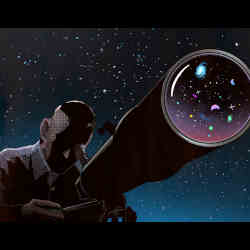
Back in 1977, astronomer Jerry Ehman circled an anomaly on a printout of narrowband radio signal data recorded by Ohio State University's Big Ear telescope as it swept the skies for signs of extraterrestrial life. Alongside, Ehman wrote one now-famous word "Wow!"
We are still scouring the heavens for evidence that may answer the enduring question: are we alone in the universe? We don't yet know, but artificial intelligence (AI) is now supporting our investigations.
A number of methods have been deployed in the search for extraterrestrial life. They include in situ observations, like sending a rover to Mars to search for evidence of current or historical life, as well as remote sensing, like probing distant planetary atmospheres using technologies such as the future James Webb Space Telescope (JWST), and searching for signs of extraterrestrial technologies and communications.
AI is being used to support each of these research avenues.
Looking for planets that have similar life-supporting conditions to Earth, such as the presence of liquid water, is a vital part of the search. Exoplanets, or planets that orbit stars beyond our solar system, are a primary target. Explains Greg Olmschenk, a machine learning specialist at the U.S. National Aeronautics and Space Administration (NASA) Goddard Institute for Space Sciences, "Our best bet right now for finding life elsewhere is to look for life as we know it, because that's the only kind of life that we know."
NASA missions, such the Transiting Exoplanet Survey Satellite (TESS) and the now-retired Kepler space telescope, survey the skies looking for exoplanets. Tiny dips in a star's perceived brightness can reveal the presence of an exoplanet as it transits, or crosses in front of, the star. These dips can be detected in light curves, or measurements of a star's light over time. TESS data, for example, contains around 60 million such light curves, which helps to explain the incorporation of AI into the process.
"It's not practical to have an astrophysicist look at each one of those and so we trained a neural network to do that," Olmschenk said.
To accomplish that, Olmschenk collaborated with researchers from the Universities Space Research Association, the Catholic University of America, the University of Maryland, and Science Systems and Applications, Inc. in the U.S., and the Università degli Studi di Napoli Federico II in Italy. They developed a one-dimensional (1D) convolutional neural network (CNN) to identify planetary transit signals. Olmschneck explained that while 2D convolutional networks are more common, as most neural networks are looking at images, "In this case, what we're looking at is one-dimensional time data."
Olmschenk said the CNN was trained to dismiss false positives, dips caused by other types of signals, such as the eclipsing of binary stars. It has a stack of convolutional layers that process input data, with each layer looking for certain patterns, such as light curves going up or down.
"You'll start to get pieces of the neural network that are recognizing peaks and troughs in the light curve, and eventually when you get down to the lower layers, you start to recognize things like actual transit dips."
The process filtered millions of light curves down to a few thousand that looked most likely to be planets. Astrophysicists then carried out follow-up observations to produce a short list of exoplanet candidates.
Sophisticated techniques such as radial velocity measurement, which detects the tell-tale 'wobble' produced by the gravitational tug of a planet on a star, ultimately determine whether candidates are truly planets. At the time of writing, 183 such candidates are still being investigated.
Searching for Signs of Intelligence
At the SETI Institute in Mountain View, CA, researchers have been looking for evidence of life on other worlds since 1985. The non-profit research organization was born from earlier SETI (search for extraterrestrial intelligence) projects funded by NASA.
SETI Institute CEO Bill Diamond draws a distinction between 'life' and 'intelligent life' on other worlds. While there may be biological life elsewhere in our solar system, he says, "We're talking about intelligent and technological beings that might exist on planets outside of our own solar system."
The Institute has close links with the nearby NASA Ames Research Center, and its researchers developed AI for NASA'S Kepler and TESS missions.
The search for other-worldly intelligence includes looking for evidence of alien technologies—which might include radio signals, megastructures, or laser pulses. Sweeping the skies for alien transmissions, as Big Ear did back in 1977, remains a go-to SETI technique.
SETI Institute scientists use algorithms, including fast Fourier transforms (FFT), to analyze millions of signals captured by the Allen Telescope Array (ATA) in order to identify anomalous signals that can be flagged for further investigation. The captured data includes wide-band signals from distant exoplanets, such as the TRAPPIST-1 planetary system identified in 2017 as including the most Earth-sized planets found in the habitable zone of a single star.
"We can use machine learning and AI to do anomaly detection with greater speed, with greater sensitivity," said Diamond.
The search goes on
AI, and machine learning in particular, will play a vital role in the ongoing search for extraterrestrial life, said Olmschneck. "It's something that can learn for whatever task you're trying to accomplish, as long as you have enough data to have the methods learn what they need to do."
In our own solar system, AI will aid the analysis of soil sample data when the 2022 ExoMars mission sets out to look for signs of life on Mars. In deeper space, it will support the James Webb Space Telescope (JWST), an orbiting infrared observatory to be launched in the near future to perform spectroscopy on exoplanet atmospheres. Planetary atmospheres may harbor evidence of life, including biosignatures and anomalies like the presence of chemicals with no natural explanation. Spectroscopy on the atmospheres of distant planets may reveal kinds of spectra that humans have never considered, Olmschneck said.
"Having a neural network that can learn the different kinds, and then be able to learn different combinations of them, allows it to have the potential to detect those — even if you wouldn't have been able to do such a thing."
The limitations of human perception could be the greatest obstacle to determining whether or not we are alone in the universe. It may take an artificial intelligence to finally find an extraterrestrial intelligence.
Karen Emslie is a location-independent freelance journalist and essayist.
Join the Discussion (0)
Become a Member or Sign In to Post a Comment