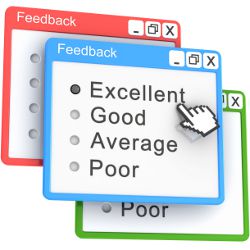
As commerce increasingly moves online, consumers worldwide are relying more and more on online reviews to help them make decisions about products to buy and businesses to patronize.
According to a recent study by BrightLocal (http://selnd.com/1xzy0Xb), 88% of U.S. consumers read online reviews “to determine whether a local business is a good business” at least occasionally—39% do so regularly. Also, 72% say positive reviews lead them to trust a business more, while 88% say that in “the right circumstances,” they trust online reviews as much as personal recommendations.
What are “the right circumstances?” In response to that last question in the BrightLocal survey, 30% said they trust online reviews if they believe they are authentic.
The problem of authenticity in online reviews is a long-standing and stubborn one. In one famous incident back in 2004, Amazon’s Canadian site accidentally revealed the true identities of thousands of its previously anonymous U.S. book reviewers. One insight the mistake revealed was that many authors were using fake names in order to give their own books favorable reviews.
The value of a positive review has also led to various review-for-hire operations, in which Web users get paid to write reviews on sites such as Amazon and Yelp. Bing Liu, professor of computer science at the University of Illinois at Chicago and author of Sentiment Analysis and Opinion Mining, told The New York Times in 2012 that about a third of all Internet reviews were fake.
In their 2013 paper titled “Fake It Till You Make It: Reputation, Competition, and Yelp Review Fraud,” Michael Luca, assistant professor of business administration at the Harvard Business School, and Georgios Zervas, assistant professor of marketing at Boston University School of Management, estimated 16% of Yelp restaurant reviews were fraudulent.
The effects of rampant review fraud can be devastating for both businesses and consumers.
The effects of rampant review fraud can be devastating for both businesses and consumers. Sometimes fake reviews are posted by business owners either leaving negative reviews for their competitors, or positive reviews for their own businesses. Having to combat false negative reviews can take a lot of time and energy that a business would better apply elsewhere. In addition, many small business owners are not sufficiently computer-savvy to stay on top of such attacks.
The importance of online reviews also leaves a business open to extortion, in which customers threaten to write a negative review if they do not get special treatment—a form of fraudulent review, since it would not reflect how an average customer actually was treated treated by the business.
For consumers, a fraudulent review can lead to negative outcomes. A traveler researching places to stay on TripAdvisor, a site built on customer reviews of hotels and restaurants, could be led by a false positive review to stay somewhere that is not up to their standards, or which does not provide the specific amenities described. A fraudulent review on Amazon could result in the purchase of a shoddy or unwanted product.
How to Spot a Fake Review
Because of the potential for negative experiences—for which consumers may blame the website where they read the erroneous review—efforts to guarantee the honesty and authenticity of online reviews have attracted the attention of researchers.
There are several ways consumers themselves can get a good idea of whether a review is fraudulent or not. On most sites, a potential reviewer must create a user account. That may not (and usually does not) reveal their real name, but it does provide a way of checking to see if the reviewer has reviewed anything else. It certainly is possible for someone to legitimately post just one review—for example, if they join TripAdvisor specifically to rave or complain about someplace they went on a recent trip—but in general, a reviewer with just a single review can be considered a red flag.
Computer algorithms can also help detect fake reviews, and this is an area prime for computer science research. It can be difficult to evaluate the authenticity of a single reviewer, but there are ways to identify fraudulent reviewers operating as a group. Creating a single user account and posting one review of a hotel or restaurant is not likely to be effective at influencing the general opinion of a community, but several reviews all saying the same thing can have a major impact and can subsequently dominate the conversation. To efficiently flood a site that way, a group needs to automate the creation of user accounts. That leads to one way an algorithm can identify such a false source: usernames with more than three numbers at the end strongly suggest accounts created by an automated program. Several reviews by different user names with numbers at the end could indicate a group of review spammers.
Researchers are looking at ways to detect collusion among fake reviewers based on the review contents. Bing Liu worked with Arjun Mukherjee, a Ph.D. candidate at the University of Illinois at Chicago, and Natalie Glance, a Google software engineer interested in social shopping, on a software algorithm to detect such activities. In a paper presented at the World Wide Web 2012 conference, they described their use of a behavioral model called GSRank to detect fake reviewer groups. Their model evaluated relationships among reviewers, groups, and products to detect clusters of people who have all reviewed the same set of products.
In his book, Liu discusses other methods of detecting fraudulent reviews. He cites such clues as consistent word choices across reviews, similarity of content and style among different reviewers, reviewers that fail to maintain a consistent identity (for example, referring to “my husband” in one review and “my wife” in another), multiple reviews from a single user all posted within a short period of time, multiple reviews from a suspected group posted around the same time, and so on. All of these things are clues an algorithm could pick up to suggest the reviews were fraudulent.
These are the kinds of indicators Yelp’s filtering algorithm looks at, according to Yelp PR specialist Hannah Cheesman. “Our recommendation software takes a large amount of data into account, such as email address, IP address, pattern recognition, etc., to determine which reviews we know enough about to recommend,” says Cheesman. “The software is engineered to weed out possible fakes (several reviews generated from the same IP address), biased reviews (written by a competitor or solicited by a business owner from friends, family, or favored customers), unhelpful rants or raves, and reviews written by users we just don’t know much about (no friends, no picture, few reviews, etc.) and therefore can’t recommend to our community.”
That last point—what the community knows about the reviewer—is a primary concern for Sorin Adam Matei, an associate professor of communications at Purdue University whose work deals with trust, reputation, and authority in social communities. Matei is a cofounder of Kredible.net, a community of researchers interested in ways to define those qualities, as well as coeditor of the forthcoming Roles, Trust, and Reputation in Social Media Knowledge Markets (part of the Springer Computational Social Sciences series). For Matei, one key to identifying trustworthy online reviews is the track record of the reviewers. “Trust and credibility in the context of social media depend primarily on functional relationships. What you do is more important than who you are,” he says. Whether human or algorithm, following the activity of a reviewer is how you know whether you can trust his or her reviews.
What to Do with Fake Reviews
All these techniques can suggest a review is fraudulent, but by themselves they do not solve the problem of how a site can make certain its visitors can trust the reviews it hosts. One approach to resolving that doubt is exemplified by Yelp, which separates recommended reviews from those that do not satisfy its screening standards. The latter are still available for users to read, but they are marked as “not recommended,” with an explanation of what that means.
One approach to resolving that doubt is exemplified by Yelp, which separates recommended reviews from those that do not satisfy its screening standards.
Other approaches involve limiting who can write reviews in the first place. Ben Shneiderman, a professor of computer science at the University of Maryland, contributed the chapter “Building Trusted Social Media Communities: A Research Roadmap for Promoting Credible Content” to the book Matei edited. He writes, “Many strategies are being tried to ensure that only trusted contributors participate, such as raising the barriers to entry for contributors by requiring a log-in (no anonymous contributions), identity verification, background check, probation periods, and public performance histories. Greater transparency about who the contributors are and what their past is has the potential to increase trust in their future contributions.”
Transparency is a vital element, in the view of Purdue’s Matei. “Trust and credibility are the product of the transparency of a user’s activities multiplied by the speed and the cost of verifying them,” he explains. “Individuals that interact online through ratings, reviewing, collaboration, or shopping trust each other on the basis of the traceable activity they leave behind. The easier it is to track them, the more trust and credibility are generated.” He cites eBay as a good example of how that can be implemented: users can rate both buyers and sellers, and their overall rating is visible to all.
Amazon, on the other hand, does not do as good a job, in Matei’s view. “The credibility of its reviewers is mostly a function of productivity,” he says. Amazon does provide links to let users examine a reviewer’s other reviews, and notes whether the reviewer is known to have purchased the item being reviewed (although, of course, if the reviewer purchased it elsewhere, Amazon would not know about it). Some reviewers are in the site’s “Hall of Fame,” meaning other visitors have rated their reviews as helpful, “but the exact algorithm and the numbers behind it are not immediately available,” says Matei.
Matei also points to Wikipedia as a site that, despite its size and popularity, has its credibility questioned because no one really knows who its authors and editors are.
Another approach to promoting trustworthy reviews is illustrated by Reddit, a community where the visibility of content is determined by up-and-down votes from its users. When a user visits a Reddit community, what they see first is based on the opinion of the other members about its relative value. That can be distorted, of course—people tend to vote down comments they disagree with, regardless of their quality—but in general, it represents a consensus about the value of content.
It is vital for e-commerce sites and social media communities for participants to be confident the reviews they read are authentic. As more and more people do their shopping online and rely on strangers’ recommendations, the ability to filter out fraudulent reviews, whether left by a business’s competitors or by non-customers hired for the purpose, becomes of critical importance. Computer science can help with ways to both identify and sequester fraudulent reviews and to promote trustworthy ones, and sites can implement measures to help visitors track reviewers’ activities and judge which are the most reliable.
Further Reading
Bertino, E. and Matei, Sorin Adam, eds.
Roles, Trust, and Reputation in Social Media Knowledge Markets, Springer (2014).
Hu, N., Liu, L., and Sambamurthy, V.
Fraud Detection in Online Consumer Reviews, Decision Support Systems, vol. 50, no. 3 (2011).
Liu, B.
Sentiment Analysis and Opinion Mining (Synthesis Lectures on Human Language Technologies), Morgan & Claypool (2012).
Liu, B., Mukherjee, A., and Glance, N.
Spotting Fake Reviewer Groups in Consumer Reviews, Proceedings of the 2012 World Wide Web Conference, http://www2012.org/proceedings/proceedings/p191.pdf.
Luca, M. and Zervas, G.
Fake It Till You Make It: Reputation, Competition, and Yelp Review Fraud, Harvard Business School NOM Unit Working Paper No. 14-006 (2013).
Figures
Figure 1. Yelp lets users see reviews that do not satisfy its authentication algorithm, but explains why they are in a separate section.
Figure 2. Yelp uses algorithms to ensure reviews in the “Recommended Reviews” section are authentic; this also provides a way to check a reviewer’s activity on the site.
Figure 3. Amazon provides tools to evaluate the authenticity of reviews, such as links to all a reviewer’s activities, and verification they actually own a product they review.
Figure 4. A framework for analysis of social media communities. From Roles, Trust, and Reputation in Social Media Knowledge Markets, courtesy of Ben Shneiderman.
Join the Discussion (0)
Become a Member or Sign In to Post a Comment