers can apply machine learning algorithms (such as clustering and Principal Component Analysis) to identify neurons involved in specific behaviors. The same code can be run in batch jobs on data from previous runs or in interactive queries during live experiments. Figure 8 shows an example image generated using Spark.
Spark components used. Because Spark is a unified data-processing engine, the natural question is how many of its libraries organizations actually use. Our surveys of Spark users have shown that organizations do, indeed, use multiple components, with over 60% of organizations using at least three of Spark’s APIs. Figure 9 outlines the usage of each component in a July 2015 Spark survey by Databricks that reached 1,400 respondents. We list the Spark Core API (just RDDs) as one component and the higher-level libraries as others. We see that many components are widely used, with Spark Core and SQL as the most popular. Streaming is used in 46% of organizations and machine learning in 54%. While not shown directly in Figure 9, most organizations use multiple components; 88% use at least two of them, 60% use at least three (such as Spark Core and two libraries), and 27% use at least four components.
Deployment environments. We also see growing diversity in where Apache Spark applications run and what data sources they connect to. While the first Spark deployments were generally in Hadoop environments, only 40% of deployments in our July 2015 Spark survey were on the Hadoop YARN cluster manager. In addition, 52% of respondents ran Spark on a public cloud.
Why Is the Spark Model General?
While Apache Spark demonstrates that a unified cluster programming model is both feasible and useful, it would be helpful to understand what makes cluster programming models general, along with Spark’s limitations. Here, we summarize a discussion on the generality of RDDs from Zaharia.24 We study RDDs from two perspectives. First, from an expressiveness point of view, we argue that RDDs can emulate any distributed computation, and will do so efficiently in many cases unless the computation is sensitive to network latency. Second, from a systems point of view, we show that RDDs give applications control over the most common bottleneck resources in clusters—network and storage I/O—and thus make it possible to express the same optimizations for these resources that characterize specialized systems.
Expressiveness perspective. To study the expressiveness of RDDs, we start by comparing RDDs to the MapReduce model, which RDDs build on. The first question is what computations can MapReduce itself express? Although there have been numerous discussions about the limitations of MapReduce, the surprising answer here is that MapReduce can emulate any distributed computation.
To see this, note that any distributed computation consists of nodes that perform local computation and occasionally exchange messages. MapReduce offers the map
operation, which allows local computation, and reduce
, which allows all-to-all communication. Any distributed computation can thus be emulated, perhaps somewhat inefficiently, by breaking down its work into timesteps, running maps to perform the local computation in each timestep, and batching and exchanging messages at the end of each step using a reduce. A series of MapReduce steps will capture the whole result, as in Figure 10. Recent theoretical work has formalized this type of emulation by showing that MapReduce can simulate many computations in the Parallel Random Access Machine model.8 Repeated Map-Reduce is also equivalent to the Bulk Synchronous Parallel model.20
While this line of work shows that MapReduce can emulate arbitrary computations, two problems can make the “constant factor” behind this emulation high. First, MapReduce is inefficient at sharing data across timesteps because it relies on replicated external storage systems for this purpose. Our emulated system may thus become slower due to writing out its state after each step. Second, the latency of the MapReduce steps determines how well our emulation will match a real network, and most Map-Reduce implementations were designed for batch environments with minutes to hours of latency.
RDDs and Spark address both of these limitations. On the data-sharing front, RDDs make data sharing fast by avoiding replication of intermediate data and can closely emulate the in-memory “data sharing” across time that would happen in a system composed of long-running processes. On the latency front, Spark can run MapReduce-like steps on large clusters with 100ms latency; nothing intrinsic to the MapReduce model prevents this. While some applications need finer-grain timesteps and communication, this 100ms latency is enough to implement many data-intensive workloads, where the amount of computation that can be batched before a communication step is high.
In summary, RDDs build on Map-Reduce’s ability to emulate any distributed computation but make this emulation significantly more efficient. Their main limitation is increased latency due to synchronization in each communication step, but this latency is often not a factor.
Systems perspective. Independent of the emulation approach to characterizing Spark’s generality, we can take a systems approach. What are the bottleneck resources in cluster computations? And can RDDs use them efficiently? Although cluster applications are diverse, they are all bound by the same properties of the underlying hardware. Current datacenters have a steep storage hierarchy that limits most applications in similar ways. For example, a typical Hadoop cluster might have the following characteristics:
Local storage. Each node has local memory with approximately 50GB/s of bandwidth, as well as 10 to 20 local disks, for approximately 1GB/s to 2GB/s of disk bandwidth;
Links. Each node has a 10Gbps (1.3GB/s) link, or approximately 40x less than its memory bandwidth and 2x less than its aggregate disk bandwidth; and
Racks. Nodes are organized into racks of 20 to 40 machines, with 40Gbps–80Gbps bandwidth out of each rack, or 2x–5x lower than the in-rack network performance.
Given these properties, the most important performance concern in many applications is the placement of data and computation in the network. Fortunately, RDDs provide the facilities to control this placement; the interface lets applications place computations near input data (through an API for “preferred locations” for input sources25), and RDDs provide control over data partitioning and colocation (such as specifying that data be hashed by a given key). Libraries (such as GraphX) can thus implement the same placement strategies used in specialized systems.6
Beyond network and I/O bandwidth, the most common bottleneck tends to be CPU time, especially if data is in memory. In this case, however, Spark can run the same algorithms and libraries used in specialized systems on each node. For example, it uses columnar storage and processing in Spark SQL, native BLAS libraries in MLlib, and so on. As we discussed earlier, the only area where RDDs clearly add a cost is network latency, due to the synchronization at parallel communication steps.
One final observation from a systems perspective is that Spark may incur extra costs over some of today’s specialized systems due to fault tolerance. For example, in Spark, the map tasks in each shuffle operation save their output to local files on the machine where they ran, so reduce tasks can refetch it later. In addition, Spark implements a barrier at shuffle stages, so the reduce tasks do not start until all the maps have finished. This avoids some of the complexity that would be needed for fault recovery if one “pushed” records directly from maps to reduces in a pipelined fashion. Although removing some of these features would speed up the system, Spark often performs competitively despite them. The main reason is an argument similar to our previous one: many applications are bound by an I/O operation (such as shuffling data across the network or reading it from disk) and beyond this operation, optimizations (such as pipelining) add only a modest benefit. We have kept fault tolerance “on” by default in Spark to make it easy to reason about applications.
Ongoing Work
Apache Spark remains a rapidly evolving project, with contributions from both industry and research. The codebase size has grown by a factor of six since June 2013, with most of the activity in new libraries. More than 200 third-party packages are also available.c In the research community, multiple projects at Berkeley, MIT, and Stanford build on Spark, and many new libraries (such as GraphX and Spark Streaming) came from research groups. Here, we sketch four of the major efforts.
DataFrames and more declarative APIs. The core Spark API was based on functional programming over distributed collections that contain arbitrary types of Scala, Java, or Python objects. While this approach was highly expressive, it also made programs more difficult to automatically analyze and optimize. The Scala/Java/Python objects stored in RDDs could have complex structure, and the functions run over them could include arbitrary code. In many applications, developers could get suboptimal performance if they did not use the right operators; for example, the system on its own could not push filter
functions ahead of maps.
To address this problem, we extended Spark in 2015 to add a more declarative API called DataFrames2 based on the relational algebra. Data frames are a common API for tabular data in Python and R. A data frame is a set of records with a known schema, essentially equivalent to a database table, that supports operations like filtering and aggregation using a restricted “expression” API. Unlike working in the SQL language, however, data frame operations are invoked as function calls in a more general programming language (such as Python and R), allowing developers to easily structure their program using abstractions in the host language (such as functions and classes). Figure 11 and Figure 12 show examples of the API.
Spark’s DataFrames offer a similar API to single-node packages but automatically parallelize and optimize the computation using Spark SQL’s query planner. User code thus receives optimizations (such as predicate pushdown, operator reordering, and join algorithm selection) that were not available under Spark’s functional API. To our knowledge, Spark DataFrames are the first library to perform such relational optimizations under a data frame API.d
While DataFrames are still new, they have quickly become a popular API. In our July 2015 survey, 60% of respondents reported using them. Because of the success of DataFrames, we have also developed a type-safe interface over them called Datasetse that lets Java and Scala programmers view DataFrames as statically typed collections of Java objects, similar to the RDD API, and still receive relational optimizations. We expect these APIs to gradually become the standard abstraction for passing data between Spark libraries.
Performance optimizations. Much of the recent work in Spark has been on performance. In 2014, the Databricks team spent considerable effort to optimize Spark’s network and I/O primitives, allowing Spark to jointly set a new record for the Daytona GraySort challenge.f Spark sorted 100TB of data 3x faster than the previous record holder based on Hadoop MapReduce using 10x fewer machines. This benchmark was not executed in memory but rather on (solid-state) disks. In 2015, one major effort was Project Tungsten,g which removes Java Virtual Machine overhead from many of Spark’s code paths by using code generation and non-garbage-collected memory. One benefit of doing these optimizations in a general engine is that they simultaneously affect all of Spark’s libraries; machine learning, streaming, and SQL all became faster from each change.
R language support. The SparkR project21 was merged into Spark in 2015 to provide a programming interface in R. The R interface is based on DataFrames and uses almost identical syntax to R’s built-in data frames. Other Spark libraries (such as MLlib) are also easy to call from R, because they accept DataFrames as input.
Research libraries. Apache Spark continues to be used to build higher-level data processing libraries. Recent projects include Thunder for neuroscience,5 ADAM for genomics,15 and Kira for image processing in astronomy.27 Other research libraries (such as GraphX) have been merged into the main codebase.
Conclusion
Scalable data processing will be essential for the next generation of computer applications but typically involves a complex sequence of processing steps with different computing systems. To simplify this task, the Spark project introduced a unified programming model and engine for big data applications. Our experience shows such a model can efficiently support today’s workloads and brings substantial benefits to users. We hope Apache Spark highlights the importance of composability in programming libraries for big data and encourages development of more easily interoperable libraries.
All Apache Spark libraries described in this article are open source at http://spark.apache.org/. Databricks has also made videos of all Spark Summit conference talks available for free at https://spark-summit.org/.
Acknowledgments
Apache Spark is the work of hundreds of open source contributors who are credited in the release notes at https://spark.apache.org. Berkeley’s research on Spark was supported in part by National Science Foundation CISE Expeditions Award CCF-1139158, Lawrence Berkeley National Laboratory Award 7076018, and DARPA XData Award FA8750-12-2-0331, and gifts from Amazon Web Services, Google, SAP, IBM, The Thomas and Stacey Siebel Foundation, Adobe, Apple, Arimo, Blue Goji, Bosch, C3Energy, Cisco, Cray, Cloudera, EMC2, Ericsson, Facebook, Guavus, Huawei, Informatica, Intel, Microsoft, NetApp, Pivotal, Samsung, Schlumberger, Splunk, Virdata, and VMware.
Figures
Figure 1. Apache Spark software stack, with specialized processing libraries implemented over the core engine.
Figure 2. Lineage graph for the third query in our example; boxes represent RDDs, and arrows represent transformations.
Figure 3. A Scala implementation of logistic regression via batch gradient descent in Spark.
Figure 4. Performance of logistic regression in Hadoop MapReduce vs. Spark for 100GB of data on 50 m 2.4xlarge EC2 nodes.
Figure 5. Example combining the SQL, machine learning, and streaming libraries in Spark.
Figure 6. Comparing Spark’s performance with several widely used specialized systems for SQL, streaming, and machine learning. Data is from Zaharia24 (SQL query and streaming word count) and Sparks et al.17 (alternating least squares matrix factorization).
Figure 7. PanTera, a visualization application built on Spark that can interactively filter data.
Figure 8. Visualization of neurons in the zebrafish brain created with Spark, where each neuron is colored based on the direction of movement that correlates with its activity. Source: Jeremy Freeman and Misha Ahrens of Janelia Research Campus.
Figure 9. Percent of organizations using each Spark component, from the Databricks 2015 Spark survey; https://databricks.com/blog/2015/09/24/.
Figure 10. Emulating an arbitrary distributed computation with MapReduce.
Figure 11. Example of Spark’s DataFrame API in Python. Unlike Spark’s core API, DataFrames have a schema with named columns (such as age and city) and take expressions in a limited language (such as age > 20) instead of arbitrary Python functions.
Figure 12. Working with DataFrames in Spark’s R API. We load a distributed DataFrame using Spark’s JSON data source, then filter and aggregate using standard R column expressions.
Figure. Analyses performed using Spark of brain activity in a larval zebrafish: (left) matrix factorization to characterize functionally similar regions (as depicted by different colors) and (right) embedding dynamics of whole-brain activity into lower-dimensional trajectories. Source: Jeremy Freeman and Misha Ahrens, Janelia Research Campus, Howard Hughes Medical Institute, Ashburn, VA.
Figure. Watch the authors discuss their work in this exclusive Communications video. http://cacm.acm.org/videos/spark
Join the Discussion (0)
Become a Member or Sign In to Post a Comment