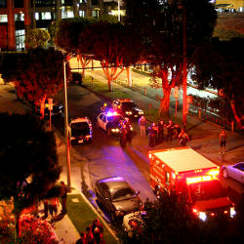
In a dark alley in north Los Angeles, two men exit a car and don ski masks. They make their way to the back window of a first-floor apartment and shatter the locked window with a hammer. One of the men carefully reaches through the broken glass and attempts to unlock the dead bolt from inside. At that instant, however, a pair of police officers shine their flashlights on the burglars and order them to freeze.
It might appear the police officers just happened to be at the right place at the right time. In reality, they had been patrolling the area more closely because a computer program had informed them the likelihood of a burglary was high. The software even pin-pointed the location and time frame that the crime was most likely to occur.
Such accounts might sound like the stuff of science-fiction novels and films, such as Minority Report. But, remarkably, the future is now. A growing number of researchers and law enforcement agencies are exploring—and in some cases already testing—predictive policing. Using increasingly sophisticated computer models and algorithms, they are transforming Philip K. Dick novels into reality.
“The goal is to transform policing from a reactive process to a proactive process,” says Andrew Adams.
Police departments in a number of U.S. cities, including Los Angeles, New York, Memphis, and Santa Cruz, are turning to the concept to gain a better understanding of how and where to deploy resources. They are looking to stop burglaries, armed robberies, car thefts, and snag motorists running red lights.
During the last several years, predictive policing has gained more acceptance among police departments. “The goal is to transform policing from a reactive process to a proactive process,” says Andrew Adams, professor of information ethics and deputy director of the Center of Business Information Ethics at Meiji University. “It’s being driven by marked advances in computing processing power and the ability to build visualizations that weren’t possible only a few years ago.”
Data-based Policing
The concept behind predictive policing is relatively straightforward: Feed reams of data—particularly data focused on the time, distribution, and geography of past events—into a database and ferret out patterns that would not be apparent to the human eye or brain. With the resulting data, it is possible to adjust patrols and other resources to create a stronger deterrent, but also to predict where crimes are likely to take place and be in a better position to apprehend suspects.
Predictive policing “doesn’t replace police knowledge or experience, it simply complements them and helps law enforcement agencies do their work better,” notes Jeff Brantingham, associate professor and vice chair of the Department of Anthropology at the University of California, Los Angeles (UCLA) and a leading expert on predictive policing. UCLA has worked with the Los Angeles and Santa Cruz police departments to create predictive policing models that are now in use.
Predictive policing is becoming one of the hottest trends in law enforcement. Minneapolis police have used predictive analytics to forecast where the next likely armed robbery will occur after a series of fast-food restaurant holdups. In Texas, the Arlington Police Department has created maps overlaying residential burglaries with building code violations and discovered that as physical decay increases, so do burglaries. In Memphis, police are using an IBM analytics program dubbed Blue Crush to establish focus areas that benefit from additional police resources. Memphis officials say the system has reduced serious crime by 15% over a four-year span.
The Los Angeles Police Department (LAPD) has worked with UCLA researchers for the last seven years to explore mathematical models that could help combat crime. In 2008, the department came across an academic journal article that mentioned predictive policing. “We began looking into the concept and decided to implement it within a 50-square-mile area that has approximately 250,000 people living in it,” says Sean Malinowski, a captain and patrol commanding officer for the LAPD’s Foothill Division.
Predictive policing “doesn’t replace police knowledge or experience, it simply complements them and helps law enforcement agencies do their work better,” notes Jeff Brantingham.
The software, developed by UCLA, is currently in a testing phase. The LAPD taps into about three years of crime data to generate daily probability reports of when and where crimes are more likely to occur. Every day, officers participate in a briefing that identifies high-risk areas. They view 20 small geographic boxes overlaying a map that displays the highest probably of crimes. Since implementing the program, officers spend more time patrolling these areas, but all policing decisions are left to officers’ discretion. The result? “A significant decline in crime,” Malinowski says.
Based on early results, a drop in crime exceeding 25% might not be beyond the realm of possibility. The project is under close scrutiny by UCLA researchers, who have established randomized, controlled field trials to better understand how officers apply the data. “There can be a placebo-like effect,” Brantingham cautions. “The simple fact that data exists and officers have access to it means that they are more likely to change their behavior and the way they police.”
At the Santa Cruz Police Department, which has been forced to cope with a 30% increase in service calls and a 20% decline in staffing over the last decade, predictive policing has also garnered attention. Every day, the department produces 10 hot spot maps for the city and distributes them at roll call to officers patrolling these areas that day. The maps display high-risk neighborhoods and street corners in red. George Mohler, an assistant professor of mathematics at Santa Clara University and a leading researcher in predictive policing, developed the software program.
The department enters daily data into a spreadsheet and geocodes the information before it is run through the software. During the first six months of using the predictive policing model, the Santa Cruz Police Department recorded a 15% decrease in burglary and property theft crimes year-over-year. However, the department admits it is too early to make a definitive call on the effectiveness of predictive policing. “We haven’t added more officers, we didn’t allocate additional resources, we didn’t change the beat structures,” says Zach Friend, a crime analyst for the department. What’s more, there is virtually no additional cost associated with using predictive policing techniques.
Friend says the department’s officers have been extremely receptive to the concept. A small amount of initial skepticism and resistance has been replaced with genuine enthusiasm for predictive policing. “They’re excited about it,” says Friend. “They understand that they are preventing actual burglaries and break-ins. This is ultimately about putting their knowledge and skills to work in a more effective way. The system supplements and aids their intuition.”
Of course, some observers have concerns about the concept. They question whether predictive policing could put some neighborhoods under permanent armed patrol and create biases among officers. Adams of Meiji University describes the entire topics of policing and information as a “minefield.” There’s a tendency, he says, “for political judgment to become intermingled with the data.”
Adams believes that transparency is paramount. Police agencies must develop policies and procedures for using the data and ensure that communities fully trust it is being put to use honestly, fairly, and in the public’s best interest. “There are concerns that predictive policing can create a shield of objectivity,” says Adams. “Also, if the technique is used to put individuals under surveillance, it could touch on privacy, civil liberty, and due process issues.”
A New Policing Model
The genesis of predictive policing lies in crime-tracking systems such as CompStat that compile statistics but rely heavily on humans to connect the dots. CompStat was first introduced in the mid-1990s by the New York Police Department and is now widely used in major U.S. cities. Although it is not a software system per se and it does not incorporate mathematical algorithms, many departments rely on CompStat’s interactive mapping capabilities to better understand crime patterns and forecast where crime might occur.
“The real world is messy and fuzzy,” says Andrews. “Predictive policing offers a lot of promise, but it’s important to keep it in context. Predicting behavior is not an exact science.”
The science behind predictive policing is advancing rapidly. Today, mathematicians and computer scientists are building far more sophisticated algorithms based on a variety of models. Andrea Bertozzi, a professor of mathematics at UCLA, says that “crime will always have a significant stochastic or random component. The non-random component is what we try to exploit.” But by developing models that mimic nature, such as earthquake aftershock patterns, it is possible to design mathematical algorithms to find order in seemingly chaotic events. In fact, the capabilities extend beyond predictive policing and into areas such as solving crimes.
Bertozzi, who has helped develop UCLA’s models, says the mathematical framework used for predictive policing is still in the early stages. Over time, researchers could add other variables into the model, including epidemiology data, climate and weather, economic factors, even spatial geography and census data. Yet, she is also quick to point out that it is essential to keep models “fairly simple.” By adding additional data layers, “you can wind up with a model that doesn’t say anything definitive,” says Bertozzi. “There are simply too many variables to conduct valid statistical modeling.”
There is also no guarantee that predictive policing will yield permanent results. It is possible that, over time, some criminals may react to what the machines have learned and alter their behavior and patterns to evade detection. This dynamic already occurs in many models, including online gaming. As a result, any drop in crime might prove temporary and an ongoing cat-and-mouse game could ensue.
Brantingham says predictive policing will never become a magic elixir or a real-world version of Minority Report. It is simply about crunching numbers and identifying patterns so law enforcement agencies can better meet the needs of communities. “The process shouldn’t change the way policing is done, it should make it more efficient,” he says.
Ultimately, predictive policing is likely to become a cornerstone of law enforcement. What’s more, it will change the way law enforcement agencies operate and the way they allocate people and resources. “The real world is messy and fuzzy,” says Andrews. “Predictive policing offers a lot of promise, but it’s important to keep it in context. Predicting behavior is not an exact science. Predictions should never be taken as absolute reality.”
Further Reading
Beck, C. and McCue, C.
Predictive policing: What we can learn from Wal-Mart and Amazon about fighting crime during a recession. The Police Chief 76, 11, Nov. 2009.
Malek, M.
Predictive algorithms and technologies for availability enhancement, Lecture Notes in Computing Science 5017, 2008.
Mills, E.
An ounce of prevention, Law Enforcement Technology 36, 9, Sept. 2009.
Short, M.B., D’Orsogna, M.R., Pasour, G.E., Brantingham, P.J., Bertozzi, A.L., and Chayes, L.B.
A statistical model of criminal behavior, Mathematical Models and Methods in Applied Sciences 18, Suppl., 2008.
Stomakhin, A., Short, M.B., and Bertozzi, A.L.
Reconstruction of missing data in social networks based on temporal patterns of interactions, Inverse Problems 27, 11, Nov. 2011.
Join the Discussion (0)
Become a Member or Sign In to Post a Comment