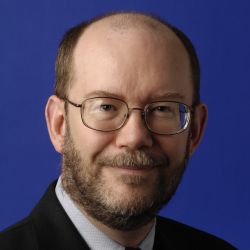
"Big data" is the meme of the day. Like all such phrases, it is a tabula rasa on which everyone writes their own version of the tale. What then, is big data? Superficially, it is data so large that it challenges one’s standard methods for storage, processing and analysis. Like all adjectives, big likes in the eye of the beholder. If your traditional approach to data management has been based on spreadsheets, you may view gigabytes as big data. Conversely, if you are operating a social network site or a major search engine, big has an entirely different meaning, where a petabyte is often the smallest unit of measure worth discussing.
Although much of the sturm und drang surrounding big data has focused on the deluge of data from online consumer behavior — web site visits and cookies, social network interactions, search engine queries and online retailing — there are equally daunting, though different big data problems in science and engineering. The scale and scope of data produced by a new generation of instruments, from domains as diverse as astronomy and high-energy physics through geosciences and engineering to biology and medicine, are challenging both our technical approaches and our social and economic structures. One need look no further than high-throughput genetic sequencers, the Large Hadron Collider, and whole sky astronomy surveys to see the challenges and the opportunities.
The challenges to technical approaches are self-evident; any shift by orders of magnitude inevitably brings change, and we need new tools and techniques to extract insights from the data tsunami. As the late Herb Simon once remarked, ". . . a wealth of information creates a poverty of attention and a need to allocate that attention efficiently among the overabundance of information sources that might consume it."
The social and economic challenges are just as difficult, though much less discussed. Two issues merit special attention, the culture of sharing, within and across disciplines, and the economics of research data sustainability.
Data Sharing
An old joke defines data mining as (insert possessive gesture here) data are mine. Sadly, this hoary saw is more often truthful than humorous. Historically, competitive research advantage accrued to those individuals and groups who first conducted the experiments and captured new data, for they could ask and then answer questions before others. The rise of large-scale, shared instrumentation is necessitating new models of sharing and collaboration across disciplines and research cultures. When many groups have access to the same data, advantage shifts to those who can ask and answer better questions.
The rising importance of data fusion across disciplines brings a deeper issue than simple sharing. Often, data proves to be most valuable in disciplines and groups other than the ones where it was first captured. Social network data illuminates the spread of disease; geosciences data guide urban planning; and atmospheric measurements reveal the health effects of effluents. All of these sometimes unexpected uses have timelines and utility extending far beyond the specific research projects and groups that produced the data. The question then becomes how we maintain this data and cross the cultural boundaries needed to make it accessible to others, particularly when the timescales for initial research data creation and later use by other disciplines may differ by decades.
Data Sustainability
The default reaction to the question of data sustainability is often to propose retaining everything. After all, device storage capacities continue to grow rapidly. However, like an iceberg, the raw cost of storage is simply the small and most obviously visible portion of total cost of data ownership. The majority of the cost lurks beneath — metadata management and creation, access systems and security, curation and coordination — and some entity must bear these costs for sustainability. More pointedly, rarely do the creators of the data have either the technical skills or the incentives to maintain data for long periods. At a higher level, research agencies and universities now face fiscal exigencies that further exacerbate the financial strain of research data sustainability.
Even in the most financially opportune times, not everything can or should be saved. The challenge is in creating economic and social models that extract a larger measure of research and economic value from the data, providing subsidies for data sustainability and further research. Equally importantly, such models could provide the backdrop for choosing which data to retain and which to discard. Lest this seem a Luddite perspective, remember that librarians and archivists have been triaging materials for thousands of years.
Simply put, we must find a new way forward that defines the principles and processes for protecting intellectual property while also creating appropriate cultural and economic rewards for data sharing and sustainability. This is a challenge facing not just individual disciplines, but society at large. We must work together to find a solution.
Join the Discussion (0)
Become a Member or Sign In to Post a Comment