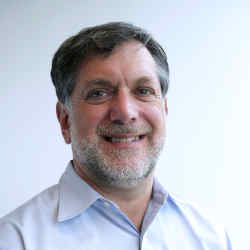
Opportunities to investigate and improve how people learn beckon today’s computer scientists for three reasons. By choosing learning as a topic area, computer scientists can contribute to major societal challenges, win grants in new programs, and tackle new computer science challenges.
In a recent example that touches on each of these reasons, the University of Colorado Boulder announced the award of a $20-million AI Institute National Science Foundation award to address the pressing societal need to wisely use AI technologies in today’s schools and other learning contexts. Over five years, this institute plans to investigate how AI can enable students in underrepresented populations to learn collaboration and teamwork skills necessary to reach their fullest potential in our knowledge society. Although applied research is a part of the plan, the bulk of the effort aims for fundamental AI advances to make sense of collaborative interactions and to take action in support of orchestrating multi-person/multi-device teamwork.
The National Science Foundation (NSF) is currently calling for proposals for another AI Institute to address learning, again with the potential to win a $20-million award. Further, the NSF RETTL program has a January 25, 2021 due date and explicitly invites computer scientists to partner with learning scientists to explore emerging new technologies that could transform learning. Looking beyond federal funding, Schmidt Futures has been investing to bring data scientists and learning researchers together around shared platforms. There are similar opportunities in Europe, Asia, and other parts of the world.
These new funding programs call for strong collaborations between computer scientists and learning scientists. Over the past decades, the science of learning has advanced dramatically. Funders are realizing that to tackle profound educational challenges, integrating computer and learning science perspectives will be necessary.
My own experience bears this out. For the past eight years, I led the Center for Innovative Research in Cyberlearning, that was a community hub for NSF-funded convergent research teams of computer and learning scientists. Over 450 projects were funded. We saw deep interdisciplinary collaborations to tackle the potential of technologies like multimodal analytics, augmented reality, haptic interactions and national language processing to enhance learning, along with the development of necessary theories to guide this, around concepts like embodied cognition, development of STEM identities, and social learning.
The spirit of collaboration among disciplines has also been accelerating worldwide. For example, ACM’s Learning@Scale participated in a major event in London in 2018 that brought three conferences under one roof — Learning@Scale, AI and Education and the Learning Sciences — highlighting the opportunities to connect research finding across disciplines. Now I lead a successor center, CIRCLS.org which will support interdisciplinary collaboration in the RETTL community and interconnect with AI Institutes as they are funded. Indeed, the center recently released an expert panel report on AI and the Future of Learning.
Within these collaborations, I see three broad dimensions that capture why computer science problems in education are so challenging and worthy of computer scientists’ attention.
1. Learning is constructive and social. Learning these days is widely understood to be social. Learners actively construct their understandings of concepts with peers and mentors over time, often as they build something tangible. Imagine, for example, a team of students in a robotics competition working together to program a robot to solve a series of complex missions — learning happens as students work together over time to build something complex. The learning data in such situations is noisy, involves speech, gesture, physical action and written code. An AI agent or system must address dual learning goals: how students collaborate to learn and how students learn to collaborate. For computer scientists, sensing, analyzing and acting in rich social and constructive situations could fruitfully push the state of the art.
2. Learning is longitudinal and participatory. Important learning outcomes we often care about (e.g., broadening participation in computer science education and careers) are not achieved in a singular or episodic manner. Learning occurs over time and involves participation in many levels and kinds of organizational structures—study groups, classrooms, informal out-of-school activities, clubs, and more. Large, longitudinal data sets are frequently available in education. Further, opportunities to collect learning data over time and as learners participate in different settings is expanding rapidly. Challenging problems lie in making sense of data sets that have long-term time trajectories and where individuals participate in overlapping structures across different groups and settings. For example, how can we uncover students growing competencies in a soft skill like “leading collaborative teams” across settings?
3. Learning is contextual and should be equitable. Becoming involved in research with the aim to improve learning brings a scientist face to face with the realities of education as occurring in specific contexts and requiring attention to equity and ethics. Actors in a learning environment bring different purposes, identities and abilities to the table; learner variability is an essential challenge. Successful learning requires culturally responsive approaches; it recognizes student agency and draws upon assets in the local context. Further, challenges of responsible computing are front and center as learning applications need to take extra care to be unbiased, transparent, and equitable.
These dimensions provide opportunities to apply and advance computer science expertise. For example, within the new Boulder institute, I am aware of prior research that built upon the availability of national data sets of video and audio recordings of complex classroom interactions; interactions that had already been rigorously coded by human observers for features relevant to learning theory. When I last checked in with the Boulder team last summer, they had made progress automatically analyzing both video and speech of student-teacher interactions in classrooms using learning analytics approaches, work that is challenging because behavior in classrooms is noisy and messy. Further, deciding how an AI agent might act to support student-teacher interaction is also very challenging, as teachers and students are in the midst of complex performances and are often already using all their available cognitive processing power — providing complex, cockpit-like dashboards rarely meets teachers’ needs. Other groups are working on augmented reality heads up displays, virtual simulated students and classrooms for training teachers, and tools to support “orchestrating” the work of all the actors in a classroom. All of the above dimensions come alive in a situation like this, and present opportunities that I believe could drive both computer science and learning sciences forward.
In the midst of the coronavirus pandemic, many computer scientists are now at home seeing the limitations of today’s scalable technologies for learning. We can and must do better. The funding is available, the opportunities for societal impact are obvious, and the computer science problems are challenging. Now is a great time for computer scientists to form teams with learning scientists to do good in education while also pursuing more fundamental computing advances.
Jeremy Roschelle is Executive Director of Learning Sciences Research at Digital Promise and a Fellow of the International Society of the Learning Sciences.
Join the Discussion (0)
Become a Member or Sign In to Post a Comment