Embodied Artificial Intelligence (EAI) integrates artificial intelligence into physical entities like robots, endowing them with the ability to perceive, learn from, and dynamically interact with their environment. This post serves as a brief review of the history of EAI, its current developments, as well as a discussion of its future outlook.
1. Early Foundations of EAI
The idea of EAI was first extensively explored in Rodney Brooks’s research paper “Intelligence without representation” published in 1991 [1], which proposed the radical view that intelligent behavior could arise directly from the simple physical interactions of an autonomous machine with its environment, without the need for complex algorithms or internal representations.
Then in 1999, Rolf Pfeifer and Christian Scheier wrote “Understanding Intelligence” to argue that intelligence is not confined to the brain or certain algorithms, but is a comprehensive manifestation of the entire bodily structure and function of an agent [2]. By this view, the authors opposed the traditional brain- or computation-centric understanding of intelligence, emphasizing the fundamental impact of the body on the formation of intelligence.
Starting from cognitive science, Linda Smith proposed the “embodiment hypothesis” in 2005, which emphasizes the core role of the body’s interaction with its environment in cognitive processes [3]. According to the embodiment hypothesis, our thinking, perception, and capabilities are formed through continuous interactions between our bodies and the physical environment. Particularly, the embodiment hypothesis emphasizes the essential role of the environment, considering it not only provides sensory inputs, but also participates in forming bodily behaviors and cognitive structures.
These foundational studies highlight three principles for developing EAI systems. First, EAI systems must not rely on predefined, complex logic to manage specific scenarios. Second, it is essential that EAI systems incorporate evolutionary learning mechanisms, enabling them to adapt continuously to their operational environments. Lastly, the environment plays a pivotal role in shaping not just physical behaviors, but also cognitive structures.
2. Recent Developments of EAI
Recent advancements in foundation models, such as large language models (LLMs), vision language models (VLMs), and the application of technologies such as ChatGPT in humanoids [4] have led to a common but incorrect belief that EAI is solely about having these foundation models performing inference tasks in robots to enhance the robots’ cognitive capabilities.
Foundation models like GPT-4, BERT, CLIP, and DALL-E enhance robots’ ability to interpret both visual and textual information, significantly improving their perception. These models allow robots to perform complex tasks by understanding context, objects, and instructions more akin to human interaction [5]. Also, these foundation models do satisfy principle one of EAI systems design, such that the inferences of these foundation models do not rely on predefined logic to manage specific scenarios.
However, these foundation models alone do not encapsulate the full spectrum of EAI system requirements. These models must be integrated with evolutionary learning frameworks to learn effectively from their physical interactions with open environments. In addition, we need to develop a virtual environment to efficiently interact with the EAI systems, as obtaining real-world interaction data is extremely costly and inefficient [6].
One demonstration of principle two is the development of the Deep Evolutionary Reinforcement Learning (DERL) framework [7], which allows for the exploration and testing of various agent morphologies in response to environmental challenges, significantly enhancing the morphological and behavioral adaptability of the agents. In addition, with new morphologies come new data to improve the foundation models’ capabilities to adapt to the new environment. Therefore, beyond just utilizing foundation models for inference, it is crucial to establish an effective feedback loop that facilitates continuous enhancements, enabling robots to adapt dynamically to their operating environments.
With foundation models to extend robots cognitive capabilities, and an evolutionary learning framework to adapt to new environments, a virtual environment that can effectively emulate the real world and interact with the EAI systems is imperative to satisfy principle three. One recent example is the Habitat platform [8], which facilitates the development of EAI by providing a highly efficient, photorealistic 3D simulation environment where virtual robots can be trained. Habitat has been demonstrated in improving EAI systems, specifically in tasks like point-goal navigation, where the platform’s ability to provide massive, scalable training environments can significantly enhance learning outcomes over traditional methods.
3. Future Outlook
By integrating the three components mentioned above, we can build a fully functional EAI system capable of dynamically adapting to different operating environments. The natural next step is to teach robots to understand the physical world, such as the concept of gravity. We believe teaching robots physical laws through data is the immediate barrier to the widespread adoption of robots in our daily lives.
Despite significant advances in AI and robotics, current robotic systems still lack a deep, intuitive understanding of the physical world. Research has shown that while robots can perform certain tasks or mimic certain aspects of human behavior, they don’t have true human-like understanding [9]. This problem can be addressed by generating accurate physical interaction data with the virtual environment and improving the foundation models with these interaction data through evolutionary learning mechanisms.
Several approaches have been explored to teach robots the laws of physics. One approach to address this problem is PLATO, which has been proposed to learn physics by watching simulated videos depicting objects interacting according to the laws of physics [10]. This system can distinguish between realistic and nonsensical scenarios, such as objects disappearing or behaving in impossible ways. By training PLATO with videos where objects follow predictable, physical laws, the AI learns to anticipate and understand basic physical concepts, which enhances its general reasoning about the physical world.
A second approach explores how generative neural networks can learn physical concepts and compares these learning trajectories with those of children [11]. The study evaluates two hypotheses regarding developmental processes: stochastic optimization and complexity increase. It finds that while neural networks can acquire a broad range of physical concepts, their sequence of learning these concepts does not align with the developmental trajectories observed in children. This discrepancy suggests that these models, despite their sophistication, do not entirely capture the nuanced ways in which humans develop physical understanding.
4. Conclusion
This post traces the evolution of EAI from its conceptual underpinnings to modern applications and future challenges. Particularly, we have highlighted three principles for developing EAI systems. First, EAI systems must not apply predefined, complex logic to manage specific scenarios. Second, EAI systems need to incorporate evolutionary learning mechanisms to continuously provide feedbacks. Third, a virtual environment that interacts with EAI systems is required for interaction data generation. Recent research advances have satisfied these three principles individually, but we have yet to see a fully functioning commercial system that incorporates all three components. When such a system is ready, an imminent challenge is to teach EAI systems to understand physical laws for them to operate smoothly in the physical world.
References
- Brooks, R.A., 1991. Intelligence without representation. Artificial intelligence, 47(1-3), pp.139-159.
- Pfeifer, R. and Scheier, C., 2001. Understanding intelligence. MIT press.
- Smith, L.B., 2005. Cognition as a dynamic system: Principles from embodiment. Developmental Review, 25(3-4), pp.278-298.
- OpenAI and Figure AI develop humanoid robot, BBC News, https://www.youtube.com/watch?v=cjVMQl9pVB0, accessed 4/23/2024
- Hu, Y., Xie, Q., Jain, V., Francis, J., Patrikar, J., Keetha, N., Kim, S., Xie, Y., Zhang, T., Zhao, Z. and Chong, Y.Q., 2023. Toward general-purpose robots via foundation models: A survey and meta-analysis. arXiv preprint arXiv:2312.08782
- Liu, S, The Value of Data in Embodied Artificial Intelligence, Communications, https://cacm.acm.org/blogcacm/the-value-of-data-in-embodied-artificial-intelligence/
- Gupta, A., Savarese, S., Ganguli, S. and Fei-Fei, L., 2021. Embodied intelligence via learning and evolution. Nature Communications, 12(1), p.5721.
- Savva, M., Kadian, A., Maksymets, O., Zhao, Y., Wijmans, E., Jain, B., Straub, J., Liu, J., Koltun, V., Malik, J. and Parikh, D., 2019. Habitat: A platform for embodied ai research. In Proceedings of the IEEE/CVF international conference on computer vision (pp. 9339-9347).
- Torresen, J., 2018. A review of future and ethical perspectives of robotics and AI. Frontiers in Robotics and AI, 4, p.75.
- Piloto, L.S., Weinstein, A., Battaglia, P. and Botvinick, M., 2022. Intuitive physics learning in a deep-learning model inspired by developmental psychology. Nature human behaviour, 6(9), pp.1257-1267.
- Buschoff, L.M.S., Schulz, E. and Binz, M., 2023, July. The acquisition of physical knowledge in generative neural networks. In International Conference on Machine Learning (pp. 30321-30341). PMLR.
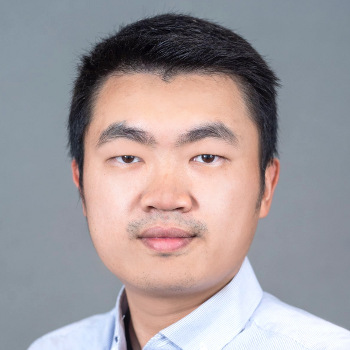
Shaoshan Liu is currently a member of the ACM U.S. Technology Policy Committee, and a member of the U.S. National Academy of Public Administration’s Technology Leadership Panel Advisory Group. His educational background includes a Ph.D. in Computer Engineering from U.C. Irvine, and a Master of Public Administration (MPA) from Harvard Kennedy School.
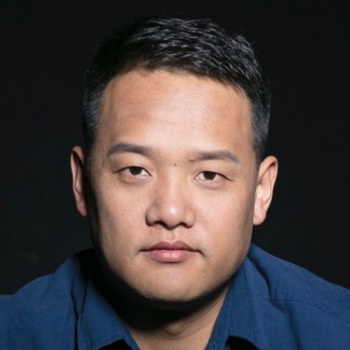
Shuang Wu is currently a Principal Scientist at Nvidia focusing on autonomous driving technologies. He has worked in computer vision, machine learning, and other areas of artificial intelligence for over two decades in both academic and industrial settings. His educational background includes a Ph.D. in Physics from USC and a B.S. in Physics from Peking University.
Join the Discussion (0)
Become a Member or Sign In to Post a Comment