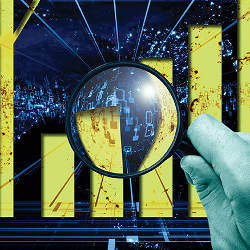
“Our view of reality is conditioned by our position in space and time—not by our personalities as we like to think. Thus every interpretation of reality is based upon a unique position. Two paces east or west and the whole picture is changed.”
—Lawrence Durrell, Balthazaar10
“…to be human is to be ‘in place’.”
—Tim Cresswell, Place: A Short Introduction7
On April 20, 2011, U.K. researchers Alasdair Allan and Peter Warden caused a media frenzy by announcing their discovery of an iPhone file—consolidated.dba—that contained time-stamped user-location data.4 A FAQ published by Apple3 and congressional testimony by Apple’s vice president for software technology26 subsequently revealed that at least some of the initial concerns were groundless. Assuming Apple’s anonymity-preservation techniques are adequate, Apple does not compile location traces for individual users, instead enlisting those users as data collectors in a worldwide exercise in crowdsourcing. Apple is creating a highly precise map of cell sites and access points in an effort to improve the speed and accuracy of its user-location estimates, thus providing more-refined location-based services. However, despite Apple’s quick and thorough response, long-term issues remain.
Key Insights
- The precision of cellular-location estimates means service providers are able to obtain location estimates with address-level precision, creating a serious privacy problem, as the estimates can be highly revealing of user behavior, preferences, and beliefs.
- Supposedly anonymous location traces can be de-anonymized through correlation with publicly available databases.
- Privacy-aware design makes it possible to retain the full benefit of LBS while preventing accumulation of address-level location traces for a given individual and reducing the potential for de-anonymization.
This article explores the evolution of location-based services (LBS), culminating in Apple’s and Google’s use of crowdsourced data to create a system for obtaining location fixes potentially faster and more accurate than the global positioning system (GPS). This article also develops an intuitive sense of the potentially revelatory power of fine-grain location data, then addresses the question of potential harm. The most obvious concern is the stalker, while others involve manipulation and threats to autonomy. Also provided is a brief review of the philosophy of place, focusing on the ability of location-based advertising (LBA) to disrupt individuals’ relationships with their surroundings. It then turns to the potential for anonymous LBS, with the aim of saving the benefit while avoiding potential harm. Finally, it asks: How much location data must a marketer acquire before a correlation attack can de-anonymize the data?, answering through the Shannon-theoretic concept of “unicity” distance and recommending ground rules for development of truly anonymous LBS.
Technology of Place
Cellular telephony has always been a surveillance technology. As discussed by the author,27 cellular networks are designed to track a phone’s location so incoming calls are routed to the most appropriate cell tower, usually the one closest to the user. As most users are aware, recent generations of cellphones are capable of much more fine-grain location resolution. The first step toward adding this capability came with E911, the Federal Communications Commission’s 1996 effort to enhance location resolution for cellular 911 calls.b E911 established a requirement that cellular service providers send location information to the Public Safety Answering Point when subscribers make 911 calls with their cellphones. The intuition underlying E911 was clear: It would be desirable for emergency services to be able to locate a victim without searching the entire coverage area of a cell site. However, the technological and sociological impact has far outstripped this intuition over the past 16+ years.
One of the more immediate consequences of E911 is that many cellular handsets now have some form of GPS capability, whether standalone or network-assisted.29 With it, service providers increasingly recognize that a much broader (and more lucrative) range of location-based services could be provided. However, it should be understood that GPS was not designed with cellphones in mind.c GPS was intended for outdoor use; the weak signals transmitted from the 24 space vehicles (SVs) that constitute the GPS space segment are difficult to detect indoors and blocked by tall buildings.16 GPS is also designed to work with autonomous receivers; GPS signals are modulated to provide the receiving unit with the locations and orbits of the SVs, information needed to compute the receiver’s location.d The locations and orbits are provided on the same carrier used for (civilian) distance estimation. In order to avoid interference, the data rate for these transmissions is slow—only 50bps—so a receiver takes up to 12.5 minutes to obtain all the information it needs to perform a location fix. Networks often assist cellphones by providing this information over much-faster cellular links,9 but cellphone manufacturers are apparently looking to other means for quick, accurate location fixes for their subscribers.
This brings us to the April 2011 kerfuffle over Apple’s and Google’s use of cellphones to identify Wi-Fi and cell-tower locations. In testimony before the U.S. Congress’s Judiciary Committee’s Subcommittee on Privacy, Technology and the Law, Guy Tribble, Apple’s vice president for software technology, confirmed what analysts of the consolidated.db file had already determined: Apple iPhones record the MAC address and signal strengthe for detected access points, then time-stamp and geo-tag that data. The geo-tag consists of a GPS/cell-tower-derived location estimate of the iPhone that has detected the access point. For detected cell sites, the cell-tower ID and-signal strength are combined with the detecting iPhone’s location estimate.
Tribble provided little technical detail but did suggest that by obtaining such data from a large number of iPhones (crowdsourcing), highly accurate estimates of the location of sites and access points could be determined. With a map of these locations, precise location estimates can be generated for phones that report receiving signals from the cell sites and access points.
A simple analysis makes the point. Consider a data set of n records for a single access point, with each record consisting of the location of a different receiving unit and the strength with which that unit receives the signal from the access point. The location of the access point can be computed by determining the weighted centroid of the measurements.5 Following creation of a map of the locations of cell sites and access points, a position fix for a cellphone can be computed through trilateration using received signal-strength measurements.
Trilateration is similar to what is performed by GPS receivers, with the added benefit that the distances are much shorter and the access points and cell towers are not moving. Overall, one would expect the resulting location estimates to be at least as good as a GPS fix in urban and residential areas and could be of sufficiently fine granularity as to be able to resolve an individual address.
The presence of consolidated.db in iPhones (a database of time-stamped GPS fixes for the cellphone) gives the appearance that Apple is tracking iPhone users, but Tribble said the “data is extracted from the database, encrypted, and transmitted—anonymously—to Apple over a Wi-Fi connection every 12 hours (or later if the device does not have Wi-Fi access at that time).”
The extent the data is anonymous is questionable without further detail. The author generated the figure here using the consolidated.db database on his iPhone and the iPhone Tracker application developed by Pete Warden.f His well-traveled path from Ithaca, NY, to Washington, D.C. (National Science Foundation and Defense Advanced Research Projects Agency) and onward to his parent’s house in Virginia Beach, VA, is apparent for all to see. It would take little effort to associate this trace with the author. As the Netflix example covered later suggests, there is more to anonymization than stripping a location trace of its associated phone number and user-account ID.
Personality of Place
The iPhone location trace says a lot about the author, including his predilection for visiting Washington, D.C., New York, and his parents. What would a more fine-grain set of tracking data, like that potentially being made available to LBS by emerging smartphone-location technology, have to say about an individual? Consider the following information, which can be derived through the correlation of fine-grain location data with publicly available information:
Location of your home. What kind of neighborhood do you live in? What is your address? Mortgage balances and tax levies are often available once an address is known. Your socioeconomic status can be deduced;
Location of your friends’ homes. What sort of homes do they have? Do you ever spend the night? How often?;
Location of any building you frequent with a religious affiliation. Or do you never frequent such buildings? In either case, beliefs can be deduced;
Locations of the stores you frequent. Your shopping patterns reflect your preferences and in some cases your beliefs or vices;
Locations of doctors and hospitals you visit. Do you visit frequently? How long do you stay? The fact that you have a serious illness is readily determined, as are, in some cases, even the type of illness through your visits to specialty clinics. Such information is of interest to insurance providers and the marketers of pharmaceuticals, among others; and
Locations of your entertainment venues. Do you attend the local symphony? Do your tastes run to grunge rock? Do you frequent bars? What type? One can draw multiple conclusions from the frequency of visits and types of venue.
One could go on with this list. The fact is fine-grain location information can be used to determine a great deal about an individual’s beliefs, preferences, and behavior. Databases containing such information pose a threat to individual security and privacy, as they can be a focus for hackers with criminal intent. On a less-malevolent note, such data is immensely valuable to direct-marketing firms. Entire businesses are built around the compilation of lists of such information acquired through other means.
The continued accumulation of location data may reach a point where a marketer can uniquely match an anonymous location trace to a named record in a separate database.
Is this a problem? Isn’t LBS data collection simply additional, perhaps redundant, data collection that feeds the ordinary and tedious process of direct marketing? The following sections show this is not the case. LBS supports location-based advertising (LBA), which has the potential for exerting substantially more power over individual behavior than previous modes of advertising.
The work of advertising. As of early 2012, InfoUSA maintained a list of 210 million U.S. consumers for sorting into various categories, including area code, ZIP code, home value/home ownership, housing type, mortgage, personal finance, hobbies and interests, children/grandparents/veterans, ethnicity, religion, and voter information.g As seen from the earlier thought experiment, much of it can now be derived by correlating location data with address databases. But data collection through location-based services takes the process of collection to a new level of invasiveness while adding an additional control variable to the process of advertising.
To begin, LBS data collection is able to substantially refine the personal information available from other sources; for example, one may claim to practice yoga, but marketers may now know how frequently one takes classes, pointing to a specific level of enthusiasm previously known only to individuals and their fellow yogis and yoginis.h LBS also enables an approach to consumer targeting that goes well beyond previous marketing strategies by collecting information about beliefs, preferences, and behavior while one performs the illustrative practice. A mailing list may indicate one is generally a lover of Italian food, while an LBS may have the additional knowledge that you are currently in an Italian restaurant. To understand why this is important, first consider the “work” that advertising has us do on its behalf.
In her 1978 book on the psychology of advertising, Judith Williamson described advertising as shifting meaning from one semantic network to another.28 As the book was originally written in the 1970s, Williamson focused on print advertising, with an occasional reference to broadcast television. In a canonical example she pointed to an advertisement that seems simple, a photograph of the iconic French actress Catherine Deneuve juxtaposed with a bottle of perfume (Chanel No. 5), encouraging us to bind to the perfume the association of class and beauty people of a certain age might associate with Catherine Deneuve. Meaning has been shifted from one semantic network (the realm of actresses) to another (a brand of perfume).
LBA has the potential to perform a similar sleight-of-mind, causing us to exchange the meaning we associate with a place for one suggested by an ad. Moreover, this location-based semantic shift is taking place through ads delivered to a device that can track the individual. This raises two new privacy issues: The first is that LBA has the potential to be a feedback system with dynamic control. The advertiser can present an ad when one is near a target location, then track that person to determine whether the ad has had the desired response. In the language of Gilles Deleuze,8 the advertiser can observe the response to the information stream presented to the individual, then “modulate,” or refine, that stream over time, driving the individual to a desired state of behavior; in this case, movement to and consumption at the target location. Primitive examples of modulation fueled by click-tracking can be seen by an aware observer of the Web. If one fails to produce the desired response to a pop-up window, other windows offer alternatives on behalf of the advertiser. Second, unlike click-tracking, LBA exploits consumers’ physical location, attempting to manipulate their relationship to their physical surroundings. The following highlights the potential for a more insidious form of manipulation at an entirely new level of psychological conditioning.
Philosophy of place. Many people view geography as the study of locations and facts; for example, “Jackson is the capital of Mississippi” is the stuff of geography, as is the shape and size of the Arabian Peninsula. However, in the 1970s, humanistic geographers began to move the field toward a consideration of “place” as more than a space or location, beyond latitude, longitude, and spatial extent.7 In an oft-quoted definition, the geographer and political philosopher John Agnew defined place as consisting of three things1:
Location. “Where,” as defined by, say, latitude and longitude;
Locale, or the shape of the space. Shape may include defining boundaries (such as walls, fences, and prominent geographical features like rivers and trees); and
Sense. One’s personal and emotional connections established through location and locale.
Place is thus a location to which one ascribes meaning. The process by which meaning attaches to place, and the importance of this process to the individual and to society, have become a prime focus for humanistic geographers. One aspect of it builds on the work of the phenomenologists. Phenomenology, generally associated with the German philosopher Franz Brentano and the Austrian philosopher Edmund Husserl, studies the structures of consciousness. Phenomenology proceeds by first bracketing-out our assumptions of an outside world, then focusing on our experience of the world through our perception. Phenomenologists study consciousness by focusing on human perception of phenomena, hence the name.i
The more that can be done within the handset and kept within the handset, the greater the preservation of anonymity.
Brentano is credited with one of the key results of the phenomenologist approach. In his 1874 book Psychology from an Empirical Standpoint,j he said one of the main differences between mental and physical phenomena is the former has intentionality; that is, it is about, or directed at, an object, or one cannot be conscious without being conscious of something. In the latter part of the 20th century, humanistic geographers took this philosophy a step further; in his 1976 book Place and Placelessness, Edward Relph asserted that consciousness could only be about something in its place, making place “profound centers of human existence.”23
Another thread in the philosophy of place originates with 20th century German philosopher Martin Heidegger who described human existence in terms of dasein, a German word that can be translated as “human existence,” or perhaps more helpfully as “being there.” The important thing for us here is to understand that dasein is always in the world.k As humans we enter a preexisting world of things and other people and develop our sense of self by (and only by) interacting with them. According to Heidegger, an in-authentic existence is one in which the individual fails to distinguish him or herself from the surrounding crowd and its priorities.
Humanistic geographers have taken up the concept of dasein, using it to explore the role of place in human existence. In his 2007 book Place and Experience: A Philosophical Topography, Jeff Malpas invoked dasein and related concepts of spaciality and agency to show that place is primary to the construction of meaning and society.l,19
Using these concepts, this article now aims to characterize the potential impact of LBA, the objective of which is to alter the ever-present, ongoing human process of interaction with the immediate surroundings. LBA attempts to shift intentionality, diverting consciousness from an experience of the immediate surroundings to the consumption of advertised goods. In Heideggerian terms, LBA interferes directly with the individual’s project of crafting an authentic existence.
Consider the following situation, developed in two stages: A family is seated at their dining room table enjoying dinner together, but there is an exception—the father, a relentless worker, is reading texts and email messages instead of joining the conversation. One could say he is no longer present. He has left the place. Or to turn it around, as far as the father is concerned, the dinner table is no longer a “place” with familial meaning but merely a location for eating. Now, to complete the example, assume that someone who wants to communicate with the father from afar knows when he is at the table and chooses that time to send texts. The texter now has the ability to disrupt the father’s relationship with the family dinner, a relationship often filled with a strong, even defining, sense of meaning.
The dinner table is a natural example for the author,m but one might consider a walk through one’s hometown, visiting an old high school, or attending a play. LBA has the potential to detract from the experience of these familiar and meaning-filled environs. One’s surroundings may thus lose their “placeness” through LBA, including their meaning, and become merely a path to be traversed. As places become locations, meaning is lost to the individual. That is, we lose some of ourselves, as well as one of the critical processes through which we become a self.
Location Anonymity
Having established the importance of location privacy, is it necessary to for-go the benefits of LBS and LBA? Fortunately the answer is no, but it needs to be clear to data collectors that it is not sufficient to simply scrub names and phone numbers from location traces. As AOL15 and Netflix21 have learned, supposedly anonymous datasets are often susceptible to correlation attacks in which datasets are associated with individuals through comparison of the datasets to previously collected data. Netflix is particularly instructive; in 2006 it issued a public challenge to develop a better movie-recommendation system.22 As part of the challenge, it released training data consisting “of more than 100 million ratings from over 480,000 randomly chosen, anonymous customers on nearly 18 thousand movie titles.” Within weeks, computer scientists Arvind Narayanan and Vitaly Shmatikov had showed the data was not as anonymous as Netflix might have thought. Narayanan and Shmatikov devised an elegant algorithm that correlated the NetFlix data with other publicly available data and thus identified a number of users in the Netflix training data.21 Along the way, they developed rules of thumb for such correlation attacks, noting such attacks work well when they emphasize rare attributes and that the winning match should have a much higher score than the second-place match. The first can be understood intuitively; a marketer would learn more from the knowledge that someone has purchased the author’s most recent text on error-control coding than from finding that someone has purchased a Harry Potter book. The second rule is equally intuitive, as it is intended to avoid false positives.
Here, these rules are useful for developing a Shannon-theoretic model for correlation attacks on supposedly anonymized location traces. In his 1949 paper “Communication Theory of Secrecy Systems,”24 Claude Shannon defined unicity distance as the minimum amount of ciphertext needed before uncertainty about a piece of plaintext could be reduced to zero. The translation to the de-anonymization of location traces is clear; the continued accumulation of location data may reach a point where a marketer can uniquely match an anonymous location trace to a named record in a separate database.
The goal in this article is not a specific number as a cutoff for data accumulation or an all-encompassing framework into which all de-anonymizing attacks have a place. Rather, it develops an example model and evaluates its dynamics—how the structure of the model changes as the amount of location data increases—in order to craft design rules for anonymous LBS.
A Shannon-theoretic approach to location anonymity. Let a marketing database S consist of a collection of binary preference vectors {Xi} of length n, where the index i indicates a specific user. The individual vectors have the form
Each coordinate xi,j is a binary indicator representing the user i‘s preference with regard to some specific item, belief, or behavior; for example, xi,0 might indicate whether the user likes cats (yes or no), and xi,1 might indicate feelings about dogs. Some preferences (such as the identity of the user’s favorite rugby team) might cover several coordinates, depending on the number of teams that can be represented in the database. If a given preference for a particular user is unknown, the associated coordinate is given the value “e” for erasure.n The marketer’s knowledge concerning a user’s beliefs, preferences, and behavior are thus coded into binary vectors of a fixed length (n) with a consistent semantic attribution to each coordinate or block of coordinates.
Now let Lm be a trace of length m, a sequence of m location fixes generated by a single subscriber
As discussed earlier, marketers can associate locations with beliefs and preferences, but the amount of information derived clearly varies depending on the type of location in the trace. Now consider a preference mapping F that maps location traces to preference vectors while acknowledging such mapping may not be one to one and is situation-dependent. The preference vectors have the same syntactic and semantic structure as the vectors in the marketer’s database
Narayanan and Shmatikov21 discussed several ways to identify the Xi
S that is the best match for a given P, thereby (potentially) de-anonymizing P. This article takes a somewhat different approach, attempting to characterize the dynamics of the de-anonymization problem as the length of the location trace grows.
Suppose a location trace of length m is mapped into a preference vector P of length n. P will have some t non-erased coordinates and n t erased coordinates. Assume that as m increases, t increases or remains the same.o This follows from the fact that as data collectors obtain more location information, they typically increase their knowledge about the associated individual.
Now consider those vectors in the marketing database for which individual preferences on these t coordinates are known. Within S there will be some Nm vectors with support for all t non-erased coordinates of P. These Nm vectors form a subset C ⊂ S. For each vector in C, delete all but the t coordinates of interest (those corresponding to the non-erased coordinates of P). We now have a set C‘ of Nm vectors of length t. The problem of de-anonymization now looks like an error-control coding problem, so which vector in C‘ provides the closest match to the non-erased coordinates of the preference vector P? The ability of the marketing database to distinguish between users can now be expressed (using coding-theoretic terminology) as the minimum distance between the vectors in C‘. The minimum distance is the minimum number of coordinates in which any pair of vectors differ. In more compact form, this can be expressed as
The greater the value of dmin, the greater the ability of a correlation attack to associate a location trace with a single record, and thus a single individual. When dmin is large, the individuals represented by the vectors in C‘ are readily distinguished from one another. On the other hand, if dmin is small or zero (as happens when two or more identical vectors are in C‘), then the problem of de-anonymization becomes difficult or even impossible. Marketers are unable to distinguish between the individuals so are unable to determine with which individual to associate a given location trace.
Privacy-aware system designers can now develop rules of thumb for preserving anonymity in the face of correlation attacks by exploring the dynamics of the relationship between location traces Lm, preference vectors P, and the minimum distance dmin of the corresponding set of vectors C’:
- As the length m of a location trace Lm increases, the number of non-erased coordinates of a preference vector P increases; the reverse is also the case;
- As the number of non-erased coordinates of P increases, the length of the vectors in C‘ increases, while the cardinality of C‘ decreases; fewer vectors in C will have the requisite support as the number of coordinates requiring support increases. The overall effect is an increase in minimum distance and a corresponding increase in the efficacy of correlation attacks;
- As the number of non-erased coordinates of P decreases, the length of the vectors in C‘ decreases while the cardinality of C‘ increases; more vectors in C have the requisite support, as less support is required. The overall effect is a decrease in minimum distance and in the efficacy of correlation attacks.
It follows both intuitively and analytically that the number of non-erased coordinates in P should be kept as small as possible and can be done in either of two ways:
Reduce the length of location traces. If the preference map has less information on which to operate, it generates a preference vector with more erased coordinates;
Reduce the ability of the preference mapping to resolve a location trace into specific coordinate values in a preference vector. This can be done by reducing or eliminating the extent each trace location provides preference-vector information.
System designers can exploit these results to design anonymity-preserving location-based services.
Anonymous LBS. Consider a basic location-based service; call it “The Doppio Detector,” giving users directions from their current location to the nearest espresso shop. For it to work, two basic types of information must be brought together: the subscriber’s location at an appropriate level of granularity and a geographic database containing the locations of all nearby espresso shops. With it, the server or the user’s handset can superimpose the user’s location onto a geographic database, then generate directions through a routing algorithm.
The structure of an LBS can thus be generalized as performing two basic functions:
- Determine subscriber location to the desired level of granularity; and
- Use a database to map the location to the desired information (such as directions to an espresso shop).
Separating these functions clarifies the anonymity problem while opening up the range of available anonymity-preserving techniques. We begin by determining subscriber location. The best means for preserving anonymity is to do an independent GPS fix on a cellphone. The handset may thus acquire an accurate location estimate without releasing any information to the outside world. This is a general theme; the more that can be done within the handset and kept within the handset, the greater the preservation of anonymity.
However, this approach can be slow. If the handset is to download all necessary SV location information from the SVs themselves, the user may have to wait as long as 12.5 minutes, a potentially excruciating delay when one needs caffeine. If the process is to be sped up through provision of constellation information by the cellular service provider, some location information must be leaked to that provider. However, such data can be coarse; the network must know only the cell site that is serving the user to provide the data for SVs that are potentially visible to the handset. Such coarse location information provides relatively little information about the user’s beliefs and preferences. Or to use the language of this article’s unicity distance analysis, the preference mapping F operating on cell-site information will produce a preference vector with a large number of erased coordinates.
Khoshgozaran and Shahabi17 suggested another approach to determining location anonymously: use the network to determine the location fix while preventing the network from knowing the subscriber’s actual location. The mobile device biases the data used for the location fix by applying a randomly selected transform to the mobile’s measurements. When the mobile receives the resulting location fix from the network, it removes the effects of the bias by adjusting the fix accordingly.
It follows from these options that obtaining a location fix of the desired granularity on the handset need not reduce the user’s location privacy. However, the second piece of LBS, the mapping function, creates two significant obstacles to maintaining privacy, with the second posing a potential personal security concern:
Consistent input granularity. The mapping function requires input granularity consistent with the inherent granularity of the query; a user who wants directions to the nearest espresso shop needs directions, beginning with a position with street-level resolution; and
Known location. Many if not most LBS queries involve objects of known, fixed location; for example, a bookstore has a known location and is generally not in motion. A request for directions indicates the requesting cellphone user will probably be at the location sometime soon.
Using access-point and cell-site location information, service providers are able to obtain location estimates with address-level precision.
The following paragraphs consider general means for accomplishing the mapping function while retaining a measure of anonymity:
A release of data is said to provide k-anonymity protection “…if the information for each person contained in the release cannot be distinguished from at least k1 individuals whose information also appears in the release.”25 It seems logical that such protection can be obtained for the LBS mapping function by stripping identifying information from k LBS requests, bundling and submiting them all at once. The LBS server then provides a combined response from which individual users are able to extract information responsive to their specific requests.
But who or what bundles the original k requests? Gruteser and Grunwald14 suggested a trusted server that bundles and forwards requests on behalf of users, while Ghinita et al.13 suggested a tamper-proof device on the frontend of an untrusted server that combines queries based on location. However, such approaches fall short of k-anonymity in that there may be side information (such as home location or a known place of business) that would allow the server to disaggregate one or more users from the bundled request. For example, I benefit little from a bundled request if the request includes my home as a starting point; my request is too easily disaggregated from the bundle.
Bear in mind that system designers need not completely eliminate the transfer of location information; it would be sufficient to reduce the precision of the location information to where the preference mapping gives the attacker or marketer little with which to work.p Given the decreasing cost of memory and bandwidth, it is both efficacious and inexpensive to simply blur the location estimate provided with the request for mapping functionality.q An LBS user may, for example, submit a request to the Doppio Detector that includes his or her location as “somewhere in downtown Ithaca,” rather than a specific address. The server will respond with a map that indicates the locations of all the espresso shops in downtown Ithaca. The user’s handset can then use its more precise knowledge of his or her location to determine the nearest espresso shop and generate directions accordingly.
Anonymity can also be preserved by limiting the length m of each location trace. This limitation is accomplished by preventing the LBS from determining which requests, if any, come from a given user.r As described in Wicker,27 public-key infrastructure and encrypted authorization messages can be used to authenticate users of a service without providing their actual identities. Random tags can be used to route responses back to anonymous users. Anonymity for frequent users of an LBS may thus be protected by associating each request with a different random tag. All users of the LBS thus enjoy a form of k-anonymity. Coupled with coarse location estimates or random location offsets, this approach shows great promise for preserving user anonymity while allowing users to enjoy the benefits of location-based services.
Conclusion
The increasing precision of cellular-location estimates is at a critical threshold; using access-point and cell-site location information, service providers are able to obtain location estimates with address-level precision. Compilation of these estimates creates a serious privacy problem, as it can be highly revealing of user behavior, preferences, and beliefs. The subsequent danger to user safety and autonomy is substantial.
To determine the extent to which location data can be anonymized, this article has explored the Shannon-theoretic concept of unicity distance to reveal the dynamics of correlation attacks through which existing data records are used to attribute individual identities to allegedly anonymous information. With this model in mind, it has also laid out rules of thumb for designing anonymous location-based services. Critical to them is maintenance of a coarse level of granularity for any location estimate available to service providers and the disassociation of repeated requests for location-based services to prevent construction of long-term location traces.
Acknowledgments
This work is funded in part by the National Science Foundation TRUST Science and Technology Center and the NSF Trustworthy Computing Program. I gratefully acknowledge the technical and editorial assistance of Sarah Wicker, Jeff Pool, Nathan Karst, Bhaskar Krishnamachari, Kaveri Chaudhry, and Surbhi Chaudhry.
Join the Discussion (0)
Become a Member or Sign In to Post a Comment