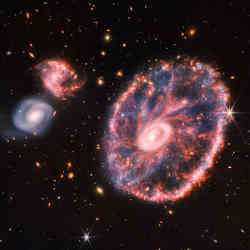
The July 12 release of the images from NASA's James Webb Space Telescope (JWST) has captivated and excited everyone from schoolchildren to space buffs, thanks to the vivid colors and crisp captures of the distant reaches of space. The images from the telescope, which is the largest, most complex and powerful space telescope ever constructed, brought into focus thousands of galaxies, both known and unknown, as well as so-called "cosmic cliffs" of dust and gas, and even a dying star.
The telescope detects near-infrared and mid-infrared wavelengths, the light beyond the red end of the visible spectrum, which allows otherwise hidden regions of space to be captured. Infrared light can uncover and reveal new details in images, based on the object. For example, bodies of matter such as young planets that are cool and do not emit much energy or visible brightness, still radiate in the infrared. Similarly, visible light's short wavelengths often can be obscured by space dust or a dense nebula (a group of interstellar clouds), keeping their images from being captured by telescopes that only detect visible light, such as the Hubble telescope. Infrared light, with its longer wavelengths, can penetrate through dust more easily, and infrared-based telescopes can detect lower-energy objects that often form within nebulae, such as brown dwarf stars and newly forming stars. Thus, the JWST can reveal objects that previously were hidden from view.
Although the images themselves are astounding, the real value for astronomers and scientists will come from the deep analysis of the objects contained in the images. While artificial intelligence (AI) can be used to determine which data are important to be sent for processing, thereby reducing the overall amount of information that needs to be analyzed and stored, the use of deep learning provides massive benefits in the processing and analysis of the data.
Deep learning is being used to identify and classify objects from the images and can provide a significant advantage over manual classification techniques. Using a supervised approach, a training set of previously identified objects and their specific attributes and features are fed into a system to "teach" the models to yield the desired outputs. For space object classification, the training dataset includes inputs and correct outputs to identify objects such as stars, galaxies, space dust and clouds, black holes, and other elements of interest, which allow the model to learn over time. The algorithm measures its accuracy through the loss function, adjusting until the error has been sufficiently minimized to ensure confidence.
Once the model has been developed, the algorithm is ready to process, analyze, and classify new objects found by telescopes such as the JWST, saving significant amounts of time and effort over manual analysis.
"Without AI tools to perform classification, objects in astronomical images have to be inspected by professional or amateur astronomers, with a classification determined by weighting the opinions of people," says Brant Robertson, a professor of astronomy in the astrophysics department at the University of California Santa Cruz (UCSC), who is involved in the process of analyzing recently captured JWST images. "The speed of visual inspection by humans is limited by how quickly the information can be provided and by how many people can provide useful inspections of many objects. [However,] the speed of AI classification is only limited by the amount of computing available, which is no longer a considerable limitation, and the careful preparation of the datasets."
Morpheus, a deep learning framework based on TensorFlow (an end-to-end open-source platform for machine learning), will be used to perform image classification on the data captured by the JWST. Originally developed in 2019, Morpheus is a model for generating pixel-level structural classifications of astronomical data sources. It leverages deep learning to perform source detection, source segmentation, and morphological classification on a pixel-by-pixel basis, using a semantic segmentation algorithm adopted from the field of computer vision. By using this structural data about the flux of real astronomical sources during object detection, Morpheus has demonstrated resiliency to false-positive identifications of sources, according to an evaluation using data captured by the Hubble Space Telescope.
"Deep learning models like Morpheus use the full pixel information in an image to perform classification, so all the visual features of galaxies or stars are used by the model," Robertson says. "Galaxies come in three broad categories: elliptical galaxies are ellipsoidal and relatively smooth; disk galaxies are usually flattened and have spiral structure or dark dust lanes, and irregular galaxies tend to be clumpy and amorphous. Since each of these objects have visually distinct morphologies, the model can tell them apart." Robertson says his team can "process the largest JWST surveys with Morpheus in just a few hours with the computational resources we have here at UCSC."
In a podcast conducted a few months prior to the release of the JWST images, Robertson said the telescope may be able to look for features in the atmospheres of planets that could indicate a presence of life. While Morpheus has not yet been trained to analyze this type of date, Robertson said, "We'd very much welcome collaboration from scientists interested in AI methods for evaluating JWST spectroscopic data of atmospheres."
Indeed, the study of space is a worldwide, collaborative effort, and other researchers also have developed AI platforms that can also be used to identify, evaluate, and classify objects found by space telescopes. RobERt (Robotic Exoplanet Recognition) is a deep neural network created by Ingo Waldmann and his team at the U.K.'s University College London, which used more than 85,000 simulated light curves from five classes of exoplanets to train RobERt to recognize the presence of specific molecules and gases in exoplanets' atmospheres. The platform was used to model exoplanet data from the Hubble Space Telescope, and after training, RobERt was able to identify molecules such as water, carbon dioxide, ammonia, and titanium oxide in light curves from real exoplanets with 99.7% accuracy.
Keith Kirkpatrick is principal of 4K Research & Consulting, LLC, based in New York, NY, USA.
Join the Discussion (0)
Become a Member or Sign In to Post a Comment