November 1990 - Vol. 33 No. 11
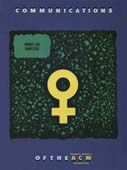
Features
Personal computing: Windows, DOS and the MAC
Direct-manipulation or graphical user interfaces (GUIs) are nearly as old as command-line interfaces.1 At the ACM Conference on The History of Personal Workstations, Doug…
Legally speaking: how to interpret the Lotus decision (and how not to)
On June 28, 1990, a federal court judge in Boston made public his decision in favor of Lotus Development Corporation in its software copyright lawsuit against Paperback…
There is mounting evidence that many women opting for careers in computing either drop out of the academic pipeline or choose not to get advanced degrees and enter…
It is well known that women are significantly underrepresented in scientific fields in the United States, and computer science is no exception. As of 1987- 1988, women…
Connectionist ideas and algorithms
In our quest to build intelligent machines, we have but one naturally occurring model: the human brain. It follows that one natural idea for artificial intelligence (AI)…
Transaction processing monitors
A transaction processing (TP) application is a program that performs an administrative function by accessing a shared database on behalf of an on-line user. A TP system…
SCISOR: extracting information from on-line news
The future of natural language text processing is examined in the SCISOR prototype. Drawing on artificial intelligence techniques, and applying them to financial news…
An object-oriented relational database
A relational DBMS and an object-oriented programming language can be combined to yield a surprisingly effective OO-DBMS for many applications.
Inside risks: risks in computerized elections
Background: Errors and alleged fraud in computer-based elections have been recurring Risks Forum themes. The state of the computing art continues to be primitive.…