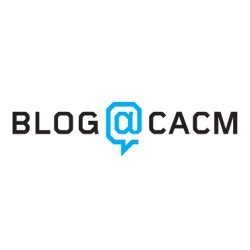
http://cacm.acm.org/blogs/blog-cacm/155468-what-does-big-data-mean/fulltext
September 21, 2012
It is interesting to note that a substantial subset of the computer science community has redefined their research agenda to fit under the marketing banner of "Big Data." As such, it is clearly the "buzzword du jour." As somebody who has been working on database problems for a very long time (which, by definition, deal with big data), I would like to explain what I think "big data" means, and discuss what I see as the research agenda.
In the community I travel in, big data can mean one of four things:
Big volumes of data, but "small analytics." Here the idea is to support SQL on very large datasets. Nobody runs "Select*" from something big, as this would overwhelm the recipient with terabytes of data. Instead, the focus is on running SQL analytics (count, sum, max, min, and avg with an optional group_by) on large amounts of data. I term this "small analytics" to distinguish this use case from the one that follows.
Big analytics on big volumes of data. By big analytics, I mean data clustering, regressions, machine learning, and other much more complex analytics on very large amounts of data. At the present time, users tend to run big analytics using statistical packages, such as R, SPSS and SAS. Alternately, they use linear algebra packages such as ScalaPack or Arpack. Lastly, there is a fair amount of custom code (roll your own) used here.
Big velocity. By this I mean being able to absorb and process a fire hose of incoming data for applications like electronic trading, real-time ad placement on Web pages, real-time customer targeting, and mobile social networking. This use case is most prevalent in large Web properties and on Wall Street, both of whom tend to roll their own.
Big variety. Many enterprises are faced with integrating a larger and larger number of data sources with diverse data (spreadsheets, Web sources, XML, traditional DBMSs). Many enterprises view this as their number-one headache. Historically, the extract, transform, and load (ETL) vendors serviced this market on modest numbers of data sources.
In summary, big data can mean big volume, big velocity, or big variety. In the remainder of this post, I talk about small analytics on big volumes of data.
Big Volume, Small Analytics
I am aware of more than five multi-petabyte data warehouses in production use running on three different commercial products. No doubt there are a couple of dozen more. All are running on "shared nothing" server farms with north of 100 usually "beefy" nodes, survive hardware node failures through failover to a backup replica, and perform a workload consisting of SQL analytics as defined previously. All report operational challenges in keeping a large configuration running, and would like new DBMS features. Number one on everybody’s list is resource elasticity (i.e., add 50 more servers to a system of 100 servers, automatically repartitioning the data to include the extra servers, all without taking down time and without interrupting query processing). In addition, better resource management is also a common request. Here, multiple cost centers are sharing a common resource, and everybody wants to get their fair share. The pundits—for example, Curt Monash—often identify some of these data warehouses.
A second solution to this use case appears to be Hive/Hadoop. I know of a couple of multi-petabyte repositories using this technology, most notably Facebook. Again, there are probably a couple of dozen more, and I know of many IT shops that are prototyping this solution. There have been quite a few papers in the recent literature documenting the inefficiency of Hadoop, compared to parallel DBMSs. In general, you should expect at least an order of magnitude performance difference. This will translate into an order of magnitude worse response time on the same amount of hardware, or an order of magnitude more hardware to achieve the same performance. If the latter course is chosen, this is a decision to buy a lot of iron and use a lot of power. As detailed in my previous blog post with Jeremy Kepner, I am not a big fan of this solution.
In addition, Google and other large Web properties appear to be running large configurations with this sort of workload on home-brew software. Some of it looks much like commercial RDBMSs (e.g., F1) and some of it looks quite different (e.g., BigTable).
Off into the future, I see the main challenge in this world to be 100% uptime (i.e., never go down, no matter what). Of course, this is a challenging "ops" problem. In addition, this will require the installation of new hardware, the installation of patches, and the next iteration of a vendor’s software, without ever taking down time. Harder still is schema migration without incurring downtime.
In addition, I predict the SQL vendors will all move to column stores, because they are wildly faster than row stores. In effect, all row store vendors will have to transition their products to column stores over time to be competitive. This will likely be a migration challenge to some of the legacy vendors.
Lastly, there is a major opportunity in this space for advanced storage ideas, including compression and encryption. Sampling to cut down query costs is also of interest.
Judy Robertson "On the Pleasures of Teaching Computer Science Students"
http://cacm.acm.org/blogs/blog-cacm/164619-on-the-pleasures-of-teaching-computer-science-students/fulltext
May 23, 2013
Yesterday was the most important day of my work calendar. We awarded degrees to 50 computer science students, thus fulfilling one of our main purposes as academics. I had the pleasure of telling some of the top students their marks in person. I’ve been doing this job for a good few years now, but I still can’t quite get used to the buzz I get when students hear that they have succeeded. Their faces are pictures of incredulity, joy, but mostly relief that they made it. I am proud to have played a small part in their learning journeys.
We often interview students as part of our selection process into the first year, at the start of their journey. That can be extremely revealing. It’s quite a daunting situation for some teenagers to find themselves in a room with an unknown adult and try to talk their way into a university course. From time to time, though, the young person’s sheer passion for computer science shines out through the shyness. One chap this year was carefully cultivating an air of teenage boredom until we stared talking about computer games development, when he revealed his awe and reverence of his game development heroes (who no doubt were bored teenagers themselves once). Another candidate, the first member of his family to apply to university, spoke fondly of how he put his first computer together with his granddad when he was eight years old. School students at our Turing birthday party last year were delighted to talk to our students about their programming projects, as they said their teachers didn’t understand what they were working on. I strongly remember one of our current Ph.D. students almost dancing with excitement when he got to talk with one of the professors about Open GL. He had been teaching himself for years but now he had someone else to talk to about his favorite topic. He had come home.
As academics, our role is to teach the foundations of computer science while fueling—rather than dampening—this passionate geekery. We try to fan the flames of geekery in those who have never had the good fortune to experience it before. It is hard for us to do this, and even harder for the students to keep motivated throughout the long journey to graduation. To get a CS degree at my university, you need to pass 32 different courses, picking up 480 credits on the way. On each of these 32 courses, there are possible ways to slip up: course work whose spec you cannot fathom, compilers that hate you, unit tests that spontaneously fail just before the deadline, exams in which your mind goes inexplicably blank. Many students also have the hurdles of young adulthood to deal with too—a potential mixture of financial hardship, leaving home, relationship break-ups, bereavement, or mental health difficulties.
In spite of all this, the students get through it. They learn where to put their semicolons. They grasp how Quicksort works. They sort out their matrices and master the halting problem. They fall in love with APIs and engrave comms protocols on their hearts. They learn how to write, how to present their ideas, how to think. This is a privilege to witness. Academics really do have the best job.
Join the Discussion (0)
Become a Member or Sign In to Post a Comment