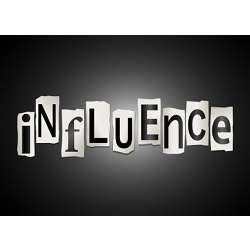
Academic search engines and indices are valuable tools for assessing the frequency of being published and cited in academic journals. However, some of these tools do not provide a comprehensive way to truly measure whether a researcher is influencing others with his or her work, and advancing the field with new ideas and approaches.
For example, Google Scholar is a free tool that crawls for and indexes a wide swath of academic research papers, and provides an overall count on the number times a particular paper has been cited.
The h index is a metric that builds on the functionality of Google Scholar by discounting both people who have a large number of rarely cited papers, and people who have a few highly cited papers, both situations in which the overall, lasting influence of the author likely is limited.
As a result, Semantic Scholar on April 20 introduced three key metrics: Citation Velocity, Citation Acceleration, and Most Influential Citations. These metrics are calculated by a machine learning algorithm that de-emphasizes strict citation counts and focuses on metrics that look at the context, recency, and rate of citations to better determine the level of influence.
“It really starts with our philosophy, which is different than Google Scholar,” says Oren Etzioni, CEO of the Allen Institute for Artificial Intelligence, developer of Semantic Scholar. “It’s important to be selective.”
Citation Velocity is the average rate at which an author’s papers have been cited in recent years, excluding self-citations. Meanwhile, Citation Acceleration refers to the change in citation velocity in recent years, which can illustrate whether a paper’s citation rate is increasing, decreasing, or remaining static. Each of these metrics can be useful in determining whether an author’s work is gaining or losing influence.
According to Etzioni, the value in this service is to help academics quickly ascertain a researcher’s true level of influence in the computer science field, without having to read through hundreds of papers, or simply guessing which papers or authors are influential.
“Not all papers were created equal, and not all citations were created equal,” Etzioni says, noting the algorithm automatically excludes self-citations, which can drive up citation counts but do not illustrate any influence on other research, and looks at the context of citations to determine influence. This results in the Most Influential Citation metric, which incorporates the machine learning algorithm to assess whether the cited work is used or extended in the new work or paper, based on the number of times a reference is mentioned in the body of the paper, as well as the surrounding context for each citation.
“Many people include what we would call ‘throwaway citations,’ where you’re mentioning things for completeness,” Etzioni says. “When we analyze the relationships between a paper and what may be 700 papers that cite it, we’ll explicitly identify the subset that is influential, and often that’s a reduction of a factor of 10 or more. So that would mean that out of 700 papers, maybe 20 are actually influential.”
The results of these outputs are manifest in the Author Profiles. Displayed with a target author in the center of the Influence screen, they show authors that have influenced the target author on the left, and authors that have been most influenced by that target author on the right. The profiles also contain drill-downs that display the ranking of papers, authors, and departments. These profiles are built directly from the machine learning algorithm, and cannot be influenced by authors or any other outside forces.
An Author Profile on Semantic Scholar.
Still, when it comes to evaluating potential candidates to be hired or promoted, simply looking at one’s publishing history is just one part of a larger assessment process, according to Lance Fortnow, chair of the School of Computer Science in the Georgia Institute of Technology College of Computing.
“Where the papers are published, particularly in the major conferences, also makes a difference,” Fortnow says. “But I want to emphasize that’s only a small part of how we judge candidates. What I truly look for is leadership in the field, is the candidate creating research ideas that lead the field, as opposed to just extending other people’s work. For that, you need to look at the work itself, as well as the recommendation letters.”
Fortnow also says he follows the recommendations of the recent Computing Research Association Best Practice Memos, which say candidates should upload a single paper for evaluation, rather than submitting a spate of papers or a list of citations.
Keith Kirkpatrick is principal of 4K Research & Consulting, LLC, based in Lynbrook, NY.
Join the Discussion (0)
Become a Member or Sign In to Post a Comment